SANE 2023 - Speech and Audio in the Northeast
October 26, 2023
SANE 2023, a one-day event gathering researchers and students in speech and audio from the Northeast of the American continent, was held on Thursday October 26, 2023 at NYU in Brooklyn, New York.
It was the 10th edition in the SANE series of workshops, which started in 2012 and is typically held every year alternately in Boston and New York. Since the first edition, the audience has steadily grown, and SANE 2023 broke SANE 2019's record with 200 participants and 51 posters.
This year's SANE took place in conjunction with the WASPAA workshop, held October 22-25 in upstate New York.
SANE 2023 featured invited talks by leading researchers from the Northeast as well as from the wider community. It also featured a lively poster session, open to both students and researchers.
Details
- Date: Thursday, October 26, 2023
- Venue: Pfizer Auditorium, New York University, 5 MetroTech Center - Dibner Hall, Brooklyn, New York.
Schedule
Click on the talk title to jump to the abstract and bio.8:00-8:55 | Breakfast |
8:55-9:00 | Welcome |
9:00-9:45 | Arsha Nagrani (Google) Audio-Visual Learning for Video Understanding |
9:45-10:15 | Coffee break |
10:15-11:00 | Gaël Richard (Télécom Paris) Deep Hybrid Learning and Its Application to Unsupervised Singing Voice Separation |
11:00-11:45 | Gordon Wichern (MERL) Defining "Source" in Audio Source Separation |
11:45-12:00 | Transfer to Lunch/Poster Area |
12:00-14:00 | Lunch and Poster Session at 370 Jay St |
14:00-14:15 | Transfer to Auditorium |
14:15-15:00 | Kyunghyun Cho (NYU / Prescient Design) Beyond Test Accuracies for Studying Deep Neural Networks |
15:00-15:45 | Anna Huang (Google DeepMind / MILA) AI for Musical Creativity |
15:45-16:15 | Coffee break |
16:15-17:00 | Wenwu Wang (University of Surrey) Audio-Text Learning for Automated Audio Captioning and Generation |
17:00-17:45 | Yuan Gong (MIT) Audio Large Language Models: From Sound Perception to Understanding |
17:45-17:50 | Closing remarks |
17:50-......... | Drinks somewhere nearby |
Directions
The workshop was hosted in New York University's Pfizer Auditorium, 5 MetroTech Center - Dibner Hall, in Brooklyn, NY. The Lunch and Poster Session took place at 370 Jay St, Room 233.
Organizing Committee
- Jonathan Le Roux (MERL)
- Juan P. Bello (NYU)
- John R. Hershey (Google)
Sponsors
SANE remains a free workshop thanks to the generous contributions of the sponsors below.
Talks
Beyond Test Accuracies for Studying Deep Neural Networks
NYU / Prescient Design
Already in 2015, Leon Bottou discussed the prevalence and end of the training/test experimental paradigm in machine learning. The machine learning community has however continued to stick to this paradigm until now (2023), relying almost entirely and exclusively on the test-set accuracy, which is a rough proxy to the true quality of a machine learning system we want to measure. There are however many aspects in building a machine learning system that require more attention. Specifically, I will discuss three such aspects in this talk; (1) model assumption and construction, (2) optimization and (3) inference. For model assumption and construction, I will discuss our recent work on generative multitask learning and incidental correlation in multimodal learning. For optimization, I will talk about how we can systematically study and investigate learning trajectories. Finally for inference, I will lay out two consistencies that must be satisfied by a large-scale language model and demonstrate that most of the language models do not fully satisfy such consistencies.
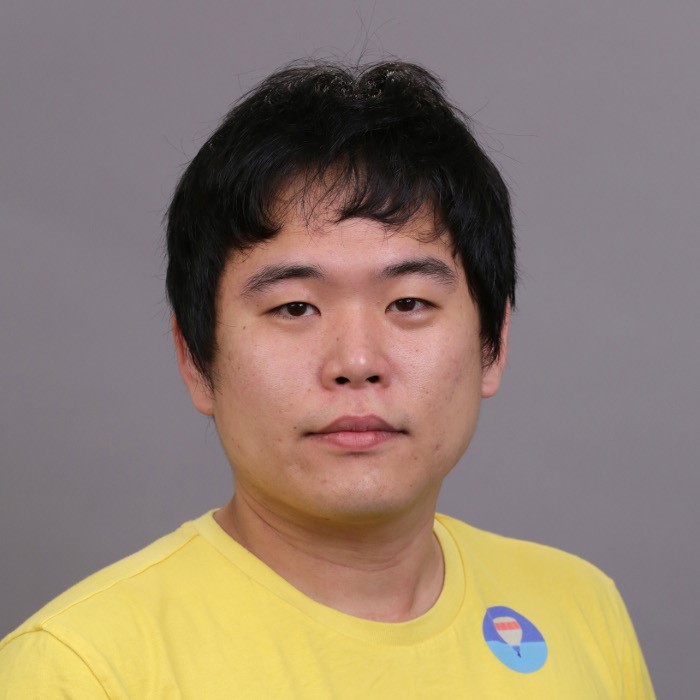
Kyunghyun Cho is an associate professor of computer science and data science at New York University and a senior director of frontier research at the Prescient Design team within Genentech Research & Early Development (gRED). He is also a CIFAR Fellow of Learning in Machines & Brains and an Associate Member of the National Academy of Engineering of Korea. He was a research scientist at Facebook AI Research from June 2017 to May 2020 and a postdoctoral fellow at University of Montreal until Summer 2015 under the supervision of Prof. Yoshua Bengio, after receiving MSc and PhD degrees from Aalto University April 2011 and April 2014, respectively, under the supervision of Prof. Juha Karhunen, Dr. Tapani Raiko and Dr. Alexander Ilin. He received the Samsung Ho-Am Prize in Engineering in 2021. He tries his best to find a balance among machine learning, natural language processing, and life, but almost always fails to do so.
Audio Large Language Models: From Sound Perception to Understanding
MIT
Our cognitive abilities enable us not only to perceive and identify sounds but also to comprehend their implicit meaning. While significant advancements have been achieved in general audio event recognition in recent years, models trained with discrete sound label sets possess limited reasoning and understanding capabilities, e.g., the model may recognize the clock chime 6 times, but not know that it indicates a time of 6 o'clock. Can we build an AI model that has both audio perception and reasoning ability?
In this talk, I will share our recent progress in audio large language model (LLM) development. Specifically, I will first introduce a novel GPT-assisted method to generate our large-scale open-ended audio question-answering dataset OpenAQA. I will then discuss the key design choices and the model architecture of our audio large language model. Finally, I will also discuss how to connect an automatic speech recognition model with an audio large language model for joint audio and speech understanding.
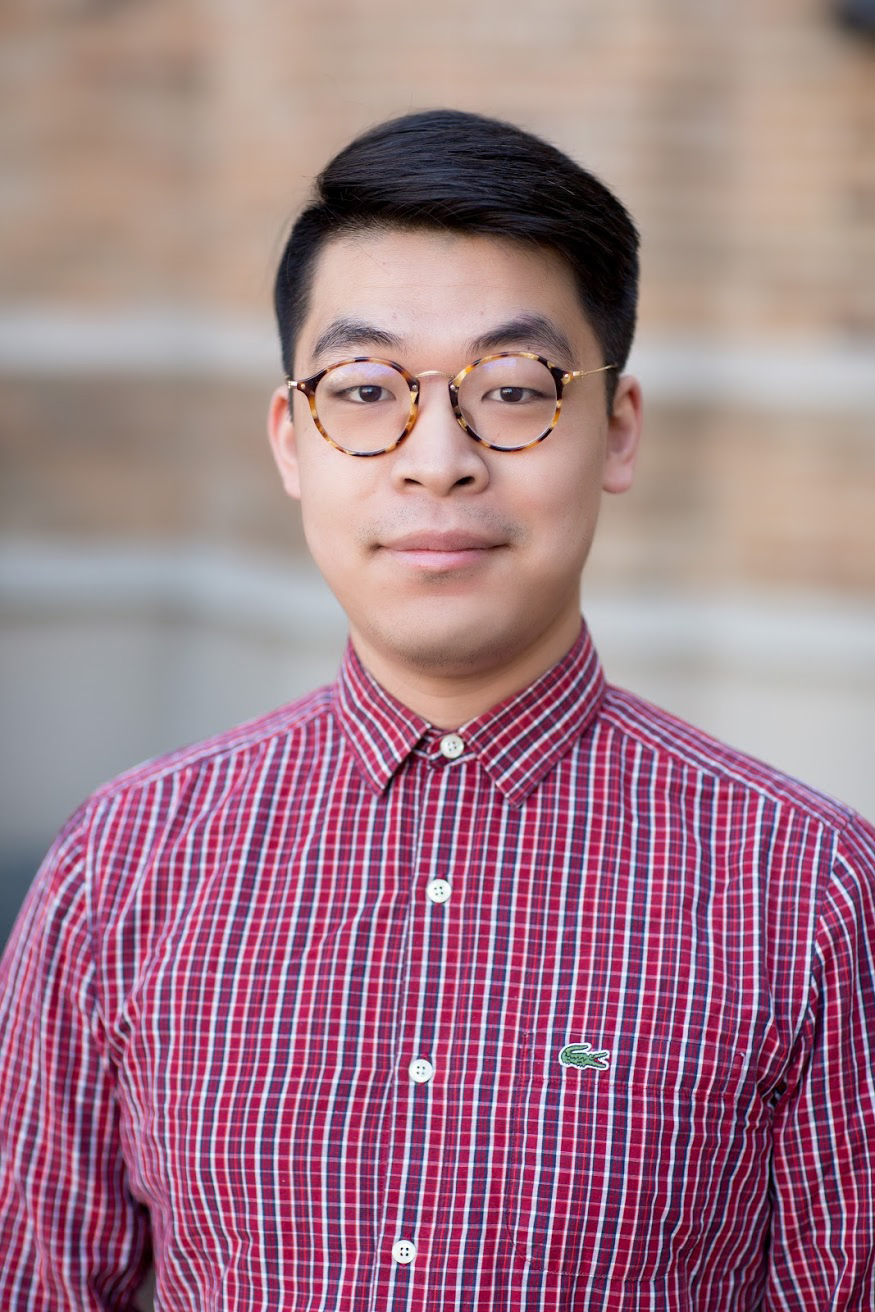
Yuan Gong is a research scientist at the MIT Computer Science and Artificial Intelligence Laboratory (CSAIL) working on audio and speech signal analysis. Recently he has been focusing on connecting audio perception with understanding with audio-conditioned large language models. He received his Ph.D. degree in computer science from the University of Notre Dame, IN, USA, and his B.S. degree in biomedical engineering from Fudan University, Shanghai, China in 2020 and 2015, respectively. He has published over 25 peer-reviewed papers in Interspeech, ICASSP, ICLR, ICCV, AAAI, etc. He won the 2017 AVEC depression detection challenge and one of his papers was nominated for the Best Student Paper Award in Interspeech 2019.
