SANE 2014 - Speech and Audio in the Northeast
October 23, 2014
The workshop is now over. Slides for the talks are available through the links in the schedule below. Photos of the day are available through the SANE News group.
SANE 2014, a one-day event gathering researchers and students in speech and audio from the Northeast of the American continent, will be held on Thursday October 23, 2014 at MIT, in Cambridge, MA.
It is a follow-up to SANE 2012, held at Mitsubishi Electric Research Labs (MERL), and SANE 2013, held at Columbia University, which each gathered around 70 researchers and students.
SANE 2014 will feature invited talks by leading researchers from the Northeast as well as Europe. It will also feature a lively poster session during lunch time, open to both students and researchers.
Details
- Date: Thursday, October 23, 2014
- Venue: MIT, Cambridge, MA (Stata Center, 4th floor, Kiva room)
Schedule
8:30-9:55 | Registration and Breakfast |
8:55-9:00 | Welcome |
9:00-9:45 | Najim Dehak (MIT) "I-vector representation based on GMM and DNN for audio classification" [Slides] |
9:45-10:30 | Hakan Erdogan (MERL - Sabanci University) "Using deep neural networks for single channel source separation" [Slides] |
10:30-11:00 | Coffee Break |
11:00-11:45 | George Saon (IBM Research) "Speaker adaptation and sequence discriminative training for DNN acoustic models" [Slides] |
11:45-12:30 | David Wingate (Lyric Labs) "Improving Automatic Speech Recognition through Non-negative Tensor Factorization" [Slides] |
12:30-2:45 | Lunch / Poster Session |
2:45-3:30 | Gaël Richard (Telecom ParisTech) "Informed Audio Source Separation" |
3:30-4:15 | Andrew Senior (Google Research, NYC) "LVCSR with Long-Short-Term Memory Recurrent Neural Networks" [Slides] |
4:15-5:00 | Stavros Tsakalidis (BBN - Raytheon) "Keyword spotting for low resource languages" [Slides] |
5:00-5:15 | Closing remarks |
5:30-... | Drinks at Cambridge Brewing Company (map) |
All talks: Kiva Seminar Room (32-G449), 4th floor, Stata Center (Gates Tower)
Lunch/Poster: Hewlett Room (32-G882), 8th floor, Stata Center (Gates Tower)
Poster Session
- "Turbo Automatic Speech Recognition Operating on Magnitude and Phase Features"
Simon Receveur (TU Braunschweig) - "Dynamic Stream Weight Estimation in Coupled-HMM-based Audio-visual Speech Recognition Using Multilayer Perceptrons"
Ahmed Hussen Abdelaziz, Dorothea Kolossa (Ruhr-Universität Bochum) - "The effects of whispered speech on state-of-the-art voice based biometrics systems"
Milton Orlando Sarria Paja (University of Quebec) - "Strong similarities between deep neural networks trained on speech tasks and human auditory cortex"
Alexander J E Kell*, Daniel Yamins*, Sam Norman-Haignere, Josh H McDermott (MIT — *AK & DY contributed equally to this work) - "Child Automatic Speech Recognition for US English: Child Interaction with Living-Room-Electronic-Devices"
Sharmistha S. Gray, Daniel Willett, Jianhua Lu, Joel Pinto, Paul Maergner, Nathan Bodenstab (Nuance) - "Characterizing engaged and distressed interactions: a short case study on the 'Comcast belligerent' call"
John Kane, Cristina Gorrostieta, Ali Azarbayejani (Cogito) - "Speech Recognition Robustness Studies at Ford Motor Company"
Francois Charette, John Huber, Brigitte Richardson (Ford Motor Company) - "A Big Data Approach to Acoustic Model Training Corpus Selection"
Olga Kapralova, John Alex, Eugene Weinstein, Pedro Moreno, Olivier Siohan (Google) - "Speech Acoustic Modeling from Raw Multichannel Waveforms"
Yedid Hoshen, Ron Weiss, and Kevin Wilson (Google) - "Speech Representations based on a Theory for Learning Invariances"
Stephen Voinea, Chiyuan Zhang, Georgios Evangelopoulos, Lorenzo Rosasco, Tomaso Poggio (MIT) - "Evaluation of Speech Enhancement Methods on ASR Systems using the 2nd CHiME Challenge Track 2"
Yi Luan, Shinji Watanabe, Jonathan Le Roux, John R. Hershey, Emmanuel Vincent (MERL, INRIA) - "Responses to Natural Sounds Reveal the Functional Organization of Human Auditory Cortex"
Sam Norman-Haignere, Josh McDermott, Nancy Kanwisher (MIT)
Registration
Registration is free but required. We are now at capacity and accept registrations on the waiting list. We typically have a few slots opening up in the days leading to the workshop, so we encourage those interested in attending this event to contact us as soon as possible by sending an email to with your name and affiliation.
