SANE 2024 - Speech and Audio in the Northeast
October 17, 2024
The workshop is now over. Videos and slides for the talks are available through the links in the schedule below. There is also a YouTube Playlist for all talks.
SANE 2024, a one-day event gathering researchers and students in speech and audio from the Northeast of the American continent, was held on Thursday October 17, 2024 at Google, in Cambridge, MA.
It was the 11th edition in the SANE series of workshops, which started in 2012 and is typically held every year alternately in Boston and New York. Since the first edition, the audience has steadily grown, with a new record of 200 participants and 53 posters in 2024.
SANE 2024 featured invited talks by leading researchers from the Northeast as well as from the wider community. It also featured a lively poster session, open to both students and researchers.
Details
- Date: Thursday, October 17, 2024
- Venue: Google, 325 Main St, Cambridge, MA.
Schedule [Watch all recorded talks on YouTube]
Click on the talk title to jump to the abstract and bio.8:30-9:05 | Registration and Breakfast |
9:05-9:10 | Welcome [Youtube] [Slides] |
9:10-10:00 | Quan Wang (Google) Speaker diarization at Google: From modularized systems to LLMs [Youtube] [Slides] |
10:00-10:50 | Greta Tuckute (MIT) Computational models of auditory and language processing in the human brain [Youtube] [Slides] |
10:50-11:20 | Coffee break |
11:20-12:10 | Mark Hamilton (MIT) Separating the "chirp" from the "chat": Self-supervised visual grounding of sound and language [Youtube] [Slides] |
12:10-13:00 | Bhuvana Ramabhadran (Google) Multilingual Speech Representations [Youtube] [Slides] |
13:00-13:30 | Lunch |
13:30-16:00 | Poster Session + Coffee |
16:00-16:50 | Zhiyao Duan (University of Rochester) Frontiers of Speech Synthesis: Controllability, Expressiveness, and Natural Conversations [Youtube] [Slides] |
16:50-17:40 | Chris Donahue (Carnegie Mellon University) The expanding horizons of music AI research [Youtube] [Slides] |
17:40-17:45 | Closing remarks |
17:45-......... | Drinks at Cambridge Brewing Co. |
Directions
The workshop was hosted at Google, in Cambridge MA. Google Cambridge is located at 325 Main St, right above the Kendall/MIT station on the T (i.e., subway) Red Line.
Organizing Committee
- Jonathan Le Roux (MERL)
- John R. Hershey (Google)
Sponsors
SANE remains a free workshop thanks to the generous contributions of the sponsors below.
Talks
Speaker diarization at Google: From modularized systems to LLMs
In this talk, we will introduce the development and evolution of speaker diarization technologies at Google in the past decade, and how they landed as impactful products such as Cloud Speech-to-Text and the Pixel Recorder app. The talk will cover four critical milestones of the speaker diarization technologies at Google: (1) leveraging deep speaker embeddings; (2) leveraging supervised clustering; (3) leveraging sequence transducers; and (4) leveraging large language models. The talk will also discuss how speaker diarization will evolve in the new era of multimodal large language models.
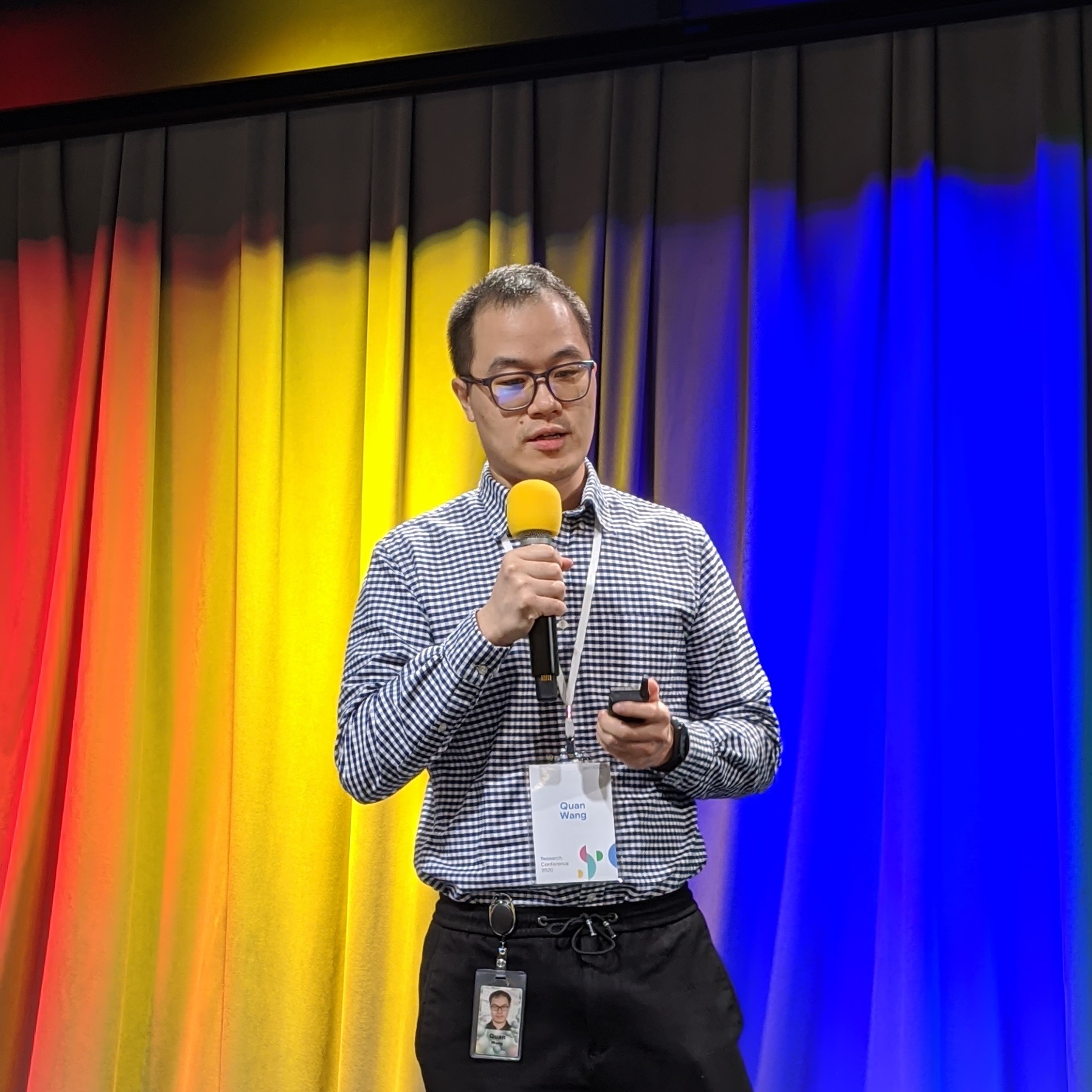
Quan Wang is a Senior Staff Software Engineer at Google, leading the Hotword Modeling team and Speaker, Voice & Language team. Quan is an IEEE Senior Member, and was a former Machine Learning Scientist at Amazon Alexa team. Quan received his B.E. degree from Tsinghua University, and received his Ph.D. degree from Rensselaer Polytechnic Institute. Quan is the author of the award winning Chinese textbook "Voice Identity Techniques: From core algorithms to engineering practice". Quan is also the instructor of the bestselling course "Speaker Recognition" on Udemy and Udemy Business.