AI for Musical Creativity
Google DeepMind / MILA
Advances in generative modeling have opened up exciting new possibilities for music making. How can we leverage these models to support human creative processes? First, I’ll illustrate how we can design generative models to better support music composition and performance synthesis: Coconet, the ML model behind the Bach Doodle, supports a nonlinear compositional process through an iterative block-Gibbs like generative procedure, while MIDI-DDSP supports intuitive user control in performance synthesis through hierarchical modeling. Second, I’ll propose a common framework, Expressive Communication, for evaluating how developments in generative models and steering interfaces are both important for empowering human-ai co-creation, where the goal is to create music that communicates an imagery or mood. Third, I’ll introduce the AI Song Contest and discuss some of the technical, creative, and sociocultural challenges musicians face when adapting ML-powered tools into their creative workflows. Looking ahead, I’m excited to co-design with musicians to discover new modes of human-ai collaboration. I’m interested in designing visualizations and interactions that can help musicians understand and steer system behavior, and algorithms that can learn from their feedback in more organic ways. I aim to build systems that musicians can shape, negotiate with, and jam with in their creative practice.
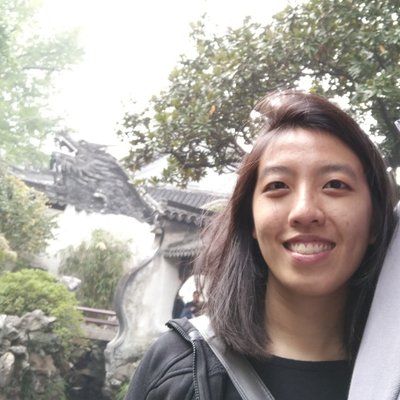
Anna Huang co-leads the Magenta project at Google DeepMind. She is also a Canada CIFAR AI Chair at Mila—Québec AI Institute, and an Adjunct Professor at Université de Montréal. Her research is at the intersection of machine learning and human-computer interaction, with the goal of supporting music making, and more generally the human creative process. She is the creator of Music Transformer and Coconet. Coconet was the ML model that powered Google’s first AI Doodle, the Bach Doodle, in two days enabling tens of millions of users around the world to co-compose with ML in their web browser. She was an organizer for the international AI Song Contest, and a guest editor for TISMIR's special issue on AI and Musical Creativity.
Audio-Visual Learning for Video Understanding
Humans are effortlessly able to learn from a constant video stream of multimodal data (audio and visual). This is in contrast to machine learning models for audio and vision, which have traditionally been trained separately. We begin by reviewing biological models for audio-visual learning, and then dive into some recent fusion architectures, exploring how auditory and visual signals can be combined for various video tasks such as video classification, video retrieval and automatic video captioning. We will cover recent papers at Neurips, CVPR and ECCV.
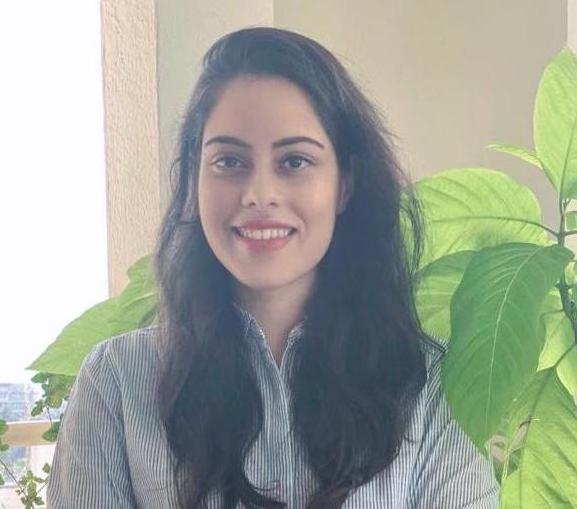
Arsha Nagrani is a Senior Research Scientist at Google Research. She obtained her PhD from the VGG group in the University of Oxford, where her thesis won the ELLIS PhD Award. Her research focuses on cross-modal and multi-modal machine learning techniques for video recognition. Her work has been recognised by a Best Student Paper Award, Outstanding Paper Award, a Google PhD Fellowship and a Townsend Scholarship, and has been covered by major outlets such as The New Scientist, MIT Tech review and Verdict.
Deep Hybrid Learning and Its Application to Unsupervised Singing Voice Separation
Télécom Paris
Access to ever-larger supercomputing facilities combined with the availability of massive data sets (although largely unannotated) has led to a clear trend towards purely data-driven approaches for many applications in speech, music, and audio processing. The field has even moved towards end-to-end neural approaches aimed at directly solving machine learning problems for raw acoustic signals (e.g., waveform-based signals captured directly from microphones) while only crudely considering the nature and structure of the data being processed. We believe that it is important to rather build hybrid deep learning methods by integrating our prior knowledge about the data. In the speech or music domain, prior knowledge can relate to the production of sound (using an acoustic or physical model), the way sound is perceived (based on a perceptual model), or for music for instance how it is composed (using a musicological model). In this presentation, we will first illustrate the concept and potential of such model-based deep learning approaches (or hybrid deep learning) and describe in more details its application to unsupervised singing voice separation from choir recordings.
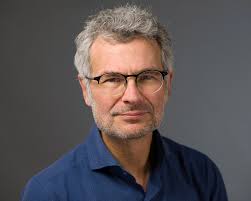
Gaël Richard received the State Engineering degree from Telecom Paris, France in 1990, the Ph.D. degree and Habilitation from University of ParisSaclay respectively in 1994 and 2001. After the Ph.D. degree, he spent two years at Rutgers University, Piscataway, NJ, in the Speech Processing Group of Prof. J. Flanagan, where he explored innovative approaches for speech production. From 1997 to 2001, he successively worked for Matra, Bois d’Arcy, France, and for Philips, Montrouge, France. He then joined Telecom Paris, where he is now a Full Professor in audio signal processing. He is also the scientific co- director of the Hi! PARIS interdisciplinary center on Artificial Intelligence and Data analytics. He is a coauthor of over 250 papers and inventor in 10 patents. His research interests are mainly in the field of speech and audio signal processing and include topics such as signal representations, source separation, machine learning methods for audio/music signals and music information retrieval. He received, in 2020, the Grand prize of IMT-National academy of science for his research contribution in sciences and technologies. He is a fellow member of the IEEE and the past Chair of the IEEE SPS Technical Committee for Audio and Acoustic Signal Processing. In 2022, he is awarded of an advanced ERC grant of the European Union for the project “HI-Audio: Hybrid and Interpretable deep neural audio machines”.
Audio-Text Learning for Automated Audio Captioning and Generation
University of Surrey
Cross modal generation of audio and text has emerged as an important research area in audio signal processing and natural language processing. Audio-to-text generation, also known as automated audio captioning, aims to provide a meaningful language description of the audio content for an audio clip. This can be used for assisting the hearing-impaired to understand environmental sounds, facilitating retrieval of multimedia content, and analyzing sounds for security surveillance. Text-to-audio generation aims to produce an audio clip based on a text prompt which is a language description of the audio content to be generated. This can be used as sound synthesis tools for film making, game design, virtual reality/metaverse, digital media, and digital assistants for text understanding by the visually impaired. To achieve cross modal audio-text generation, it is essential to comprehend the audio events and scenes within an audio clip, as well as interpret the textual information presented in natural language. Additionally, learning the mapping and alignment of these two streams of information is crucial. Exciting developments have recently emerged in the field of automated audio-text cross modal generation. In this talk, we will give an introduction of this field, including problem description, potential applications, datasets, open challenges, recent technical progresses, and possible future research directions.