Directions
The workshop will be hosted in the Stata Center of MIT, in Cambridge, MA. The Stata Center is a short (5 min) walk from the Kendall/MIT station on the T Red Line.
Lecture sessions, as well as the breakfast and coffee break, will take place on the 4th floor, in the Kiva room (32-G449). The lunch and poster session will take place on the 8th floor, in the Hewlett room (32-G882).
Organizing Committee
- Jonathan Le Roux (MERL)
- Jim Glass (MIT)
- John R. Hershey (MERL)
Sponsors
Talks
I-vector representation based on GMM and DNN for audio classification
The I-vector approach became the state of the art approach in several audio classification tasks such as speaker and language recognition. This approach consists of modeling and capturing all the different variability in the Gaussian Mixture Model (GMM) mean components between several audio recordings. More recently this approach had been successfully extended on modeling the variability between the GMM weights rather than the GMM means. This last technique named Non-Negative Factor Analysis (NFA) needed to deal with the fact that the GMM weights are always positive and they should sum to one. In this talk, we will show how the NFA approach or similar subspaces approaches can be also used to model the neuron activations on the deep neural network model for language and dialect recognition task.
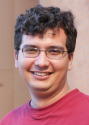
Najim Dehak received his Engineering degree in Artificial Intelligence in 2003 from Universite des Sciences et de la Technologie d'Oran, Algeria, and his MS degree in Pattern Recognition and Artificial Intelligence Applications in 2004 from the Universite de Pierre et Marie Curie, Paris, France. He obtained his Ph.D. degree from Ecole de Technologie Superieure (ETS), Montreal in 2009. During his Ph.D. studies he was also with Centre de recherche informatique de Montreal (CRIM), Canada. In the summer of 2008, he participated in the Johns Hopkins University, Center for Language and Speech Processing, Summer Workshop. During that time, he proposed a new system for speaker verification that uses factor analysis to extract speaker-specific features, thus paving the way for the development of the i-vector framework. Dr. Dehak is currently a research scientist in the Spoken Language Systems (SLS) Group at the MIT Computer Science and Artificial Intelligence Laboratory (CSAIL). His research interests are in machine learning approaches applied to speech processing and speaker modeling. The current focus of his research involves extending the concept of an i-vector representation into other audio classification problems, such as speaker diarization, language- and emotion-recognition.
Using deep neural networks for single channel source separation
Deep neural networks have seen a surging interest in the speech recognition and visual object recognition communities due to their superior performance in complex recognition tasks. Recently, researchers started using neural networks for single channel audio source separation and speech enhancement problems as well. Various alternative formulations have been proposed and improvements over non-negative matrix factorization based baseline results have been shown. In this talk, these recently proposed approaches will be covered and their similarities and differences will be indicated. Possible future directions will be discussed as well.
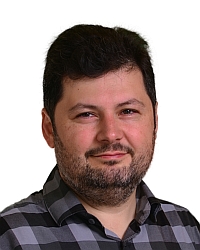
Hakan Erdogan is currently working as a visiting researcher at Mitsubishi Electric Research Laboratories on sabbatical from Sabanci University, Turkey, where he is a faculty member. He has been with Sabanci University since 2002. After obtaining his PhD from University of Michigan in 1999 which was on medical image reconstruction, Hakan worked at IBM TJ Watson Research on speech recognition and language technologies until 2002. His current research interests include speech separation and recognition, biometrics, sparse signal recovery and object recognition.
Informed Audio Source Separation
Audio source separation remains today challenging in many cases, especially in the undetermined case, when there are less observations than sources. In order to improve audio source separation performance, many recent works have turned towards so-called informed audio source separation approaches, where the separation algorithm relies on any kind of additional information about the sources to better extracting them.
The goal of this talk is to present a review of three major trends in informed audio source separation, namely:
* Auxiliary data-informed source separation, where the additional information can be for example a musical score corresponding to the musical source to be separated.
* User-guided source separation where the additional information is created by a user with the intention to improve the source separation, potentially in an iterative fashion. For example, this can be some indication about source activity in the time-frequency domain.
* Coding-based informed source separation where the additional information is created by an algorithm at a so-called encoding stage where both the sources and the mixtures are assumed known. This trend is at the crossroads of source separation and compression, and shares many similarities with the recently introduced Spatial Audio Object Coding (SAOC) scheme.