Computational models of auditory and language processing in the human brain
MIT
Advances in machine learning have led to powerful models for audio and language, proficient in tasks like speech recognition and fluent language generation. Beyond their immense utility in engineering applications, these models offer valuable tools for neuroscience. In this talk, I will demonstrate how these artificial neural network models can be used to understand how the human brain processes language. The first part of the talk will focus on how audio neural networks can help understand how the different parts of the human auditory cortex supports auditory behavior. The second part will examine the similarities between language processing in large language models and language processing in the human brain—and critically, how we can leverage these models to gain insights into brain processes that have previously been out of reach.
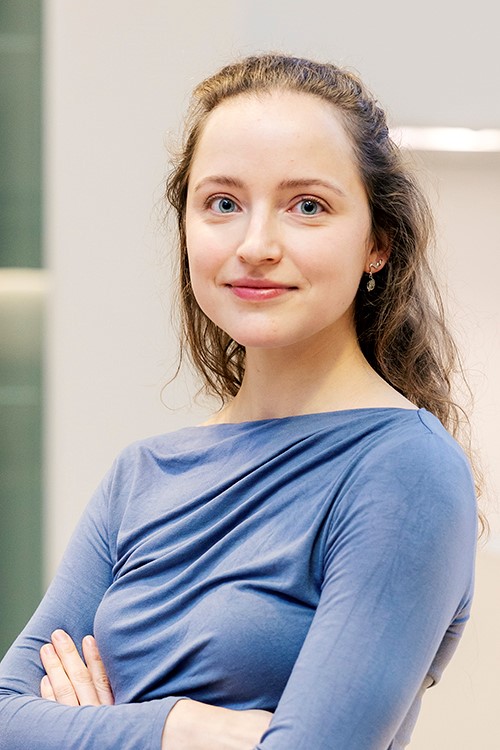
Greta Tuckute is a PhD candidate in the Department of Brain and Cognitive Sciences at MIT. Before joining MIT, she obtained her bachelor’s and master’s degrees at The University of Copenhagen in Denmark. Greta works at the intersection of neuroscience, artificial intelligence, and cognitive science. She is interested in understanding how language is processed in the human brain and how the representations learned by humans compare to those of artificial systems.
Separating the "Chirp" from the "Chat": Self-supervised Visual Grounding of Sound and Language
MIT
We present DenseAV, a novel dual encoder grounding architecture that learns high-resolution, semantically meaningful, and audio-visually aligned features solely through watching videos. We show that DenseAV can discover the ``meaning'' of words and the ``location'' of sounds without explicit localization supervision. Furthermore, it automatically discovers and distinguishes between these two types of associations without supervision. We show that DenseAV's localization abilities arise from a new multi-head feature aggregation operator that directly compares dense image and audio representations for contrastive learning. In contrast, many other systems that learn ``global'' audio and video representations cannot localize words and sound. Finally, we contribute two new datasets to improve the evaluation of AV representations through speech and sound prompted semantic segmentation. On these and other datasets we show DenseAV dramatically outperforms the prior art on speech and sound prompted semantic segmentation. DenseAV outperforms the previous state-of-the-art, ImageBind, on cross-modal retrieval using fewer than half of the parameters.
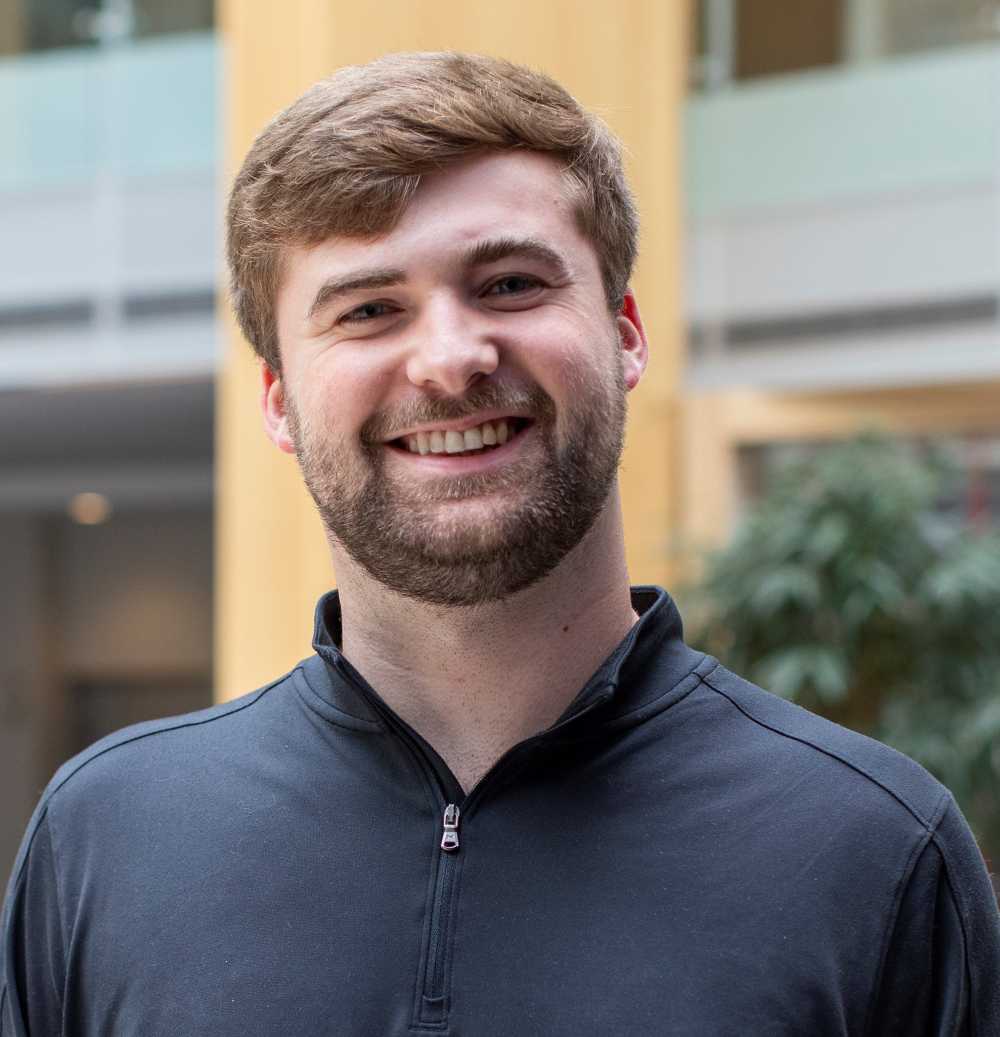
Mark Hamilton is a PhD student in William T Freeman's lab at the MIT Computer Science & Artificial Intelligence Laboratory and a Senior Engineer Manager at Microsoft. Mark’s research aims to discover "structure" in complex systems using unsupervised learning and large foundation models. His prior works include STEGO, a system capable of classifying every pixel of the visual world without any human supervision, and FeatUp, an algorithm for increasing the spatial or temporal resolution of any foundation model by 16-32x. He values working on projects for social, cultural, and environmental good and aims to use ML to empower scientific discovery.
Multilingual Speech Representations
Machine Learning continues to produce models that can scale and solve multilingual, speech and language understanding tasks. Self-supervised learning, first introduced in the field of computer vision, is used to refer to frameworks that learn labels or targets from the unlabeled input signal. In other words, self-supervised learning makes use of proxy supervised learning tasks, such as contrastive learning to identify specific parts of the signal that carry information, thereby helping models to learn robust representations. Recently, self-supervised (pre-training) approaches have gained popularity and become key to the representations learnt by foundational models capable of addressing several tasks in many languages. Multilinguality and code-switching, common in multilingual societies, pose several challenges for speech and language processing. This talk addresses the following questions: Is there a joint latent and robust representation of multiple modalities that can help multilingual speech and language understanding? Are there unsupervised techniques to address languages with scarce data resources? Can this type of cross lingual transfer aid in zero-shot learning with these representations?