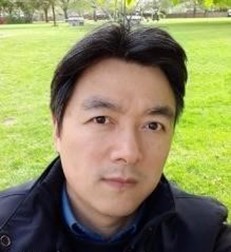
Wenwu Wang is a Professor in Signal Processing and Machine Learning, and a Co-Director of the Machine Audition Lab within the Centre for Vision Speech and Signal Processing, University of Surrey, UK. He is also an AI Fellow at the Surrey Institute for People Centred Artificial Intelligence. His current research interests include signal processing, machine learning and perception, artificial intelligence, machine audition (listening), and statistical anomaly detection. He has (co)-authored over 300 papers in these areas. He has been involved as Principal or Co-Investigator in more than 30 research projects, funded by UK and EU research councils, and industry (e.g. BBC, NPL, Samsung, Tencent, Huawei, Saab, Atlas, and Kaon). He is a (co-)author or (co-)recipient of over 15 awards including the 2022 IEEE Signal Processing Society Young Author Best Paper Award, ICAUS 2021 Best Paper Award, DCASE 2020 Judge’s Award, DCASE 2019 and 2020 Reproducible System Award, LVA/ICA 2018 Best Student Paper Award, FSDM 2016 Best Oral Presentation, and Dstl Challenge 2012 Best Solution Award. He is an Associate Editor (2020-2025) for IEEE/ACM Transactions on Audio Speech and Language Processing, an Associate Editor (2022-) for (Nature) Scientific Report, and a Specialty Editor (2021-) in Chief of Frontier in Signal Processing. He was a Senior Area Editor (2019-2023) and Associate Editor (2014-2028) for IEEE Transactions on Signal Processing. He is a Board Member (2023-2024) of IEEE Signal Processing Society (SPS) Technical Directions Board, the elected Chair (2023-2024) of IEEE SPS Machine Learning for Signal Processing Technical Committee, the Vice Chair (2022-2024) of the EURASIP Technical Area Committee on Acoustic Speech and Music Signal Processing, an elected Member (2022-2024) of the IEEE SPS Signal Processing Theory and Methods Technical Committee, and an elected Member (2019-) of the International Steering Committee of Latent Variable Analysis and Signal Separation. He was a Satellite Workshop Co-Chair for INTERSPEECH 2022, a Publication Co-Chair for IEEE ICASSP 2019, Local Arrangement Co-Chair of IEEE MLSP 2013, and Publicity Co-Chair of IEEE SSP 2009. He is a Satellite Workshop Co-Chair for IEEE ICASSP 2024.
Defining "Source" in Audio Source Separation
MERL
The cocktail party problem aims at isolating any source of interest within a complex acoustic scene, and has long inspired audio source separation research. In the classical setup, it is generally clear that the source of interest is one speaker among the several simultaneously talking at the party. However, with the explosion of purely data-driven techniques, it is now possible to separate nearly any type of sound from a wide range of signals including non-professional ambient recordings, music, movie soundtracks, and industrial machines. This increase in flexibility has created a new challenge: defining how a user specifies the source of interest. To better embrace this ambiguity, I will first describe how we use hierarchical targets for training source separation networks, where the model learns to separate at multiple levels of granularity, e.g., separate all music from a movie soundtrack in addition to isolating the individual instruments. These hierarchical relationships can be further enforced using hyperbolic representations inside the audio source separation network, enabling novel user interfaces and aiding model explainability. Finally, I will discuss how we incorporate the different meanings for “source” into source separation model prompts using qualitative audio features, natural language, or example audio clips.
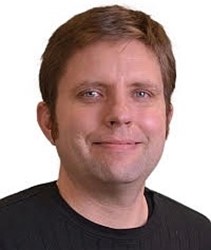
Gordon Wichern is a Senior Principal Research Scientist at Mitsubishi Electric Research Laboratories (MERL) in Cambridge, Massachusetts. He received his B.Sc. and M.Sc. degrees from Colorado State University and his Ph.D. from Arizona State University. Prior to joining MERL, he was a member of the research team at iZotope, where he focused on applying novel signal processing and machine learning techniques to music and post-production software, and before that a member of the Technical Staff at MIT Lincoln Laboratory. He is the Chair of the AES Technical Committee on Machine Learning and Artificial Intelligence (TC-MLAI), and a member of the IEEE Audio and Acoustic Signal Processing Technical Committee (AASP-TC). His research interests span the audio signal processing and machine learning fields, with a recent focus on source separation and sound event detection.
Posters
Instructions for posters: the poster boards are 30"x40", and can be placed in portrait or landscape orientation.
- 1. The Changing Sound of Music: An Exploratory Corpus Study of Vocal Trends Over Time
Elena Georgieva, Pablo Ripollés, Brian McFee (NYU)Abstract- Recent advancements in audio processing provide a new opportunity to study musical trends using quantitative methods. In this work, we conduct an exploratory study of 43,153 vocal tracks of popular songs spanning nearly a century, from 1924 to 2010. We use source separation to extract the vocal stem and fundamental frequency (f0) estimation to analyze pitch tracks. Additionally, we extract pitch characteristics including mean pitch, total variation, and pitch class entropy of each song. We conduct statistical analysis of vocal pitch across years and genres, and report significant trends in our metrics over time, as well as significant differences in trends between genres. Our study demonstrates the utility of this method for studying vocals, contributes to the understanding of vocal trends, and showcases the potential of quantitative approaches in musicology.
- 2. ROOM SCAPER: A Library to Simulate and Augment Soundscapes for Sound Event Localization and Detection in Realistic Rooms
Iran R. Roman, Christopher Ick, Siwen Ding, Adrian S. Roman, Brian McFee, Juan Bello (NYU)Abstract- Sound event localization and detection (SELD) is an important task in machine listening. Major advancements rely on simulated data with sound events in specific rooms and strongspatio-temporal labels. SELD data is simulated by convolving spatially-localized room impulse responses (RIRs) with sound waveforms to place sound events in a soundscape. However, RIRs require manual collection in specific rooms. We present room scaper, a library for SELD data simulation and augmentation. Compared to existing tools, room scaper emulates virtual rooms via parameters such as size and wall absorption. This allows for parameterized placement (includ- ing movement) of foreground and background sound sources. room scaper also includes data augmentation pipelines that can be applied to existing SELD data. As a case study, we use room scaper to add rooms and acoustic conditions to the DCASE SELD challenge data. Training a model with our data led to progressive performance improves as a di- rect function of acoustic diversity. These results show that room scaper is valuable to train robust SELD models.
- 3. Towards High Resolution Weather Monitoring with Sound Data
Enis Berk Çoban, Megan Perra, Michael I Mandel (CUNY, SUNY-ESF)Abstract- Across various research domains, remotely-sensed weather products are valuable for answering many scientific questions; however, their temporal and spatial resolutions are often too coarse to answer many questions. For instance, in wildlife research, it's crucial to have fine-scaled, highly localized weather observations when studying animal movement and behavior. This paper harnesses acoustic data to identify variations in rain, wind and air temperature at different thresholds, with rain being the most successfully predicted. Training a model solely on acoustic data yields optimal results, but it demands labor-intensive sample labeling. Meanwhile, hourly satellite data from the MERRA-2 system, though sufficient for certain tasks, produced predictions that were notably less accurate in predict these acoustic labels. We find that acoustic classifiers can be trained from the MERRA-2 data that are more accurate than the raw MERRA-2 data itself. By using MERRA-2 to roughly identify rain in the acoustic data, we were able to produce a functional model without using human-validated labels. Since MERRA-2 has global coverage, our method offers a practical way to train rain and wind models using acoustic datasets around the world.
- 4. Multi-label open-set audio classification
Sripathi Sridhar, Mark Cartwright (New Jersey Institute of Technology (NJIT))Abstract- Current audio classification models typically operate with a fixed vocabulary under the “closed-set” assumption, and may miss important yet unexpected or unknown sound events. To address this, “open-set” audio classification models have been developed to also identify the presence of unknown classes, or classes not seen during training. Although these methods have been applied to multi-class contexts such as sound scene classification, they have yet to be investigated for polyphonic audio in which sound events overlap, requiring the use of multi-label models. In this study, we establish the problem of multi-label open-set audio classification by creating synthetic datasets with varying unknown class assignments, evaluating baseline models using combinations of existing techniques, and identifying potential areas of future research.
- 5. Toward Universal Speech Enhancement For Diverse Input Conditions
Wangyou Zhang, Kohei Saijo, Zhong-Qiu Wang, Shinji Watanabe, Yanmin Qian (CMU, SJTU)Abstract- The past decade has witnessed substantial growth of data-driven speech enhancement (SE) techniques thanks to deep learning. While existing approaches have shown impressive performance in some common datasets, most of them are designed only for a single condition (e.g., single-channel, multi-channel, or a fixed sampling frequency) or only consider a single task (e.g., denoising or dereverberation). Currently, there is no universal SE approach that can effectively handle diverse input conditions with a single model. In this paper, we make the first attempt to investigate this line of research. First, we devise a single SE model that is independent of microphone channels, signal lengths, and sampling frequencies. Second, we design a universal SE benchmark by combining existing public corpora with multiple conditions. Our experiments on a wide range of datasets show that the proposed single model can successfully handle diverse conditions with strong performance.
- 6. BASS - Blockwise Adaptation for Speech Summarization
Roshan Sharma, Kenneth Zheng, Siddhant Arora, Shinji Watanabe, Bhiksha Raj, Rita Singh (CMU)Abstract- End-to-end speech summarization has been shown to improve performance over cascade baselines. However, such models are difficult to train on very large inputs (dozens of minutes or hours) owing to compute restrictions and are hence trained with truncated model inputs. Truncation leads to poorer models, and a solution to this problem rests in block-wise modeling, i.e., processing a portion of the input frames at a time. In this paper, we develop a method that allows one to train summarization models on very long sequences in an incremental manner. Speech summarization is realized as a streaming process, where hypothesis summaries are updated every block based on new acoustic information. We devise and test strategies to pass semantic context across the blocks. Experiments on the How2 dataset demonstrate that the proposed block-wise training method improves by 3 points absolute on ROUGE-L over a truncated input baseline.