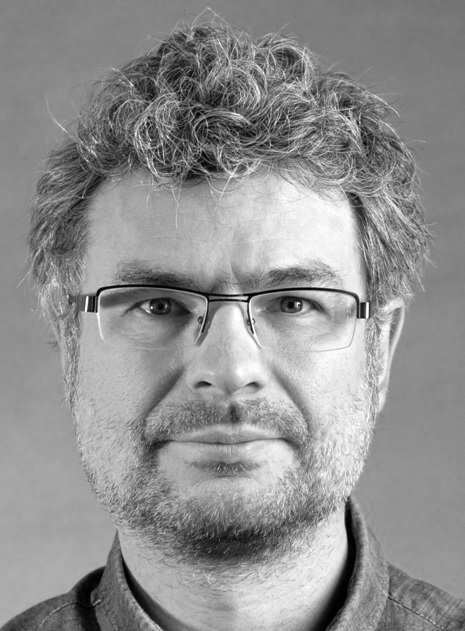
Gaël Richard received the State Engineering degree from Telecom ParisTech, France (formerly ENST) in 1990, the Ph.D. degree from LIMSI-CNRS, University of Paris-XI, in 1994 in speech synthesis, and the Habilitation à Diriger des Recherches degree from the University of Paris XI in September 2001. After the Ph.D. degree , he spent two years at the CAIP Center, Rutgers University, Piscataway, NJ, in the Speech Processing Group of Prof. J. Flanagan, where he explored innovative approaches for speech production. From 1997 to 2001, he successively worked for Matra, Bois d’Arcy, France, and for Philips, Montrouge, France. In particular, he was the Project Manager of several large scale European projects in the field of audio and multimodal signal processing. In September 2001, he joined the Department of Signal and Image Processing, Telecom ParisTech, where he is now a Full Professor in audio signal processing and Head of the Audio, Acoustics, and Waves research group. He is a coauthor of over 150 papers and inventor in a number of patents and is also one of the experts of the European commission in the field of speech and audio signal processing. He was an Associate Editor of the IEEE Transactions on Audio, Speech and Language Processing between 1997 and 2011 and one of the guest editors of the special issue on “Music Signal Processing” of IEEE Journal on Selected Topics in Signal Processing (2011). He currently is a member of the IEEE Audio and Acoustic Signal Processing Technical Committee, member of the EURASIP and AES and senior member of the IEEE.
Speaker adaptation and sequence discriminative training for DNN acoustic models
In the first part of the talk, we propose to adapt deep neural network (DNN) acoustic models to a target speaker by supplying speaker identity vectors (i-vectors) as input features to the network in parallel with the regular acoustic features for ASR. Experimental results on a Switchboard 300 hours corpus show that DNNs trained on speaker independent features and i-vectors achieve a 10% relative improvement in word error rate over networks trained on speaker independent features only. These networks are comparable in performance to DNNs trained on speaker-adapted features (with VTLN and FMLLR) with the advantage that only one decoding pass is needed. Furthermore, networks trained on speaker-adapted features and i-vectors achieve a 5-6% relative improvement in WER after hessian-free sequence discriminative training over networks trained on speaker-adapted features only.
In the second part of the talk, we compare two optimization methods for lattice-based sequence discriminative training of neural network acoustic models: distributed Hessian-free (DHF) and stochastic gradient descent (SGD). Our findings on two different LVCSR tasks suggest that SGD running on a single GPU machine achieves the best accuracy 2.5 times faster than DHF running on multiple non-GPU machines; however, DHF training achieves a higher accuracy at the end of the optimization. In addition, we present an improved modified forward-backward algorithm for computing lattice-based expected loss functions and gradients that results in a 34% speedup for SGD.
In the third part of the talk, we describe a hybrid GPU/CPU architecture for SGD sequence discriminative training of neural network acoustic models under a lattice-based minimum Bayes risk (MBR) criterion. The crux of the method is to run SGD on a GPU card which consumes frame-randomized mini-batches produced by multiple workers running on a cluster of multi-core CPU nodes which compute HMM state MBR occupancies. Using this architecture, it is possible to match the speed of GPU-based SGD cross-entropy training (1 hour of processing per 100 hours of audio on Switchboard). Additionally, we compare different ways of doing frame randomization and discuss experimental results on three LVCSR tasks (Switchboard 300 hours, English broadcast news 50 hours, and noisy Levantine telephone conversations 300 hours).