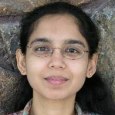
Bhuvana Ramabhadran (IEEE Fellow, 2017, ISCA Fellow 2017) is a Principal Research Scientist leading a team of researchers at Google, focusing on multilingual speech and language understanding. Previously, she was a Distinguished Research Staff Member and Manager in IBM Research AI, at the IBM T. J. Watson Research Center, Yorktown Heights, NY, USA, where she led a team of researchers in the Speech Technologies Group and coordinated activities across IBM’s world wide laboratories in the areas of speech recognition, synthesis, and spoken term detection. She has held several elected posts: Member-At-Large in the IEEE SPS Board of Governors, Chair of the Speech and Language Technical Committee (2014–2016), IEEE SPS conference board (2017-2018), Regional Director-At-Large (2018-2020), and Chair of the IEEE Flanagan Speech & Audio Award Committee. She is currently the Vice President of the International Speech Communication Association (ISCA). She has served on the organizing committees of several ICASSP and Interspeech conferences, most recently as the general chair of SLT 2023. Her research interests include speech recognition and synthesis algorithms, statistical modeling, signal processing, and machine learning.
Frontiers of Speech Synthesis: Controllability, Expressiveness, and Natural Conversations
University of Rochester
Speech synthesis research has made profound progress in the last decade. State-of-the-art text-to-speech and voice conversion systems are able to synthesize speech with high quality that is often indistinguishable from bonafide speech by human ears. However, such systems still lack controllability and expressiveness, and they show limited naturalness in conversational settings. In this talk, I will argue that controllability, expressiveness, and natural conversations are the new frontiers of speech synthesis research. I will present our recent work on these frontiers. Specifically, I will introduce ControlVC, a voice conversion system allowing users to control pitch and speech dynamically; GTR-Voice, our attempt to extend the definition of expressiveness to articulatory phonetics as professional voice actors do; and Parakeet, a system that can synthesize conversational speech with natural pauses, interruptions, and nonverbal events.
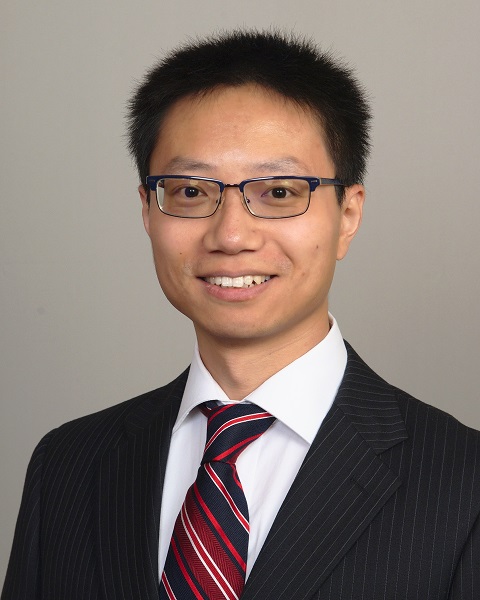
Zhiyao Duan is an associate professor in Electrical and Computer Engineering, Computer Science and Data Science at the University of Rochester. He is also a co-founder of Violy, a music tech company for instrument education. He received his B.S. in Automation and M.S. in Control Science and Engineering from Tsinghua University, China, in 2004 and 2008, respectively, and received his Ph.D. in Computer Science from Northwestern University in 2013. His research interest is in computer audition and its connections with computer vision, natural language processing, and augmented and virtual reality. He received a best paper award at the SMC 2017, a best paper nomination at ISMIR 2017, and a CAREER award from the National Science Foundation (NSF). His research is funded by NSF, NIH, NIJ, New York State Center of Excellence in Data Science, and University of Rochester internal awards on AR/VR, health analytics, and data science. He is the President of the International Society for Music Information Retrieval (ISMIR).
The expanding horizons of music AI research
Carnegie Mellon University
In the span of less than two years, music AI has suddenly leapt out of the research lab and into the real world. This development heralds both profound opportunities and profound risks, and is already redefining relationships between diverse stakeholders including musicians, listeners, the music and tech industries, policymakers, and even researchers. We face a pressing question as researchers: how should our work adapt to meet the rapidly expanding horizons of music AI? In this talk, I will discuss some of my lab’s recent work, which attempts to take a holistic view of music AI research from the core technology (ML), to its presentation to users (HCI), and all the way to broader societal considerations. In particular, I will introduce our work on SingSong and Music ControlNet, which aim to improve the controllability of core generative modeling methods. I will also share our recent work on Hookpad Aria, a “Copilot” for musicians used by thousands of songwriters, and our goals in using Aria to better understand the nature of human-AI music co-creation. Finally, I will present some of our ongoing and future work on in-the-wild evaluation, and highlight many other emerging technical research problems that could bring clarity to some of the broader societal questions surrounding music AI.
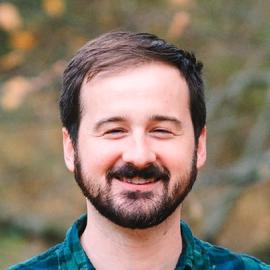
Chris Donahue is an Assistant Professor in the Computer Science Department at CMU, and a part-time research scientist at Google DeepMind working on the Magenta project. His research goal is to develop and responsibly deploy generative AI for music and creativity, thereby unlocking and augmenting human creative potential. In practice, this involves improving machine learning methods for controllable generative modeling of music and audio, and deploying real-world interactive systems that allow anyone to harness generative music AI to accomplish their creative goals through intuitive forms of control. Chris’s research has been featured in live performances by professional musicians like The Flaming Lips, and also empowers hundreds of daily users to convert their favorite music into interactive content through his website Beat Sage. His work has also received coverage from MIT Tech Review, The Verge, Business Insider, and Pitchfork. Before CMU, Chris was a postdoctoral scholar in the CS department at Stanford advised by Percy Liang. Chris holds a PhD from UC San Diego where he was jointly advised by Miller Puckette (music) and Julian McAuley (CS).
Posters
Instructions for posters: most poster boards are 32"x40" (a few are 20"x30", thank you to those who volunteered to use these smaller boards), and can be placed in portrait or landscape orientation.
- 1. HybrA: Adaptive Audio Encodings with Hybrid Filterbanks
Daniel Haider, Felix Perfler, Vincent Lostanlen, Martin Ehler, Peter Balazs
Acoustics Research Institute, LS2N, Uni. ViennaAbstract- The main motivation for this work is the question of whether time-frequency representations as audio encodings for machine learning models should be engineered or learned. In response, we propose HybrA, a hybrid audio encoder composed of a fixed and a learnable filterbank via pair-wise convolution. This offers the possibility to adjust the encoder to the characteristics of the data at hand via the fixed part and allows for further optimization of the final signal representation adaptively via the learned part. While accommodating gradient-based optimization, HybrA maintains desirable properties such as Fourier decay, design of the center frequency progression, and numerical stability throughout training. This makes it a powerful and versatile tool for encoding (and decoding) audio signals, readily integrated into any deep learning pipeline. We present two aspects of HybrA, one related to theoretical properties and a practical application. The theoretical part considers the setting where the fixed filters form a tight frame and the learned filters are initialized as i.i.d. normal random variables. We show that the resulting HybrA is more stable than a purely random filterbank, i.e., a convolutional layer with 1-D filters at initialization, and has decreased mutual incoherence. In the applied part, we present a specific application of using a HybrA as the encoder and decoder in a low-complexity masking model for speech enhancement. The fixed filters are chosen to form a tight frame specifically designed for encoding speech signals, and the learned filters are optimized to minimize a spectral condition in the coefficient domain. Equipped with this encoder-decoder pair, the model is capable of significantly improving the perceptual evaluation of speech quality (PESQ) compared to only using the fixed filterbank or a fully learned convolutional encoder.