- 7. SLUE Phase-2: A Benchmark Suite of Diverse Spoken Language Understanding Tasks
Suwon Shon, Siddhant Arora, Chyi-Jiunn Lin, Ankita Pasad, Felix Wu, Roshan S Sharma, Wei-Lun Wu, Hung-yi Lee, Karen Livescu, Shinji Watanabe (CMU)Abstract- Spoken language understanding (SLU) tasks have been studied for many decades in the speech research community, but have not received as much attention as lower-level tasks like speech and speaker recognition. In this work, we introduce several new annotated SLU benchmark tasks based on freely available speech data, which complement existing benchmarks and address gaps in the SLU evaluation landscape. We contribute four tasks: question answering and summarization involve inference over longer speech sequences; named entity localization addresses the speech-specific task of locating the targeted content in the signal; dialog act classification identifies the function of a given speech utterance. In order to facilitate the development of SLU models that leverage the success of pre-trained speech representations, we will release a new benchmark suite, including for each task (i) curated annotations for a relatively small fine-tuning set, (ii) reproducible pipeline (speech recognizer + text model) and end-to-end baseline models and evaluation metrics, (iii) baseline model performance in various types of systems for easy comparisons. We present the details of data collection and annotation and the performance of the baseline models. We also analyze the sensitivity of pipeline models’ performance to the speech recognition accuracy, using more than 20 publicly availablespeech recognition models.
- 8. Importance of negative sampling in weak label learning
Ankit Shah, Fuyu Tang, Zelin Ye, Rita Singh, Bhiksha Raj (CMU)Abstract- Weak-label learning is a challenging task that requires learning from data "bags" containing positive and negative instances, but only the bag labels are known. The pool of negative instances is usually larger than positive instances, thus making selecting the most informative negative instance critical for performance. Such a selection strategy for negative instances from each bag is an open problem that has not been well studied for weak-label learning. In this paper, we study several sampling strategies that can measure the usefulness of negative instances for weak-label learning and select them accordingly. We test our method on CIFAR-10 and AudioSet datasets and show that it improves the weak-label classification performance and reduces the computational cost compared to random sampling methods. Our work reveals that negative instances are not all equally irrelevant, and selecting them wisely can benefit weak-label learning.
- 9. Conformers are All You Need for Visual Speech Recognition
Oscar Chang, Hank Liao, Dmitriy Serdyuk, Ankit Shah, Olivier Siohan (Google Inc)Abstract- Visual speech recognition models extract visual features in a hierarchical manner. At the lower level, there is a visual front-end with a limited temporal receptive field that processes the raw pixels depicting the lips or faces. At the higher level, there is an encoder that attends to the embeddings produced by the front-end over a large temporal receptive field. Previous work has focused on improving the visual front-end of the model to extract more useful features for speech recognition. Surprisingly, our work shows that complex visual front-ends are not necessary. Instead of allocating resources to a sophisticated visual front-end, we find that a linear visual front-end paired with a larger Conformer encoder results in lower latency, more efficient memory usage, and improved WER performance. We achieve a new state-of-the-art of 12.8% WER for visual speech recognition on the TED LRS3 dataset, which rivals the performance of audio-only models from just four years ago.
- 10. SURT 2.0: Advances in Transducer-based Multi-talker Speech Recognition
Desh Raj, Daniel Povey, and Sanjeev Khudanpur (JHU)Abstract- The Streaming Unmixing and Recognition Transducer (SURT) model was proposed recently as an end-to-end approach for continuous, streaming, multi-talker speech recognition (ASR). Despite impressive results on multi-turn meetings, SURT has notable limitations: (i) it suffers from leakage and omission related errors; (ii) it is computationally expensive, due to which it has not seen adoption in academia; and (iii) it has only been evaluated on synthetic mixtures. In this work, we propose several modifications to the original SURT which are carefully designed to fix the above limitations. In particular, we (i) change the unmixing module to a mask estimator that uses dual-path modeling, (ii) use a streaming zipformer encoder and a stateless decoder for the transducer, (iii) perform mixture simulation using force-aligned subsegments, (iv) pre-train the transducer on single-speaker data, (v) use auxiliary objectives in the form of masking loss and encoder CTC loss, and (vi) perform domain adaptation for far-field recognition. We show that our modifications allow SURT 2.0 to outperform its predecessor in terms of multi-talker ASR results, while being efficient enough to train with academic resources. We conduct our evaluations on 3 publicly available meeting benchmarks -- LibriCSS, AMI, and ICSI, where our best model achieves WERs of 16.9%, 44.6% and 32.2%, respectively, on far-field unsegmented recordings.
- 11. Kid-Whisper: Towards Bridging the Performance Gap in Automatic Speech Recognition for Children VS. Adults
Ahmed Adel Attia, Jing Liu, Wei Ai, Dorottya Demszky, Carol Espy-Wilson (University Of Maryland, Stanford University)Abstract- Transformer-based Automatic Speech Recognition (ASR) systems have been shown to approach human-level performance given sufficient data and to be robust to different kinds of noise. They still, however, do not generalize well to children's speech or common classroom noises. Recent studies have investigated fine-tuning large transformer-based ASR models, like Whisper, on children’s speech. We continue this investigation by exploiting the MyST corpus, a recently published children’s speech dataset, and the largest publicly available corpus in that regard. We reduce the Word Error Rate on the MyST testset from 13.93% to 9.11% with Whisper-Small and from 13.23% to 8.61% with Whisper-Medium and show that this improvement can be generalized to unseen datasets. We also investigate the robustness of Whisper to common classroom noises and highlight important challenges towards improving children’s ASR system.
- 12. Unsupervised Improvement of Audio-Text Cross-Modal Representations
Zhepei Wang, Cem Subakan, Krishna Subramani, Junkai Wu, Tiago Tavares, Fabio Ayres, Paris Smaragdis (U. Sherbrooke, University of Illinois, Urbana Champaign)Abstract- Recent advances in using language models to obtain cross-modal audio-text representations have overcome the limitations of conventional training approaches that use predefined labels. This has allowed the community to make progress in tasks like zero-shot classification, which would otherwise not be possible. However, learning such representations requires a large amount of human-annotated audio-text pairs. In this paper, we study unsupervised approaches to improve the learning framework of such representations with unpaired text and audio. We explore domain-unspecific and domain-specific curation methods to create audio-text pairs that we use to further improve the model. We also show that when domain-specific curation is used in conjunction with a soft-labeled contrastive loss, we are able to obtain significant improvement in terms of zero-shot classification performance on downstream sound event classification or acoustic scene classification tasks.
- 13. Harmonic Analysis with Neural Semi-CRF
Qiaoyu Yang, Frank Cwitkowitz, Zhiyao Duan (University of Rochester)Abstract- Automatic harmonic analysis of symbolic music is an important and useful task for both composers and listeners. The task consists of two components: recognizing harmony labels and finding their time boundaries. Most of the previous attempts focused on the first component, while time boundaries were rarely modeled explicitly. Lack of boundary modeling in the objective function could lead to segmentation errors. In this work, we introduce a novel approach to jointly detect the labels and boundaries of harmonic regions using neural semi-CRF (conditional random field). In contrast to rule-based scores used in traditional semi-CRF, a neural score function is proposed to incorporate features with more representational power. To improve the robustness of the model to imperfect harmony profiles, we design an additional score component to penalize the match between the candidate harmony label and the absent notes in the music.
- 14. SingFake: Singing Voice Deepfake Detection
Yongyi Zang, You Zhang, Mojtaba Heydari, Zhiyao Duan (University of Rochester)Abstract- The rise of singing voice synthesis presents critical challenges to artists and industry stakeholders over unauthorized voice usage. Unlike synthesized speech, synthesized singing voices are typically released in songs containing strong background music that may hide synthesis artifacts. Additionally, singing voices present different acoustic and linguistic characteristics from speech utterances. These unique properties make singing voice deepfake detection a relevant but significantly different problem from synthetic speech detection. In this work, we propose the singing voice deepfake detection task. We first present SingFake, the first curated in-the-wild dataset consisting of 28.93 hours of bonafide and 29.40 hours of deepfake song clips in five languages from 40 singers. We provide a train/val/test split where the test sets include various scenarios. We then use SingFake to evaluate four state-of-the-art speech countermeasure systems trained on speech utterances. We find these systems lag significantly behind their performance on speech test data. When trained on SingFake, either using separated vocal tracks or song mixtures, these systems show substantial improvement. However, our evaluations also identify challenges associated with unseen singers, communication codecs, languages, and musical contexts, calling for dedicated research into singing voice deepfake detection. The SingFake dataset and related resources are available online.
- 15. SynthTab: Leveraging Synthesized Data for Guitar Tablature Transcription
Yongyi Zang, Yi Zhong, Frank Cwitkowitz, Zhiyao Duan (University of Rochester)Abstract- Guitar tablature is a form of music notation widely used among guitarists. It captures not only the musical content of a piece, but also its implementation and ornamentation on the instrument. Guitar Tablature Transcription (GTT) is an important task with broad applications in music education and entertainment. Existing datasets are limited in size and scope, causing state-of-the-art GTT models trained on such datasets to suffer from overfitting and to fail in generalization across datasets. To address this issue, we developed a methodology for synthesizing SynthTab, a large-scale guitar tablature transcription dataset using multiple commercial acoustic and electric guitar plugins. This dataset is built on tablatures from DadaGP, which offers a vast collection and the degree of specificity we wish to transcribe. The proposed synthesis pipeline produces audio which faithfully adheres to the original fingerings, styles, and techniques specified in the tablature with diverse timbre. Experiments show that pre-training state-of-the-art GTT model on SynthTab improves transcription accuracy in same-dataset tests. More importantly, it significantly mitigates overfitting problems of GTT models in cross-dataset evaluation.