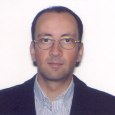
George Saon received his M.Sc. and PhD degrees in Computer Science from Henri Poincare University in Nancy, France in 1994 and 1997. In 1995, Dr. Saon obtained his engineer diploma from the Polytechnic University of Bucharest, Romania. From 1994 to 1998, he worked on two-dimensional stochastic models for off-line handwriting recognition at the Laboratoire Lorrain de Recherche en Informatique et ses Applications (LORIA). Since 1998, Dr. Saon is with the IBM T.J. Watson Research Center where he worked on a variety of problems spanning several areas of large vocabulary continuous speech recognition such as discriminative feature processing, acoustic modeling, speaker adaptation and large vocabulary decoding algorithms. Since 2001, Dr. Saon has been a key member of IBM's speech recognition team which participated in several U.S. government-sponsored evaluations for the EARS, SPINE, GALE and RATS programs. He has published over 100 conference and journal papers and holds several patents in the field of ASR. He is the recipient of two best paper awards (INTERSPEECH 2010, ASRU 2011) and serves currently as an elected member of the IEEE Speech and Language Technical Committee.
LVCSR with Long-Short-Term Memory Recurrent Neural Networks
Our recent work has shown that deep Long Short-Term Memory Recurrent Neural Networks (LSTM-RNNs) give improved accuracy over deep neural networks for large vocabulary continuous speech recognition. I will describe our task of recognizing speech from Google Now in dozens of languages and give an overview of LSTM-RNNs for acoustic modelling. I will describe distributed Asynchronous Stochastic Gradient Descent training of LSTMs on clusters of hundreds of machines, and show improved results through sequence-discriminative training.
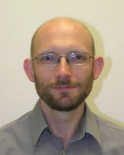
Andrew Senior received his PhD from Cambridge University for his thesis “Recurrent Neural Networks for Offline Cursive Handwriting Recognition”. He is a research scientist and technical lead at Google, New York, where he works in the speech team on deep and recurrent neural networks for acoustic modelling. Before joining Google he worked at IBM Research in the areas of handwriting, audio-visual speech, face and fingerprint recognition as well as video privacy protection and visual tracking.
Keyword spotting for low resource languages
Dr. Stavros Tsakalidis
One application of speech recognition technology is keyword search. Accurate speech recognition becomes difficult when the speech is conversational or when the amount of training for a new language is very limited. The word error rate can increase to high levels, like 60% or 70%, which makes it very difficult to read the output. But it is still possible to perform keyword search (KWS), which makes it feasible for someone to find particular passages quickly. There are many additional techniques that are critical to high quality KWS. While good speech recognition technology is necessary for KWS, it is not sufficient. This talk will describe some of the advanced techniques that are used for keyword search. Some of these topics covered will include: the basic speech recognition system and features for good speech recognition, KWS search techniques with high recall, dealing with limited training and high out-of-vocabulary rates, score normalization and system combination.