- 2. Optimizing Byte-level Representation for End-to-end ASR
Roger Hsiao, Liuhui Deng, Erik McDermott, Ruchir Travadi, Xiaodan Zhuang
AppleAbstract- In this paper, we propose an algorithm to optimize a byte-level representation for end-to-end (E2E) automatic speech recognition (ASR). Byte-level representation is often used by large scale multilingual ASR systems when the character set of the supported languages is large. The compactness and universality of byte-level representation allow the ASR models to use smaller output and therefore, provides more flexibility. UTF-8 is the most commonly used byte-level representation and has been successfully applied to ASR. However, it is not designed for ASR or any machine learning tasks. By using auto-encoder and vector quantization, we show that we can optimize a byte-level representation for ASR and achieve better accuracy. Our proposed framework can incorporate information from different modalities and provide an error correction mechanism. In an English/Mandarin dictation task, we show that the bilingual ASR model built with this approach can outperform UTF-8 representation by 5% relative in error rate.
- 3. Audio-Visual Target Speaker Speech Enhancement
Aleksandra Ma, Sile Yin, Shuo Zhang
Bose CorporationAbstract- In this work, we present a novel approach to target speech enhancement, focusing on isolating the speech of an on-screen speaker while rejecting off-screen interfering speech using lip movement information from camera input. Our approach leverages a mask-based fusion model that integrates both visual and audio cues to enhance the target speaker's voice. To achieve this, we employed a pretrained visual encoder trained on audio-visual speech recognition tasks and combined it with a CNN-based audio encoder. The visual encoder extracts lip movement information, which aids in distinguishing the target speech from interfering sources. Two variations of the audio encoder were trained: a deep encoder network and a shallow encoder network, allowing for a comparative analysis of model complexity and performance, as well as the strength of visual cues from lip movement. The models were evaluated using both the VoxCeleb2 test set and real-world video scenarios. We conducted a thorough analysis of performance across different scenarios, utilizing both objective metrics (such as Signal-to-Distortion Ratio and Perceptual Evaluation of Speech Quality) and qualitative evaluations based on demo videos.
- 4. Introducing BOSSA: A Biologically Oriented Sound Segregation Algorithm
Alex Boyd, Virginia Best, Kamal Sen
Boston UniversityAbstract- Listening in acoustically cluttered scenarios remains a difficult task for both humans and machines. For listeners with hearing loss, this difficulty is often extreme and can seriously impede communication in noisy everyday situations. It has long been recognized that spatial filtering can alleviate these difficulties, and many hearing devices now incorporate directional microphones or beamforming technology. Here we present a biologically inspired algorithm designed to isolate sounds based on spatial location, and consider its potential utility in hearing devices. The algorithm is based on a hierarchical network model of the auditory system, in which binaural sound inputs drive populations of neurons tuned to specific spatial locations and frequencies, and the spiking responses of neurons in the output layer are then reconstructed into audible waveforms. The algorithm has sharp spatial tuning, can be flexibly configured, and is well-suited to low-power real-time applications. We previously evaluated the algorithm in normal hearing listeners, by measuring speech intelligibility in a challenging mixture of five competing talkers, and found benefits similar to those provided by a multi-microphone beamforming array. In our current work we are extending this evaluation to listeners with sensorineural hearing loss. We will present those results and discuss the advantages of biologically inspired processing for hearing devices more broadly.
- 5. Meter Detection: Computing Audio Features Using Mamba-Based Models
David Liu, Brian Kulis
Boston UniversityAbstract- Meter detection is a critical task in music analysis, traditionally handled using convolutional neural networks (CNNs) and classical methods. However, these models struggle to capture long-range dependencies within musical pieces. This work explores the application of state-space models (SSMs), particularly the Mamba block architecture, to classify the meter of 30-second audio clips into one of four classes (3, 4, 5, and 7). By utilizing Harmonic-Percussive Source Separation, our proposed method can utilize the percussive element of an audio clip, which contains more information on rhythmic patterns. The results demonstrate an approximate 3.5% improvement over existing methods, highlighting the potential of SSMs for time-series tasks in music. Future work aims to extend this model to other musical features and tasks.
- 6. Self-supervised Speech Models Rediscover Phonemes
Kwanghee Choi, Eunjung Yeo, Kalvin Chang, William Chen, Shinji Watanabe, David Mortensen
CMUAbstract- Self-supervised speech models (S3Ms) have been shown to encode phonetic and phonemic information, yet how the information is structured and whether S3Ms perceive phonemes similarly to humans remains unclear. In this study, we first examine the clusteredness of phonemes within S3M representations, drawing from the concept of categorical perception in auditory phonetics. We then investigate whether phonological features are embedded within S3M representations by analyzing similarity measures between phoneme pairs. We show that phonemes differing in phonological features exhibit lower similarity than those sharing similar features, implying the existence of a metric space correlated with articulatory feature edit distance as the measure. Next, we assess how S3Ms encode phonemes, demonstrating that phonemes are represented in a decompositional manner. Specifically, we test whether each phoneme can be represented as a sum of vectors corresponding to its individual phonological features. Finally, we evaluate the models' performance in zero-shot phoneme classification tasks, focusing on unseen phonemes to determine whether this decompositional structure can be leveraged. This study aims to provide insights into how phonetic and phonological information is encoded in S3Ms and whether these models can generalize to previously unseen phonemes.
- 7. Leveraging Allophony in Self-Supervised Speech Representations for Out-of-Distribution Detection in Atypical Pronunciation Assessment
Kwanghee Choi, Eunjung Yeo, Kalvin Chang, Shinji Watanabe, David Mortensen
CMUAbstract- Allophony refers to the different phonetic realizations of a phoneme based on its phonetic environment, each with distinct acoustic characteristics. We hypothesize that accurately modeling allophonic variation, grounded in well-established phonological principles, enhances phoneme distribution modeling. This is particularly crucial for phoneme-level pronunciation assessment, a task often conceptualized as out-of-distribution (OOD) detection. However, previous studies frequently treated phonemes as single clusters, overlooking allophonic variation and limiting their ability to fully capture this important speech characteristic. In this work, we demonstrate that self-supervised speech models (S3Ms) are more effective at capturing allophonic variation compared to traditional features, such as MFCCs. To further leverage the strengths of S3Ms, we introduce MixGoP, a mixture distribution-based approach that models phoneme distributions as multiple clusters, allowing for a more accurate representation of allophonic variation. MixGoP achieves state-of-the-art performance across four out of five datasets, including three dysarthric and two non-native speech datasets. This work highlights the critical importance of modeling allophony within self-supervised speech representations for OOD detection, with significant applications for assessing atypical speech pronunciation.
- 8. Towards Robust Speech Representation Learning for Thousands of Languages
William Chen, Wangyou Zhang, Yifan Peng, Xinjian Li, Jinchuan Tian, Jiatong Shi, Xuankai Chang, Soumi Maiti, Karen Livescu, Shinji Watanabe
CMUAbstract- Self-supervised learning (SSL) has helped extend speech technologies to more languages by reducing the need for labeled data. However, models are still far from supporting the world's 7000+ languages. We propose XEUS, a Cross-lingual Encoder for Universal Speech, trained on over 1 million hours of data across 4057 languages, extending the language coverage of SSL models 4-fold. We combine 1 million hours of speech from existing publicly accessible corpora with a newly created corpus of 7400+ hours from 4057 languages, which will be publicly released. To handle the diverse conditions of multilingual speech data, we augment the typical SSL masked prediction approach with a novel dereverberation objective, increasing robustness. We evaluate XEUS on several benchmarks, and show that it consistently outperforms or achieves comparable results to state-of-the-art (SOTA) SSL models across a variety of tasks. XEUS sets a new SOTA on the ML-SUPERB benchmark: it outperforms MMS 1B and w2v-BERT 2.0 v2 by 0.8% and 4.4% respectively, despite having less parameters or pre-training data. We will release all checkpoints and model code.