- 16. SingNet: A Real-time Singing Voice Beat and Downbeat Tracking System
Mojtaba Heydari, Ju-Chiang Wang, Zhiyao Duan (University of Rochester)Abstract- Singing voice beat and downbeat tracking posses several applications in automatic music production, analysis and manipulation. Among them, some require real-time processing, such as live performance processing and auto-accompaniment for singing inputs. This task is challenging owing to the non-trivial rhythmic and harmonic patterns in singing signals. For real-time processing, it introduces further constraints such as inaccessibility to future data and the impossibility to correct the previous results that are inconsistent with the latter ones. In this paper, we introduce the first system that tracks the beats and downbeats of singing voices in real-time. Specifically, we propose a novel dynamic particle filtering approach that incorporates offline historical data to correct the online inference by using a variable number of particles. We evaluate the performance on two datasets: GTZAN with the separated vocal tracks, and an in-house dataset with the original vocal stems. Experimental result demonstrates that our proposed approach outperforms the baseline by 3–5%
- 17. Parakeet: An End-to-End, Natural Text-to-Speech System
Jordan Darefsky, Ge Zhu, Zhiyao Duan (University of Rochester)Abstract- Current text-to-speech (TTS) systems tend to consist of multiple modules. In this paper, we demonstrate that a simple autoregressive transformer, trained to predict audio codes conditioned on raw, byte-level text, can generate fluent speech. Our dataset includes 60,000 hours of initially unsupervised podcast data, which allows us to synthesize multi-speaker dialogue. We backtranslate this audio with a Whisper model fine-tuned to produce speaker annotations. After training our model, we investigate the use of classifier-free guidance and introduce a novel modification to improve sampling. Lastly, our model possesses zero-shot voice-cloning capability as a result of our simplified training objective.
- 18. Cacophony: improving CLAP through bootstrapping, pre-pretraining and captioning
Ge Zhu, Jordan Darefsky, Zhiyao Duan (University of Rochester)Abstract- Compared to recent successes in training image-text linking models like Contrastive Language Image Pretraining (CLIP), the training of contrastive audio-languge models presents two directions for improvement. First, the scale and purity of training data (audio-text pairs) needs to be greatly improved. Second, novel neural architectures to model audio structures and training strategies are needed. We propose to use data bootstrapping strategy with large language models and state-of-the-art audio captioning models for data collection. We use masked autoencoder for pre-pretraining and introduce an auxiliary captioning objective for better audio-text alignment. With proposed approaches, we achieved notable improvement over the vanilla CLAP models.
- 19. EDMSound: Spectrogram Based Diffusion Models for Efficient and High-Quality Audio Synthesis
Ge Zhu, Yutong Wen, Marc Carbonneau, Zhiyao Duan (University of Rochester, Ubisoft)Abstract- Diffusion models have showcased their capabilities in audio synthesis ranging over a variety of sounds. While generating audio with creativity, existing models often operate on the latent domain with cascaded phase recovery modules to reconstruct waveform. It potentially introduces challenges in generating high-fidelity audio. In this paper, we propose EDMSound, a diffusion-based generative model in spectrogram domain under the framework of elucidated diffusion models (EDM). Combining with efficient deterministic sampler, we achieved similar Fréchet audio distance (FAD) score as top-ranked baseline with only 10 steps and reached state-of-the-art performance with 50 steps on the DCASE2023 foley sound generation benchmark. We also revealed a potential concern regarding diffusion based audio generation models that they tend to generate samples with high perceptual similarity to the data from training data. Project page: https://tinyurl.com/4rds3bnn
- 20. Grid-Agnostic Personalized Head-Related Transfer Function (HRTF) Modeling with Implicit Neural Representations
You Zhang, Yutong Wen, Yuxiang Wang, Mark Bocko, Zhiyao Duan (University of Rochester)Abstract- The spatial filtering effect brought on by sound propagation from the sound source to the outer ear is referred to as the head-related transfer function (HRTF). The personalization of HRTF is essential to enhance the personalized immersive audio experience in virtual and augmented reality. Our work aims to employ deep learning to predict the customized HRTF from anthropometric measurements. However, existing measured HRTF databases each employ a different geographic sampling, making it difficult to combine these databases into training data-hungry deep learning methods while each of them only contains dozens of subjects. Following our previous work, we use a neural field, a neural network that maps the spherical coordinates to the magnitude spectrum to represent each subject’s set of HRTFs. We constructed a generative model to learn the latent space across subjects using such a consistent representation of HRTF across datasets. In this work, by learning the mapping of the anthropometric measurements to the latent space and then reconstructing the HRTF, we further investigate the neural field representation to carry out HRTF personalization. Thanks to the grid-agnostic nature of our method, we are able to train on combined datasets and even validate the performance on grids unseen during training.
- 21. Estimating Virtual Microphones for Active Noise Control
Manan Mittal, Yongjie Zhuang, Paris Smaragdis, Ryan Corey, Andrew Singer (University of Illinois, Urbana Champaign, Stony Brook University, University of Illinois, Chicago)Abstract- In many active sound control problems of interest, it is difficult to place sensors precisely at desired noise control locations. In such cases, it can be useful to estimate the noise field at the locations of interest and use that estimate in standard active noise cancelation algorithms like Filtered-x Least Mean Square (FxLMS). Instead of using a single point in the calculation of the residual noise at the desired location or region, we can now estimate the residual noise at numerous “virtual” microphones. Neural networks have been used in many applications related to sound field interpolation and acoustic transfer function estimation. Most recently, neural acoustic fields have been successful in modeling the sound field in various environments. A neural acoustic field is proposed to interpolate and estimate the noise field at unknown locations in the volume of interest. The method is tested in a simulated environment inside a duct.
- 22. AV2Wav: Diffusion-Based Re-synthesis from Continuous Self-supervised Features for Audio-Visual Speech Enhancement
Ju-Chieh Chou, Chung-Ming Chien, Karen Livescu (TTIC)Abstract- Speech enhancement systems are typically trained using pairs of clean and noisy speech. In audio-visual speech enhancement (AVSE), there is not as much ground-truth clean data available; most audio-visual datasets are collected in real-world environments with background noise and reverberation, hampering the development of AVSE. In this work, we introduce AV2Wav, a resynthesis-based audio-visual speech enhancement approach that can generate clean speech despite the challenges of real-world training data. We obtain a subset of nearly clean speech from an audio-visual corpus using a neural quality estimator, and then train a diffusion model on this subset to generate waveforms conditioned on continuous speech representations from AV-HuBERT with noise-robust training. We use continuous rather than discrete representations to retain prosody and speaker information. With this vocoding task alone, the model can perform speech enhancement better than a masking-based baseline. We further fine-tune the diffusion model on clean/noisy utterance pairs to improve the performance. Our approach outperforms a masking-based baseline in terms of both automatic metrics and a human listening test and is close in quality to the target speech in the listening test. Audio samples can be found at this https URL.
- 23. What do self-supervised speech models know about words?
Ankita Pasad, Chung-Ming Chien, Shane Settle, and Karen Livescu (TTIC)Abstract- Many self-supervised speech models (S3Ms) have been introduced over the last few years, improving performance and data efficiency on various speech tasks; but these empirical successes alone do not give a complete picture of what is learned during pre-training. Recent work has begun analyzing how S3Ms encode certain properties, such as phonetic and speaker information, but we still lack a proper understanding of knowledge encoded at the word level and beyond. In this work, we use lightweight analysis methods to study segment-level linguistic properties---word identity, boundaries, pronunciation, syntactic features, and semantic features---encoded in S3Ms. We present a comparative study of layer-wise representations from ten S3Ms and find that the frame-level representations within each word segment are not all equally informative, and the accessibility and distribution of linguistic information across layers is heavily influenced by the pre-training objective and model size. We also find that on several tasks---word discrimination, word segmentation, and semantic sentence similarity---S3Ms trained with visual grounding outperform their speech-only counterparts. Finally, our task-based analyses demonstrate an improved performance on word segmentation and acoustic word discrimination, while using simpler methods than prior work.
- 24. The Potential of Neural Speech Synthesis-based Data Augmentation for Personalized Speech Enhancement
Anastasia Kuznetsova, Aswin Sivaraman, Minje Kim (Indiana University)Abstract- With the advances in deep learning, speech enhancement systems benefited from large neural network architectures and achieved state-of-the-art quality. However, speaker-agnostic methods are not always desirable, both in terms of quality and their complexity, when they are to be used in a resource-constrained environment. One promising way is personalized speech enhancement (PSE), which is a smaller and easier speech enhancement problem for small models to solve, because it focuses on a particular test-time user. To achieve the personalization goal, while dealing with the typical lack of personal data, we investigate the effect of data augmentation based on neural speech synthesis (NSS). In the proposed method, we show that the quality of the NSS system's synthetic data matters, and if they are good enough the augmented dataset can be used to improve the PSE system that outperforms the speaker-agnostic baseline. The proposed PSE systems show significant complexity reduction while preserving the enhancement quality.