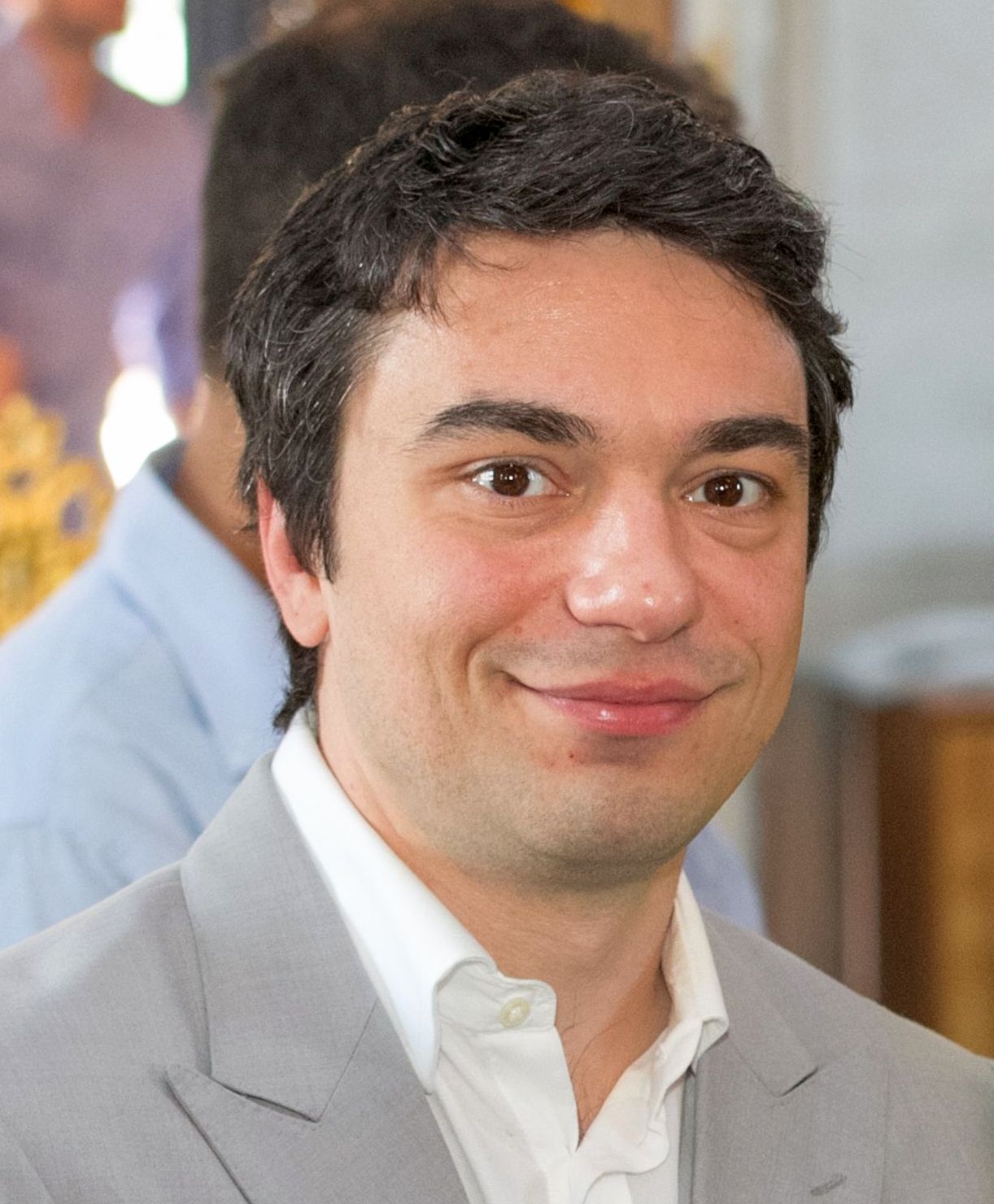
Dr. Tsakalidis, a senior Scientist at BBN Technologies, received the B.A. Degree from the Technical University of Crete (TUC), in 1998, the M.S. and Ph.D. degrees from the Johns Hopkins University, in 2005, all in electrical engineering. His expertise includes areas such as discriminative training, speaker adaptation, acoustic modeling with low resources, and keyword spotting.
He is currently the co-Principal Investigator on the IARPA Babel Program, which focuses on developing Keyword Spotting technology that can be rapidly applied to any human language. In 2011, he led the research in the spoken content analysis component for event detection in videos for the IARPA ALADDIN program. From 2007 to 2010, he was the key contributor in the development of BBN’s speech-to-speech translation system for the DARPA TRANSTAC program. At JHU, he developed discriminative training procedures that employed linear transforms for feature normalization. At TUC, he designed a novel acoustic model for SRI's DECIPHER system combining subvector quantization and mixtures of discrete distributions.
Improving Automatic Speech Recognition through Non-negative Tensor Factorization
Automatic speech recognition (ASR) in noisy and far-field environments is a challenging problem. In this talk, I will discuss Lyric Lab's recent work on improving ASR through audio source separation. Our methods combine probabilistic non-negative tensor factorization and direction-of-arrival information derived from multiple microphones in a single algorithm that simultaneously estimates models of speech, noise, and their spatial positions. I will also present experimental results in living room and automotive settings demonstrating that the separated speech signals can (sometimes dramatically) improve ASR word error rates. Time permitting, I will also discuss Lyric's work on ultra-miniature MEMS microphone arrays, with mic spacings of 1mm, and their application to source separation and ASR in the context of smartphones, tablets and wearables.
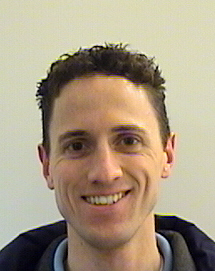
David Wingate is currently the Director of Lyric Labs, an advanced R&D group in Analog Devices, Inc. that emphasizes applied research in machine learning, novel hardware, and probabilistic inference. Before joining Lyric as a research scientist in 2012, he was a research scientist at MIT with a joint appointment in the Laboratory for Information Decision Systems and the Computational Cognitive Science group. David received a B.S. and M.S. in Computer Science from Brigham Young University in 2002 and 2004, and a Ph.D. in Computer Science from University of Michigan in 2008.
David's research interests lie at the intersection of probabilistic programming, hardware accelerated probabilistic inference and machine learning. His research spans diverse topics in audio processing, Bayesian nonparametrics, reinforcement learning, massively parallel processing, visual perception, dynamical systems modeling and robotics.