- 9. Improving Multilingual ASR in the Wild using Simple N-best Re-ranking
Brian Yan, Vineel Pratap, Shinji Watanabe, Michael Auli
CMU, MetaAbstract- Multilingual Automatic Speech Recognition (ASR) models are typically evaluated in a setting where the ground-truth language of the speech utterance is known, however, this is often not the case for most practical settings. Automatic Spoken Language Identification (SLID) models are not perfect and misclassifications have a substantial impact on the final ASR accuracy. In this paper, we present a simple and effective N-best re-ranking approach to improve multilingual ASR accuracy for several prominent acoustic models by employing external features such as language models and text-based language identification models. Our results on FLEURS using the MMS and Whisper models show spoken language identification accuracy improvements of 8.7% and 6.1%, respectively and word error rates which are 3.3% and 2.0% lower on these benchmarks
- 10. Exploring Diverse Approaches to Improve Automatic Beat Detection
Ziyun Liu, Tung-Cheng Su, Purusottam Samal
CMUAbstract- The automatic beat detection task encompasses both beat and downbeat tracking, crucial for various Music Information Retrieval (MIR) tasks, yet facing challenges in overall accuracy. To address this, we tested two potential methods to improve beat detection performances. First, we introduced osu!7K, a new dataset obtained by converting beatmaps created by users in the open-source rhythm game osu! into conventional training data, which could potentially address the issue of limited data. Preliminary experiments showed that the influence of the new dataset varied for different test datasets. Then, we tried incorporating the middle layer of Jukebox in the feature extraction process to test the potential of using representations learned by large-scale music generative models for beat detection. In preliminary experiments, we achieved SOTA on the Ballroom dataset, and the effect still varied across datasets. These experiments also inspired us to further analyze the existing datasets and current models. Drawing inspiration from how the osu!7K dataset was constructed, we propose a potential way to improve beat detection performances, especially for datasets containing highly expressive music such as SMC, by utilizing the original beatmap data from osu!.
- 11. Towards Perception-Aware Models for Music: A Sample Study on Chord Reduction
Ziyun Liu, Edward Wersocki, Leon Chen
CMU, QMULAbstract- We propose a novel approach to incorporate human perception in music-related tasks, using chord reduction as a case study. In music-related tasks such as automatic music transcription, language-inspired evaluation methods and traditional audio loss functions fail at capturing human cognition of music. While music theory models can capture tonal relationships and chord transitions, they often overlook the perceptual impacts of chord reduction, chord inversion and pitch height. By integrating frequency analysis with human auditory perception theory, we aim to provide a more human-centered evaluation for chord reduction that can also be used in evaluating non-Western music and effects of hearing loss. This approach considers how the relationships between simultaneously played notes influences our perception of the chord in a way similar to perceptual audio coding, and it could also offer a simple yet effective method for score complexity adjustment, addressing current limitations in existing models and dataset availability. We hope that this study could inspire researchers to consider novel loss functions as ways to incorporate human perception in audio-related tasks.
- 12. Phone Recognition with Linguistically Interpretable Hierarchical CTC
Kalvin Chang, Shih-Heng Wang, Kwanghee Choi, Eunjung Yeo, Aaricia Herygers, Chin-Jou Li, Farhan Samir, Jian Zhu, Shinji Watanabe, David R Mortensen
CMU, alphaspeech, UBCAbstract- Phone recognition is an important task for atypical speech assessment, sociolinguistic coding, endangered language documentation, and pronunciation training. However, existing phone recognizers display accuracies so low that they are not practical for most applications, especially in lower resource scenarios. Recent work leverages auxiliary CTC losses to predict the articulatory features of phonemes (Glocker et al 2023). Unlike prior work, we leverage a hierarchical CTC approach (Higuchi et al 2023) to predict natural classes, phonemes, allophones, and articulatory features in an interpretable linguistic hierarchy. At the lowest level, we will predict articulatory features. At the layer above, we will predict natural classes, such as stops and fricatives (List 2012). Next, we will predict the phoneme, which reflects a broad transcription. When we have allophone-level transcriptions, we will predict the allophone. When we are not confident in the G2P output, we avoid predicting the phoneme and instead predict the broad sound classes, enabling us to leverage noisy transcriptions. Our experiments leverage IPAPack, a 1000-hour phonemically transcribed dataset covering 115 languages. Our work lays the foundation for improved accuracy of phone recognition.
- 13. Historical Linguistics-Informed Speech In-Context Learning for Low-Resource Language Varieties
Kalvin Chang*, Shih-Heng Wang*, Ming-Hao Hsu*, Soh-Eun Shim*, Alex Cheng, Eunjung Yeo, Kwanghee Choi, Hung-yi Lee, Barbara Plank, Jonathan Amith, Shinji Watanabe, David R Mortensen
CMU, National Taiwan University, LMU, Gettysburg CollegeAbstract- Phonetic variation across varieties of the same language is often overlooked in automatic speech recognition, leading to biases in performance against non-standard varieties. Self-supervised pre-training has reduced the amount of labeled low-resource data needed, but recent research shows that S3Ms cannot generalize to unseen pronunciation variants. We show that we can leverage in-context learning to prompt Whisper and OWSM to generalize to unseen pronunciation variants in a few shot fashion. Unlike prior work, we prompt the model with demonstrations of sound correspondences (patterns of sound change) across varieties, which follow the Neogrammarian hypothesis: sound change is systematic, affecting all instances of a sound in specific contexts. We evaluate our approach on non-standard varieties of Mandarin, Yue, and Italian, whose standard varieties are all in Whisper’s pre-training data. In contrast to adaptation methods such as LoRA, our approach does not require fine-tuning, which is impractical for low-resource varieties. By applying historical linguistic insights, our few-shot method will reduce the need for labeled data in low-resource ASR and mitigate biases in ASR performance against non-standard varieties.
- 14. ESPnet-Codec: Comprehensive Training and Evaluation of Neural Codecs for Audio, Music, and Speech
Jiatong Shi, Jinchuan Tian, Yihan Wu, Jee-weon Jung, Jia Qi Yip, Yoshiki Masuyama, William Chen, Yuning Wu, Yuxun Tang, Massa Baali, Dareen Alharthi, Dong Zhang, Ruifan Deng, Tejes Srivastava, Haibin Wu, Alexander H. Liu, Bhiksha Raj, Qin Jin, Ruihua Song, Shinji Watanabe
CMU, Renmin University of China, Nanyang Technological University, Tokyo Metropolitan University, Fudan University, University of Chicago, National Taiwan University, MITAbstract- Neural codecs have become crucial to recent speech and audio generation research. In addition to signal compression capabilities, discrete codecs have also been found to enhance downstream training efficiency and compatibility with autoregressive language models. However, as extensive downstream applications are investigated, challenges have arisen in ensuring fair comparisons across diverse applications. To address these issues, we present a new open-source platform ESPnet-Codec, which is built on ESPnet and focuses on neural codec training and evaluation. ESPnet-Codec offers various recipes in audio, music, and speech for training and evaluation using several widely adopted codec models. Together with ESPnet-Codec, we present VERSA, a standalone evaluation toolkit, which provides a comprehensive evaluation of codec performance over 20 audio evaluation metrics. Notably, we demonstrate that ESPnet-Codec can be integrated into six ESPnet tasks, supporting diverse applications.