- 25. Improving Transcription Quality in Spanish: A Hybrid Acoustic-Lexical System for Punctuation Restoration
Xiliang Zhu, Chia-Tien Chang, Shayna Gardiner, David Rossouw, Jonas Robertson (Dialpad)Abstract- Punctuation restoration is a crucial step after an ASR system to enhance transcript readability for NLP tasks. Conventional lexical-based approaches are inadequate for solving this task in Spanish. We introduce a novel hybrid acoustic-lexical punctuation restoration system that consolidates acoustic and lexical signals through a modular process. The proposed system improves the F1 score of question marks and overall punctuation restoration on both public and internal Spanish conversational datasets. Furthermore, our approach outperforms LLMs in terms of accuracy, reliability, and latency. Additionally, the WER of the ASR module also benefits.
- 26. Analyzing the latency and stability of streaming end-to-end speech recognition models
Daniil Kulko, Shreekantha Nadig, Simon Vandieken, Riqiang Wang, Jonas Robertson (Dialpad)Abstract- Real-time conversational AI has become more common in consumer products thanks to progress in deep learning-based automatic speech recognition (ASR) and natural language processing (NLP) methods. However, some of these real-time use cases have tight computational, latency, and stability requirements which can be challenging to achieve with a real-time streaming application. Improving one of these often leads to the degradation of the other metric. Streaming ASR models output words incrementally while the user is speaking, which can be referred to as a “partial hypothesis”, and eventually stabilize over time and are called a “final hypothesis” when they stay fixed in time. In this poster, we compare how the hypothesis delay and stability of the partial hypothesis depend on different ASR architectures and decoding strategies, and propose methods to optimize stability vs. latency trade-off for a real-time ASR application.
- 27. Enhancing Multilingual Speech Recognition for Video Game Voice Chat: Leveraging Unsupervised Pre-training and Pseudo-labeling with Whisper
J.D. Fishman, Raquel Harrison, Angel Carrillo-Bermejo, Rachel Manzelli (Modulate)Abstract- The advancement of speech recognition technology has received a significant boost from the emergence of unsupervised pre-training methods, as exemplified by wav2vec2.0. These techniques enable learning directly from raw audio data without the need for human-annotated labels, making it possible to leverage large amounts of unlabeled speech data. However, when addressing the specific challenges posed by video game voice chat data, the complexity of the problem increases due to the presence of underexplored irregularities in the data distribution. One conceivable approach is to finetune a pretrained model using ground truth labels derived from existing in-domain data. Nonetheless, the labor-intensive process of manually generating labeled data poses a formidable obstacle to this approach. In the context of this research, we propose harnessing the existing Whisper model to produce “pseudolabels” for the development of our in-domain dataset, which we use to finetune a pretrained wav2vec2.0 model for the English, Spanish and Portuguese languages. We then separately finetune with a smaller amount of human-labeled (ground truth) data in each language as a comparison point. We then proceed to evaluate each model’s proficiency in managing the intricacies of voice chat data in video games. These experiments reveal that utilizing a large amount of Whisper pseudolabels to finetune results in a significant decrease in the word error rate (WER) across our in-domain test datasets. Additionally, we see that finetuning with manually annotated transcripts, even in small amounts, have significant influence on WER. With these results, we are able to conclude that there is a balance to strike between quantity and quality of data when finetuning speech recognition models.
- 28. 1-step Speech Understanding and Transcription using CTC Loss
Karan Singla, Shahab Jalalvand, Yeon-Jun Kim, Srinivas Bangalore, Ben Stern (Whissle-AI, Interactions LLC)Abstract- Our work presents a method using non-regressive CTC loss to annotate speech and natural language events in spoken conversations. Proposed approach seamlessly generates event tags and transcription tokens from streaming speech. We show leveraging conversational context and event tags improves SLU and transcription accuracy. We provide results for the public domain SLUE benchmark, along with visual evidence from the SLURP corpus and a two-channel caller-agent conversation. We also delve into the impact of fine-tuning pre-trained speech encoders to directly extract spoken entities without the need for text transcription. This approach optimizes the encoder to transcribe only entity-relevant segments of speech, disregarding superfluous elements like carrier phrases or spelled-out name entities. In the context of enterprise virtual agent dialogues, we demonstrate that the 1-step approach surpasses the typical 2-step method.
- 29. CoLLD: Contrastive Layer-to-layer Distillation for Compressing Multilingual Pre-trained Speech Encoders
Heng-Jui Chang, Ning Dong, Ruslan Mavlyutov, Sravya Popuri, Yu-An Chung (MIT, Meta)Abstract- Large-scale self-supervised pre-trained speech encoders outperform conventional approaches in speech recognition and translation tasks. Due to the high cost of developing these large models, building new encoders for new tasks and deploying them to on-device applications are infeasible. Prior studies propose model compression methods to address this issue, but those works focus on smaller models and less realistic tasks. Thus, we propose Contrastive Layer-to-layer Distillation (CoLLD), a novel knowledge distillation method to compress pre-trained speech encoders by leveraging masked prediction and contrastive learning to train student models to copy the behavior of a large teacher model. CoLLD outperforms prior methods and closes the gap between small and large models on multilingual speech-to-text translation and recognition benchmarks.
- 30. Audio-Visual Neural Syntax Acquisition
Cheng-I Jeff Lai, Freda Shi, Puyuan Peng, Yoon Kim, Kevin Gimpel, Shiyu Chang, Yung-Sung Chuang, Saurabhchand Bhati, David Daniel Cox, David Harwath, Yang Zhang, Karen Livescu, James R Glass (MIT, TTIC, UT Austin, UCSB, JHU, MIT-IBM AI Lab)Abstract- We study phrase structure induction from visually-grounded speech. The core idea is to first segment the speech waveform into sequences of word segments, and subsequently induce phrase structure using the inferred segment-level continuous representations. We present the Audio-Visual Neural Syntax Learner (AV-NSL) that learns phrase structure by listening to audio and looking at images, without ever being exposed to text. By training on paired image and spoken captions, AV-NSL exhibits the capability to infer meaningful phrase structures that are comparable to those derived by naturally-supervised text parsers, for both English and German. Our findings extend prior work in unsupervised language acquisition from speech and grounded grammar induction, and present one approach to bridge the gap between the two fields.
- 31. Instruction-Following Speech Recognition
Cheng-I Jeff Lai, Zhiyun Lu, Liangliang Cao, Ruoming Pang (Apple, MIT )Abstract- Conventional end-to-end Automatic Speech Recognition (ASR) models primarily focus on exact transcription tasks, lacking flexibility for nuanced user interactions. With the advent of Large Language Models (LLMs) in speech processing, more organic, text-prompt-based interactions have become possible. However, the mechanisms behind these models' speech understanding and "reasoning" capabilities remain underexplored. To study this question from the data perspective, we introduce instruction-following speech recognition, training a Listen-Attend-Spell model to understand and execute a diverse set of free-form text instructions. This enables a multitude of speech recognition tasks -- ranging from transcript manipulation to summarization -- without relying on predefined command sets. Remarkably, our model, trained from scratch on Librispeech, interprets and executes simple instructions without requiring LLMs or pre-trained speech modules. It also offers selective transcription options based on instructions like "transcribe first half and then turn off listening," providing an additional layer of privacy and safety compared to existing LLMs. Our findings highlight the significant potential of instruction-following training to advance speech foundation models.
- 32. Understanding Cochlear Implants Using Machine Learning
Annesya Banerjee, Mark Saddler, Josh McDermott (Harvard University, MIT)Abstract- Current cochlear implants (CIs) fail to restore fully normal auditory perception in individuals with sensorineural deafness. Several factors may limit CI outcomes, including suboptimal algorithms for converting sound into electrical stimulation, plasticity limitations of the central auditory system, and auditory nerve degeneration. Models that can predict the information that can be derived from CI stimulation could help clarify the role of these different factors and guide development of better stimulation strategies. We investigated models of CI-mediated hearing based on deep artificial neural networks, which have recently been shown to reproduce aspects of normal hearing behavior and hierarchical organization in the auditory system. To model normal auditory perception, we trained a deep neural network to perform real-world auditory tasks (word recognition and sound localization) using simulated auditory nerve input from an intact cochlea. We modeled CI hearing by testing this same trained network on simulated auditory nerve responses to CI stimulation. To simulate the possible consequences of learning to hear through a CI, we retrained this network on CI input. Further, to model the possibility that only part of the auditory system exhibits this plasticity, in some models we retrained only the late stages of the network. When the entire network was reoptimized for CI input, the model exhibited speech intelligibility scores significantly better than typical CI users. Speech recognition on par with typical CI users was achieved only when just the late stages of the models were reoptimized. However, for sound localization, model performance remained abnormal relative to normal hearing even when the entire network was reoptimized for CI input. Overall, this work provides initial validation of machine-learning-based models of CI-mediated perception. Our results help clarify the interplay of impoverished peripheral representation from CI stimulation and incomplete central plasticity in limiting CI user performance of realistic auditory tasks.
- 33. Hyperbolic Unsupervised Anomalous Sound Detection
François G. Germain, Gordon Wichern, Jonathan Le Roux (MERL)Abstract- We introduce a framework to perform unsupervised anomalous sound detection by leveraging embeddings learned in hyperbolic space. Previously, hyperbolic spaces have demonstrated the ability to encode hierarchical relationships much more effectively than Euclidean space when using those embeddings for classification. A corollary of that property is that the distance of a given embedding from the hyperbolic space origin encodes a notion of classification certainty, naturally mapping inlier class samples to the space edges and outliers near the origin. As such, we expect the hyperbolic embeddings generated by a deep neural network pre-trained to classify short-time Fourier transform frames of normal machine sounds to be more distinctive than Euclidean embeddings when attempting to identify unseen anomalous data. In particular, we show here how to perform unsupervised anomaly detection using embeddings from a trained modified MobileFaceNet architecture with a hyperbolic embedding layer, using the embeddings generated from a test sample to generate an anomaly score. Our results show that the proposed approach outperforms similar methods in Euclidean space on the DCASE 2022 Unsupervised Anomalous Sound Detection dataset.
- 34. Two-Step Knowledge Distillation for Tiny Speech Enhancement
Rayan Daod Nathoo*, Mikolaj Kegler*, Marko Stamenovic (Bose Corporation, USA)Abstract- Tiny, causal models are crucial for embedded audio machine learning applications. Model compression can be achieved via distilling knowledge from a large teacher into a smaller student model. In this work, we propose a novel two-step approach for tiny speech enhancement model distillation. In contrast to the standard approach of a weighted mixture of distillation and supervised losses, we firstly pre-train the student using only the knowledge distillation (KD) objective, after which we switch to a fully supervised training regime. We also propose a novel fine-grained similarity-preserving KD loss, which aims to match the student's intra-activation Gram matrices to that of the teacher. Our method demonstrates broad improvements, but particularly shines in adverse conditions including high compression and low signal to noise ratios (SNR), yielding signal to distortion ratio gains of 0.9 dB and 1.1 dB, respectively, at -5 dB input SNR and 63x compression compared to baseline.
- 35. Latent CLAP Loss for Improving Foley Sound Synthesis
Tornike Karchkhadze*, Hassan Salami Kavaki*, Russell Izadi, Bryce Irvin, Mikolaj Kegler, Shuo Zhang, Marko Stamenovic (UCSD, CUNY, BOSE Corporation)Abstract- Foley sound generation, the art of creating audio for multimedia, has recently seen notable advancements through text-conditioned latent diffusion models (LDM). Current LDMs often employ pre-trained multimodal text-audio representation models, such as Contrastive Language-Audio Pretraining (CLAP), whose objective is to map corresponding audio and text prompts into a joint embedding space. The text-to-audio AudioLDM model was the winner of the 2023 DCASE task 7 Foley sound synthesis challenge. Part of the method involved fine-tuning the model on a set of defined classes and post-filtering generated samples until similarity between the model output and the input text prompt in the CLAP embedding space was satisfied. While post-filtering was shown to improve output quality, it also substantially reduces data generation efficiency. Here, we propose a novel loss term for improving the Foley sound generation quality using AudioLDM without post-filtering. The proposed loss term involves a latent CLAP model optimized to map the output of the latent diffusion step and the text prompt into a common embedding space. Our results show that minimizing the distance between the two in the latent CLAP space at training time lowers Fréchet Audio Distance (FAD) metric of the generated audio while also increases its similarity score with corresponding class embedding at inference, alleviating the need for post-filtering. Through experiments and ablations studies, we demonstrate that the proposed method yields superior generation quality, improves correspondence with input text prompts, and reduces data generation time. Our system, employing the proposed latent CLAP loss, achieves a ten-fold reduction in generation time without compromising output quality, compared to current state of the art Foley sound synthesis models with post-filtering.
- 36. Learning Text-queried Sound Separation and Synthesis using Unlabeled Videos and Pretrained Language-Vision Models
Hao-Wen Dong (UCSD)Abstract- Contrastive language-image pretraining (CLIP) has revolutionized multimodal learning and showed remarkable generalizability in many downstream tasks. While similar attempts have been made to build a counterpart model for language and audio, it remains unclear whether we can scale up text-audio datasets to a size comparable to large-scale text-image datasets. In this poster, I will present my recent work on text-audio data free training for text-queried sound separation and text-to-audio synthesis. Leveraging the visual modality as a bridge, the proposed models learn the desired text-audio correspondence by combining the naturally-occurring audio-visual correspondence in videos and the multimodal representation learned by pretrained language-vision models. This offers a new direction of approaching bimodal learning for text and audio through leveraging the visual modality as a bridge, which can further be scaled up to large unlabeled video datasets in the wild.
- 37. Music De-limiter Networks via Sample-wise Gain Inversion
Chang-Bin Jeon and Kyogu Lee (Seoul National University)Abstract- The loudness war, an ongoing phenomenon in the music industry characterized by the increasing final loudness of music while reducing its dynamic range, has been a controversial topic for decades. Music mastering engineers have used limiters to heavily compress and make music louder, which can induce ear fatigue and hearing loss in listeners. In this paper, we introduce music de-limiter networks that estimate uncompressed music from heavily compressed signals. Inspired by the principle of a limiter, which performs sample-wise gain reduction of a given signal, we propose the framework of sample-wise gain inversion (SGI). We also present the musdb-XL-train dataset, consisting of 300k segments created by applying a commercial limiter plug-in for training real-world friendly de-limiter networks. Our proposed de-limiter network achieves excellent performance with a scale-invariant source-to-distortion ratio (SI-SDR) of 23.8 dB in reconstructing musdb-HQ from musdb- XL data, a limiter-applied version of musdb-HQ. The training data, codes, and model weights are available in our repository (this https URL).
- 38. Towards Sound Synthesis using Physical Modeling and Differentiable Finite Difference Schemes
Jin Woo Lee, Kyogu Lee (Seoul National University)Abstract- We study methods to synthesize sounds using a “differentiable” finite difference scheme (or finite difference time-domain; FDTD). In our exploration, we are pursuing two distinct approaches: To train a neural operator that approximates the finite difference, or just to implement a finite difference scheme supported with tractable gradient back-propagations. To alleviate these, we implement the string sound FDTD simulator using PyTorch and JAX. We further aim to train autoencoders, using the FDTD solvers as decoders.
- 39. HyPoradise: An Open Baseline for Generative Speech Recognition with Large Language Models
Chen Chen, Yuchen Hu, Chao-Han Huck Yang, Sabato Macro Siniscalchi, Pin-Yu Chen, Eng Siong Chng (Nanyang Technological University, Georgia Institute of Technology, Norwegian University of Science and Technology, IBM Research AI)Abstract- Humans address this issue by relying on their linguistic knowledge: the meaning of ambiguous spoken terms is usually inferred from contextual cues thereby reducing the dependency on the auditory system. Inspired by this observation, we introduce the first open-source benchmark to utilize external large language models (LLMs) for ASR error correction, where N-best decoding hypotheses provide informative elements for true transcription prediction. This approach is a paradigm shift from the traditional language model rescoring strategy that can only select one candidate hypothesis as the output transcription. The proposed benchmark contains a novel dataset, HyPoradise (HP), encompassing more than 334,000 pairs of N-best hypotheses and corresponding accurate transcriptions across prevalent speech domains. Given this dataset, we examine three types of error correction techniques based on LLMs with varying amounts of labeled hypotheses-transcription pairs, which gains a significant word error rate (WER) reduction. Experimental evidence demonstrates the proposed technique achieves a breakthrough by surpassing the upper bound of traditional re-ranking based methods. More surprisingly, LLM with reasonable prompt and its generative capability can even correct those tokens that are missing in N-best list. We make our results publicly accessible for reproducible pipelines with released pre-trained models, thus providing a new evaluation paradigm for ASR error correction with LLMs.
- 40. Neural Audio Decorrelation using Convolutional Neural Networks
Carlotta Anemüller, Oliver Thiergart, and Emanuël A. P. Habets (International Audio Laboratories Erlangen)Abstract- The degree of correlation between two audio signals entering the ears has a significant impact on the perception of spatial sound. As a result, audio signal decorrelation is widely used in various applications in the field of spatial audio rendering. In this study, we present a recently proposed convolutional neural network architecture for audio decorrelation. The model is trained using two different approaches. Firstly, a supervised training approach is used, where a state-of-the-art reference decorrelator is used as a reference. Secondly, a reference-free training approach based on generative adversarial networks is used. The training objective includes a number of individual loss terms that control both the correlation between the output and input signals, as well as the quality of the output signal. The obtained models from both training approaches are objectively and subjectively evaluated, considering a variety of signal types.
- 41. New Insights on Target Speaker Extraction
Mohamed Elminshawi, Wolfgang Mack, Srikanth Raj Chetupalli, Soumitro Chakrabarty, Emanuël A. P. Habets (International Audio Laboratories Erlangen)Abstract- Speaker extraction (SE) aims to segregate the speech of a target speaker from a mixture of interfering speakers with the help of auxiliary information. Several forms of auxiliary information have been employed in single-channel SE, such as a speech snippet enrolled from the target speaker or visual information corresponding to the spoken utterance. The effectiveness of the auxiliary information in SE is typically evaluated by comparing the extraction performance of SE with uninformed speaker separation (SS) methods. Following this evaluation protocol, many SE studies have reported performance improvement compared to SS, attributing this to the auxiliary information. However, such studies have been conducted on a few datasets and have not considered recent deep neural network architectures for SS that have shown impressive separation performance. In this paper, we examine the role of the auxiliary information in SE for different input scenarios and over multiple datasets. Specifically, we compare the performance of two SE systems (audio-based and video-based) with SS using a common framework that utilizes the recently proposed dual-path recurrent neural network as the main learning machine. Experimental evaluation on various datasets demonstrates that the use of auxiliary information in the considered SE systems does not always lead to better extraction performance compared to the uninformed SS system. Furthermore, we offer insights into the behavior of the SE systems when provided with different and distorted auxiliary information given the same mixture input.