- 15. UniverSLU: Universal Spoken Language Understanding for Diverse Tasks with Natural Language Instructions
Siddhant Arora, Hayato Futami, Jee-weon Jung, Yifan Peng, Roshan Sharma, Yosuke Kashiwagi, Emiru Tsunoo, Karen Livescu, Shinji Watanabe
CMU, Sony, TTI-CAbstract- Recent studies leverage large language models with multi-tasking capabilities, using natural language prompts to guide the model’s behavior and surpassing performance of task-specific models. Motivated by this, we ask: can we build a single model that jointly performs various spoken language understanding (SLU) tasks? We start by adapting a pre-trained automatic speech recognition model to additional tasks using single-token task specifiers. We enhance this approach through instruction tuning, i.e., finetuning by describing the task using natural language instructions followed by the list of label options. Our approach can generalize to new task descriptions for the seen tasks during inference, thereby enhancing its user-friendliness. We demonstrate the efficacy of our single multi-task learning model “UniverSLU” for 12 speech classification and sequence generation task types spanning 17 datasets and 9 languages. On most tasks, UniverSLU achieves competitive performance and often even surpasses task-specific models. Additionally, we assess the zero-shot capabilities, finding that the model generalizes to new datasets and languages for seen task types.
- 16. Preference Alignment Improves Language Model-Based TTS
Jinchuan Tian, Chunlei Zhang, Jiatong Shi, Hao Zhang, Jianwei Yu, Shinji Watanabe, Dong Yu
CMU, Tencent AI LABAbstract- Recent advancements in text-to-speech (TTS) have shown that language model (LM)-based systems offer competitive performance to their counterparts. Further optimization can be achieved through preference alignment algorithms, which adjust LMs to align with the preferences of reward models, enhancing the desirability of the generated content. This study presents a thorough empirical evaluation of how preference alignment algorithms, particularly Direct Preference Optimization (DPO), enhance LM-based TTS. With a 1.15B parameter LM-based TTS model, we demonstrate that preference alignment consistently improves intelligibility, speaker similarity, and proxy subjective evaluation scores, with the latter two metrics surpassing even human speech in certain evaluations. We also show preference alignment is applicable to low-resource scenarios and effectively generalized to out-of-domain applications.
- 17. Human-like feature attention emerges in task-optimized models of the cocktail party problem
Ian Griffith, R. Preston Hess, Josh McDermott
Harvard University, MITAbstract- Background: Attention enables communication in settings with multiple talkers, allowing us to select sources of interest based on their features. Decades of research have left two gaps in our understanding of feature-based attention. First, humans succeed at attentional selection in some conditions but fail in others, for reasons that remain unclear. Second, neurophysiology experiments implicate multiplicative gains in selective attention, but it remains unclear whether such gains are sufficient to account for real-world attention-driven behavior. To address these gaps, we optimized an artificial neural network with stimulus-computable feature-based gains for the task of recognizing a cued talker’s speech, using binaural audio input (a “cocktail party” setting).
Methods: We optimized a deep neural network to report words spoken by a cued talker in a multi-source mixture. Audio was spatialized within simulated reverberant rooms using human head-related transfer functions. Attentional gain was implemented as learnable logistic functions operating on the time-averaged model activations of a cued talker. Gains could be high for features of the cue, and low for uncued features, as determined by parameters optimized to maximize correct recognition. Task performance was measured by word recognition accuracy as a function of target-distractor ratio (SNR) and target-distractor spatial proximity.
Results: The model successfully learned to use both spatial and vocal timbre cues to solve the word recognition task. In the presence of competing talkers the model correctly reported the words of the cued talker and ignored the distractor talker(s). Similar to humans, the model showed higher accuracy with single-talker distractors than with multi-talker distractors. The model’s internal representations revealed that attentional selection occurred only at later model stages.
Conclusions: We provide a framework to quantitatively model feature-based auditory attention by optimizing a deep neural network to perform an attentional word recognition task. The model provides hypotheses for how attention might be expected to modulate neural responses at different stages of the auditory system, and can help understand the conditions in which attentional selection is intrinsically difficult.
- Background: Attention enables communication in settings with multiple talkers, allowing us to select sources of interest based on their features. Decades of research have left two gaps in our understanding of feature-based attention. First, humans succeed at attentional selection in some conditions but fail in others, for reasons that remain unclear. Second, neurophysiology experiments implicate multiplicative gains in selective attention, but it remains unclear whether such gains are sufficient to account for real-world attention-driven behavior. To address these gaps, we optimized an artificial neural network with stimulus-computable feature-based gains for the task of recognizing a cued talker’s speech, using binaural audio input (a “cocktail party” setting).
- 18. Waveform and spectrogram analysis to determine acoustically distinct contact calls in two subspecies of a New World warbler, Setophaga coronata
Shonit N. Sharma, Shay N. Sharma, Matthew A. Young, Thomas P. Hahn
Harvard University, MIT, Stanford, Cornell, UC DavisAbstract- The myrtle and Audubon’s warblers are two subspecies of the yellow-rumped warbler (Setophaga coronata) that share a geographical hybrid zone in North America. Studies to date differentiate these subspecies based on genetic and morphological characters. Here we distinguish them based on features of their “chip” calls. We screened crowdsourced recordings of wildlife sounds, in total, 203 recordings of myrtle and 134 recordings of Audubon’s warbler, and selected 10 high-quality recordings from each subspecies for analysis. We then performed waveform and spectrogram analyses to identify quantitative features of selected exemplar calls using a popular bioacousitcs software (Raven Lite). Short, “chip” calls of the two subspecies were distinct in starting frequency, frequency range, duration, and shape. Specifically, Audubon’s calls began at an average frequency of 3565 Hz, higher than the myrtle call at 3313 Hz. The total duration was longer for Audubon’s calls (0.0272 seconds) than for myrtle (0.0180 seconds). At 0.0091 seconds into the call, myrtle warblers attained an average peak frequency of 6278 Hz and then abruptly dropped in pitch, while Audubon’s frequency increased throughout the call to a peak of 5870 Hz at the end. The frequency range was thus greater for myrtle (3313-6278 Hz) than for Audonbon’s warblers (3565-5870 Hz). Although many bird watchers are aware that these subspecies are separable by ear based on “chip” call, there has been no previous systematic examination of the nature of this variation. This audio analysis provides a basis for a more careful examination of geographic vocal variation across the range of each taxon, and our approach should be considered when looking for hybrid individuals, which may produce intergrade calls. Applying similar waveform and spectrogram analyses to simple, innate calls of other species with multiple subspecies may yield valuable insights and potentially new taxonomic classifications.
- 19. EzAudio: Enhancing Text-to-Audio Generation with Efficient Diffusion Transformer
Jiarui Hai, Yong Xu, Hao Zhang, ChenXing Li, Helin Wang, Mounya Elhilali, Dong Yu
Johns Hopkins Unversity, Tencent AI LabAbstract- Latent diffusion models have shown promising results in text-to-audio (T2A) generation tasks, yet previous models have encountered difficulties in generation quality, computational cost, diffusion sampling, and data preparation. In this paper, we introduce EzAudio, a transformer-based T2A diffusion model, to handle these challenges. Our approach includes several key innovations: (1) We build the T2A model on the latent space of a 1D waveform Variational Autoencoder (VAE), avoiding the complexities of handling 2D spectrogram representations and using an additional neural vocoder. (2) We design an optimized diffusion transformer architecture specifically tailored for audio latent representations and diffusion modeling, which enhances convergence speed, training stability, and memory usage, making the training process easier and more efficient. (3) To tackle data scarcity, we adopt a data-efficient training strategy that leverages unlabeled data for learning acoustic dependencies, audio caption data annotated by audio-language models for text-to-audio alignment learning, and human-labeled data for fine-tuning. (4) We introduce a classifier-free guidance (CFG) rescaling method that simplifies EzAudio by achieving strong prompt alignment while preserving great audio quality when using larger CFG scores, eliminating the need to struggle with finding the optimal CFG score to balance this trade-off. EzAudio surpasses existing open-source models in both objective metrics and subjective evaluations, delivering realistic listening experiences while maintaining a streamlined model structure, low training costs, and an easy-to-follow training pipeline. Code, data, and pre-trained models are released at: https://haidog-yaqub.github.io/EzAudio-Page/.