- 42. Single Channel Speech Enhancement with Normalizing Flows
Martin Strauss, Bernd Edler (International Audio Laboratories Erlangen)Abstract- Deep generative models for Speech Enhancement (SE) received increasing attention in recent years. The most prominent examples include Generative Adversarial Networks (GANs), Denoising Diffusion Probabilistic Models (DDPMs) and Normalizing Flows (NFs). In this work we present an overview of the progress made in NF-based single channel SE from a purely time domain processing technique to improvements motivated by human hearing and a combination with GANs. Hereby, the denoising performance is competitive with other state-of-the-art models including GANs and DDPMs. Overall, this approach offers stable training and efficient high-quality SE, while maintaining the capability to estimate the log-likelihood of a given input.
- 43. A Mathematical Analysis of Temporal Noise Shaping for Transform Audio Coding
Richard Füg (International Audio Laboratories Erlangen)Abstract- Temporal Noise Shaping (TNS) based on Linear Predictive Coding (LPC) in the Modified Discrete Cosine Transform (MDCT) domain is a tool employed in many state-of-the-art transform audio codecs to avoid the pre-echo artifact. Despite its widespread usage only limited mathematical analysis of TNS has been done. In this work an in-depth analysis of TNS as standardized in Advanced Audio Coding (AAC) is presented. Highlight is put on important results for future research.
- 44. Efficient Deep Acoustic Echo Suppression with Condition-Aware Training
Ernst Seidel, Pejman Mowlaee, Tim Fingscheidt (Technische Universität Braunschweig)Abstract- The topic of deep acoustic echo control (DAEC) has seen many approaches with various model topologies in recent years. Convolutional recurrent networks (CRNs), consisting of a convolutional encoder and decoder encompassing a recurrent bottleneck, are repeatedly employed due to their ability to preserve nearend speech even in double-talk (DT) condition. However, past architectures are either computationally complex or trade off smaller model sizes with a decrease in performance. We propose an improved CRN topology which, compared to other realizations of this class of architectures, not only saves parameters and computational complexity, but also shows improved performance in DT, outperforming both baseline architectures FCRN and CRUSE. Striving for a condition-aware training, we also demonstrate the importance of a high proportion of double-talk and the missing value of nearend-only speech in DAEC training data. Finally, we show how to control the trade-off between aggressive echo suppression and near-end speech preservation by fine-tuning with condition-aware component loss functions.
- 45. Ultra Low Delay Audio Source Separation using Zeroth-Order Optimization
Gerald Schuller (TU Ilmenau)Abstract- In this poster, the "Random Directions" probabilistic optimization method is shown, demonstrating its efficacy in real-time, low-latency signal processing applications. Applied to an ultra-low delay, time-domain, multichannel source separation system, the "Random Directions" method is compared with the gradient-based method "Trinicon" and frequency domain methods like AuxIVA and FastMNMF. Results indicate that this approach often outperforms Trinicon in terms of the Signal to Interference Ratio (SIR) and presents the least non-linear distortions among all methods, as measured by the Signal to Artifacts Ratio (SAR). This study suggests that probabilistic optimization methods, traditionally perceived as slow, can indeed be effective for real-time applications.
- 46. CHiME-7 DASR Challenge Results and Future Directions
Samuele Cornell (Marche Polytechnic University)Abstract- The CHiME challenges have had a substantial impact on the advancement and assessment of robust automatic speech recognition (ASR) systems. In this line of research, the recent CHiME-7 DASR challenge focused on joint ASR and diarization (SD+ASR) in far-field settings with multiple recording devices on 3 different scenarios: CHiME-6, DiPCo and Mixer 6. The goal of this challenge was to encourage participants to develop robust SD+ASR systems that can generalize to multiple scenarios, a highly desirable characteristic for real-world transcription applications. The top-performing team achieved an impressive 30% relative reduction in concatenated minimum-permutation word error rate (cpWER) compared to the previous best CHiME-6 results. Such improvement was mostly possible by the use of self-supervised learning (SSL) large-scale pretrained models, end-to-end ASR and novel diarization techniques, while the overall contribution of participants regarding novel front-end techniques was limited. Our plan for the future iteration of DASR includes expanding the evaluation scenarios by also considering single-channel recordings and short meetings, having fully blind evaluation and adding constraints on computational complexity to discourage ensemble approaches. We firmly believe that a challenging, application-realistic, and blind benchmark dataset is essential for accurately assessing the current state and fostering advancements in meeting transcription research.
- 47. Instabilities in Raw Audio Convnets
Daniel Haider, Vincent Lostanlen, Martin Ehler, Peter Balazs (LS2N/CNRS)Abstract- What makes waveform-based deep learning so hard? Despite numerous attempts at training convolutional neural networks (convnets) for filterbank design, they often fail to outperform hand-crafted baselines. These baselines are linear time-invariant systems: as such, they are approximable by convnets with wide receptive fields. Yet, in practice, gradient-based optimization leads to a suboptimal approximation. Our article attributes this phenomenon to a poor choice of initialization. We present a theory of large deviations for the energy response of FIR filterbanks with random Gaussian weights. We find that deviations worsen for large filters and locally periodic input signals, which both are typical of audio signal processing applications. Numerical simulations align with our theory and suggest that the condition number of a convolutional layer follows a logarithmic scaling law between number of filters and receptive field size, which is reminiscent of discrete wavelet bases.
- 48. Nonnegative Tucker Decomposition, applied to Music Information Retrieval
Axel Marmoret, Jérémy E. Cohen, Frédéric Bimbot (IMT Atlantique, Univ Rennes, Inria, CNRS)Abstract- Nonnegative Tucker Decomposition (NTD) is a tensor factorization technique, studied in literature as a multilinear dimensionality reduction technique. Applied to barwise representation of a song, NTD is able to uncover barwise audio patterns, in an unsupervised way. These patterns can in turn be used as a way to compose new songs, or as a tool for Music Information Retrieval (MIR), in particular in the context of Music Structure Analysis. By presenting the mathematical foundation of NTD and its interpretation in the context of barwise music analysis, this poster aims at sharing current research about this technique and encouraging the Music Information Retrieval community to discover and investigate this technique. As this technique shares grounds with Nonnegative Matrix Factorization, largely studied in the MIR community in the last decades, we believe that NTD could benefit from this past work, and that it has room for improvement and exploration (in particular in the context of Unsupervised Source Separation, or, in a broader perspective, in paradigms mixing Low-Rank Factorization and Deep Neural Networks).
- 49. Recognise and Notify Sound Events using a Raspberry PI based Standalone Device
Gabriel Bibbó, Arshdeep Singh, Mark Plumbley (University of Surrey)Abstract- Convolutional neural networks (CNNs) have exhibited state-of-the-art performance in various audio classification tasks. However, their real-time deployment remains a challenge on resource-constrained devices like embedded systems. In this paper, we present a demonstration of our standalone hardware device designed for real-time recognition of sound events commonly known as audio tagging. Our system incorporates a real-time implementation of a CNN-based pre-trained audio neural networks (PANNs) on an embedded hardware device, Raspberry Pi. We refer to our standalone device as PiSoundSensing system, which makes sense of surrounding sounds using a Raspberry Pi based hardware. Users can interact with the system through a physical button or using an online web interface. The web interface allows users to remotely control the standalone device, and visualize sound events detected over time. We provide a detailed description of the hardware and software used to build PiSoundSensing device. Also, we highlight useful observations including hardware-based standalone device performance compared to that of the software-based performance.
- 50. E-PANNs: An efficient version of pre-trained audio neural network (PANNs) for audio tagging
Arshdeep Singh, Haohe Liu, Mark D. Plumbley (University of Surrey)Abstract- Sounds carry an abundance of information about activities and events in our everyday environment, such as traffic noise, road works, music, or people talking. Recent machine learning methods, such as convolutional neural networks (CNNs), have been shown to be able to automatically recognize sound activities, a task known as audio tagging. One such method, pre-trained audio neural networks (PANNs), provides a neural network which has been pre-trained on over 500 sound classes from the publicly available AudioSet dataset, and can be used as a baseline or starting point for other tasks. However, the existing PANNs model has a high computational complexity and large storage requirement. This could limit the potential for deploying PANNs on resource-constrained devices, such as on-the-edge sound sensors, and could lead to high energy consumption if many such devices were deployed. In this poster, we reduce the computational complexity and memory requirement of the PANNs model by taking a pruning approach to eliminate redundant parameters from the PANNs model. The resulting Efficient PANNs (E-PANNs) model, which requires 36% less computations and 70% less memory, also slightly improves the sound recognition (audio tagging) performance.
- 51. Unifying The Discrete and Continuous Emotion labels for Speech Emotion Recognition
Hira Dhamyal, Roshan Sharma, Bhiksha Raj, Rita Singh (CMU)Abstract- Traditionally, in paralinguistic analysis for emotion detection from speech, emotions have been identified with discrete or dimensional (continuous-valued) labels. Accordingly, models that have been proposed for emotion detection use one or the other of these label types. However, psychologists like Russell and Plutchik have proposed theories and models that unite these views, maintaining that these representations have shared and complementary information. This paper is an attempt to validate these viewpoints computationally. To this end, we propose a model to jointly predict continuous and discrete emotional attributes and show how the relationship between these can be utilized to improve the robustness and performance of emotion recognition tasks. Our approach comprises multi-task and hierarchical multi-task learning frameworks that jointly model the relationships between continuous-valued and discrete emotion labels. Experimental results on two widely used datasets (IEMOCAP and MSPPodcast) for speech-based emotion recognition show that our model results in statistically significant improvements in performance over strong baselines with non-unified approaches. We also demonstrate that using one type of label (discrete or continuous valued) for training improves recognition performance in tasks that use the other type of label. Experimental results and reasoning for this approach (called mis-matched training approach) are also presented