- 20. SSR-Speech: Towards Stable, Safe and Robust Zero-shot Text-based Speech Editing and Synthesis
Helin Wang, Meng Yu, Jiarui Hai, Chen Chen, Yuchen Hu, Rilin Chen, Najim Dehak, Dong Yu
Johns Hopkins Unversity, Tencent AI Lab, Nanyang Technological UniversityAbstract- SSR-Speech is a neural codec autoregressive model designed for stable, safe, and robust zero-shot text-based speech editing and text-to-speech synthesis. SSR-Speech is built on a Transformer decoder and incorporates classifier-free guidance to enhance the stability of the generation process. A watermark Encodec is proposed to embed frame-level watermarks into the edited regions of the speech so that which parts were edited can be detected. In addition, the waveform reconstruction leverages the original unedited speech segments, providing superior recovery compared to the Encodec model. SSR-Speech achieves the state-of-the-art performance in the RealEdit speech editing task and the LibriTTS text-to-speech task, surpassing previous methods. Furthermore, SSR-Speech excels in multispan speech editing and also demonstrates remarkable robustness to background sounds.
- 21. Human Action Understanding-based Robot Planning using Multimodal LLM
Motonari Kambara, Chiori Hori, Komei Sugiura, Kei Ota, Devesh K. Jha, Sameer Khurana, Siddarth Jain, Radu Corcodel, Diego Romeres, and Jonathan Le Roux
MERL, Keio UniversityAbstract- In future smart homes, robots are expected to handle everyday tasks such as cooking, replacing human involvement. Acquiring such skills autonomously for robots is highly challenging. Consequently, existing methods address this issue by collecting data by controlling real robots and training models through supervised learning. However, data collection for long-horizon tasks could be very painful. To solve this challenge, this work focuses on the task of generating action sequences for a robot arm from human videos demonstrating cooking tasks. The quality of generated action sequences by existing methods for this task is often inadequate. This is partly because existing methods do not effectively process each of the input modalities. To address this issue, we propose AVBLIP, a multimodal LLM model for the generation of robot action sequences. Our main contribution is the introduction of a multimodal encoder that allows multiple modalities of video, audio, speech, and text as inputs. This allows the generation of the next action to take into account both the speech information by humans and the audio information generated by the environment. As a result, the proposed method outperforms the baseline method in all standard evaluation metrics.
- 22. Spatially-Aware Losses for Enhanced Neural Acoustic Fields
Christopher A. Ick, Gordon Wichern, Yoshiki Masuyama, François Germain, Jonathan Le Roux
MERL, NYUAbstract- For immersive audio experiences, it is essential that sound propagation is accurately modeled from a source to a listener through space. For human listeners, binaural audio characterizes the acoustic environment, as well as the spatial aspects of an acoustic scene. Recent advancements in neural acoustic fields have demonstrated spatially continuous models that are able to accurately reconstruct binaural impulse responses for a given source/listener pair. Despite this, these approaches have not explicitly examined or evaluated the quality of these reconstructions in terms of the inter-aural cues that define spatialization for human listeners. In this work, we propose extending neural acoustic field-based methods with spatially-aware metrics for training and evaluation to better capture spatial acoustic cues. We develop a dataset based on the existing SoundSpaces dataset to better model these features, and we demonstrate performance improvements by utilizing spatially-aware losses.
- 23. MASV: Speaker Verification with Global and Local Context Mamba
Yang Liu, Li Wan, Yiteng Huang, Ming Sun, Yangyang Shi, Florian Metze
MetaAbstract- Deep learning models like Convolutional Neural Networks and transformers have shown impressive capabilities in speech verification, gaining considerable attention in the research community. However, CNN-based approaches struggle with modeling long-sequence audio effectively, resulting in suboptimal verification performance. On the other hand, transformer-based methods are often hindered by high computational demands, limiting their practicality. This paper presents the MASV model, a novel architecture that integrates the Mamba module into the ECAPA-TDNN framework. By introducing the Local Context Bidirectional Mamba and Tri-Mamba block, the model effectively captures both global and local context within audio sequences. Experimental results demonstrate that the MASV model substantially enhances verification performance, surpassing existing models in both accuracy and efficiency.
- 24. Data Efficient Reflow for Few Step Audio Generation
Lemeng Wu, Zhaoheng Ni, Bowen Shi , Gael Le Lan, Anurag Kumar, Varun Nagaraja, Xinhao Mei , Yunyang Xiong, Bilge Soran, Raghuraman Krishnamoorthi , Wei-Ning Hsu, Yangyang Shi , Vikas Chandra
Meta, Meta FAIRAbstract- Flow matching has been successfully applied onto generative models, particularly in producing high-quality images and audio. However, the iterative sampling required for the ODE solver in flow matching-based approaches can be time-consuming. Reflow finetune, a technique derived from Rectified flow, offers a promising solution by transforming the ODE trajectory into a straight one, thereby reducing the number of sampling steps. In this paper, we focus on developing data-efficient flow-based approaches for text-to-audio generation. We found that directly applying reflow to the pre-trained flow matching-based audio generation models is typically computationally expensive. It requires over 50,000 training iterations and five times the amount of training data to achieve satisfactory results. To address this issue, we introduce a novel data-efficient reflow (DEreflow) method. This method modifies the reflow data pairs and trajectory to align with the flow matching distribution. As a result of this alignment, our approach requires significantly fewer steps (8,000 compared to 50,000) and data pairs (0.5 times the scale of training data compared to 5 times). Results show that the proposed DEreflow consistently outperforms the original reflow method on the text-to-audio generation task.
- 25. Whisper-Flamingo: Integrating Visual Features into Whisper for Audio-Visual Speech Recognition and Translation
Andrew Rouditchenko, Yuan Gong, Samuel Thomas, Leonid Karlinsky, Hilde Kuehne, Rogerio Feris, James Glass
MITAbstract- Audio-Visual Speech Recognition (AVSR) uses lip-based video to improve performance in noise. Since videos are harder to obtain than audio, the video training data of AVSR models is usually limited to a few thousand hours. In contrast, speech models such as Whisper are trained with hundreds of thousands of hours of data, and thus learn a better speech-to-text decoder. The huge training data difference motivates us to adapt Whisper to handle video inputs. Inspired by Flamingo which injects visual features into language models, we propose Whisper-Flamingo which integrates visual features into the Whisper speech recognition and translation model with gated cross attention. Our audio-visual Whisper-Flamingo outperforms audio-only Whisper on English speech recognition and En-X translation for 6 languages in noisy conditions. Moreover, Whisper-Flamingo is a versatile model and conducts all of these tasks using one set of parameters, while prior methods are trained separately on each language.
- 26. Modeling Continuous Speech Perception Using Artificial Neural Networks
Gasser Elbanna, Josh H. McDermott
MITAbstract- Humans possess a remarkable ability to transform pressure waveforms entering the ear into meaningful linguistic representations. Despite decades of research in auditory perception, our understanding of speech perception remains limited. A fundamental computational challenge for speech perception is the lack of invariance in the speech signal. This challenge has driven the development of speech perception models; however, we still lack biologically plausible and fully stimulus-computable models of speech perception that replicate human levels of performance.
We developed a candidate model of human continuous speech perception by training an artificial neural network to generate sequences of American English phonemes from acoustic signals processed through a simulated cochlea. The model architecture includes six 2-dimensional convolutional layers for downsampling, followed by six bi-directional LSTM layers to capture temporal dependencies. The LSTM hidden states are mapped into phoneme space via a linear layer, and the model is trained using Connectionist Temporal Classification (CTC) loss.
To address limited phoneme-labeled data, we used a pseudo-supervised training approach, employing a Grapheme-to-Phoneme model to transcribe phonemes from large-scale speech corpora, resulting in approximately 6 million transcribed utterances for training. For human-model comparisons, we conducted a behavioral experiment in which 100 participants transcribed 5000 nonwords, allowing direct comparison under identical conditions.
Compared to existing automatic speech recognition systems, the model demonstrated competitive performance on unseen data and various transcription methods. In non-word recognition tasks, humans performed slightly better with an average phoneme error rate (PER) of 29%, compared to 33% for the model. However, at the phoneme level, the model exhibited a similar pattern of phoneme confusions as humans, both for consonants (r=0.91) and for vowels (r=0.87). The recognizability of individual phonemes was also highly correlated between humans and the model (r=0.93), highlighting the model’s alignment with human perceptual patterns.
These findings collectively suggest that human-like speech perception emerges by optimizing for phoneme recognition from cochlear representations. This work lays the groundwork for systematic comparisons between human and model perception, including analyses of confusion patterns, categorical perception, auditory illusions, and context effects.
- Humans possess a remarkable ability to transform pressure waveforms entering the ear into meaningful linguistic representations. Despite decades of research in auditory perception, our understanding of speech perception remains limited. A fundamental computational challenge for speech perception is the lack of invariance in the speech signal. This challenge has driven the development of speech perception models; however, we still lack biologically plausible and fully stimulus-computable models of speech perception that replicate human levels of performance.
- 27. Creative Text-to-Audio Generation via Synthesizer Programming
Manuel Cherep, Nikhil Singh, Jessica Shand
MITAbstract- Neural audio synthesis methods now allow specifying ideas in natural language. However, these methods produce results that cannot be easily tweaked, as they are based on large latent spaces and up to billions of uninterpretable parameters. We propose a text-to-audio generation method that leverages a virtual modular sound synthesizer with only 78 parameters. Synthesizers have long been used by skilled sound designers for media like music and film due to their flexibility and intuitive controls. Our method, CTAG, iteratively updates a synthesizer's parameters to produce high-quality audio renderings of text prompts that can be easily inspected and tweaked. Sounds produced this way are also more abstract, capturing essential conceptual features over fine-grained acoustic details, akin to how simple sketches can vividly convey visual concepts. Our results show how CTAG produces sounds that are distinctive, perceived as artistic, and yet similarly identifiable to recent neural audio synthesis models, positioning it as a valuable and complementary tool.
- 28. Contrastive Learning from Synthetic Audio Doppelgängers
Manuel Cherep, Nikhil Singh
MITAbstract- Learning robust audio representations currently demands extensive datasets of real-world sound recordings. By applying artificial transformations to these recordings, models can learn to recognize similarities despite subtle variations through techniques like contrastive learning. However, these transformations are only approximations of the true diversity found in real-world sounds, which are generated by complex interactions of physical processes, from vocal cord vibrations to the resonance of musical instruments. We propose a solution to both the data scale and transformation limitations, leveraging synthetic audio. By randomly perturbing the parameters of a sound synthesizer, we generate audio doppelgängers—synthetic positive pairs with causally manipulated variations in timbre, pitch, and temporal envelopes. These variations, difficult to achieve through transformations of existing audio, provide a rich source of contrastive information. Despite the shift to randomly generated synthetic data, our method produces strong representations, competitive with real data on standard audio classification benchmarks. Notably, our approach is lightweight, requires no data storage, and has only a single hyperparameter, which we extensively analyze. We offer this method as a complement to existing strategies for contrastive learning in audio, using synthesized sounds to reduce the data burden on practitioners.
- 29. Cross-lingual conversational speech summarization with Large Language Models
Sammi Sung, William Hartmann
RTX BBN TechnologiesAbstract- Cross-lingual conversational speech summarization is a challenging problem. We build upon the existing Fisher and Callhome Spanish-English Speech Translation corpus by supplementing the translations with summaries. The summaries are generated using GPT-4 from the reference translations and are treated as ground truth. The task is to generate similar summaries in the presence of transcription and translation errors. We build a baseline cascade-based system using open-source speech recognition (Whisper) and machine translation (NLLB) models. We test a range of LLMs for summarization and analyze the impact of transcription and translation errors. Adapting the Mistral-7B model for this task performs significantly better than off-the-shelf models and matches the performance of GPT-4.
- 30. Jordan and the jam_bot: Insights from a Human-AI co-created concert
Lancelot Blanchard, Perry Naseck, Madhav Lavakare, Eran Egozy, Joe Paradiso
MIT Media Lab, Yale University, MITAbstract- On September 21, 2023, as part of a collaboration between the MIT Media Lab and GRAMMY-winning keyboardist and technologist Jordan Rudess, we organized a novel human-AI co-created concert titled Jordan and the jam_bot. In this performance, Rudess improvised live on stage across various genres, accompanied by a diverse set of custom-trained Music Transformer models specifically designed to improvise alongside him. To enhance audience understanding of the interplay between human and AI-generated music, we developed a large kinetic sculpture that served as a medium for visualization, controlled by cross-modal transformers converting symbolic music input into visual outputs. The result was an hour-long concert that explored the boundaries of human-AI musical collaboration. In this poster, we provide insights into the design and implementation of this unique concert experience. We focus on: (1) the iterative and collaborative design process with Rudess that led to the development of six distinct AI models tailored for real-time improvisation, (2) the techniques employed to optimize these models for seamless live interaction, (3) the creation of visual models and their applications to the kinetic sculpture to help the audience discern the contributions of the AI, and (4) the findings from our post-concert survey, offering valuable insights into audience perceptions of human-AI co-created performances. We believe that modern generative AI systems will play a significant role in the future of live music, and we aim to pave the way for future research in this domain with our findings.
- 31. Hierarchical Generative Modeling Of Melodic Vocal Contours In Hindustani Classical Music
Nithya Shikarpur, Krishna Maneesha Dendukuri, Yusong Wu, Antoine Caillon, Cheng-Zhi Anna Huang
MIT, Mila Quebec Artificial Intelligence Institute, Université de Montréal, Canada CIFAR AI Chair, Google DeepMindAbstract- Hindustani music is a performance-driven oral tradition that exhibits the rendition of rich melodic patterns. In this poster, we focus on generative modeling of singers' vocal melodies extracted from audio recordings, as the voice is musically prominent within the tradition. Prior generative work in Hindustani music models melodies as coarse discrete symbols that fail to capture the rich expressive melodic intricacies of singing. Thus, we propose to use a finely quantized pitch contour, as an intermediate representation for hierarchical audio modeling. We propose GaMaDHaNi, a modular two-level hierarchy, consisting of a generative model on pitch contours, and a pitch contour to audio synthesis model. We compare our approach to non-hierarchical audio models and hierarchical models that use a self-supervised intermediate representation, through a listening test and qualitative analysis. We also evaluate audio model's ability to faithfully represent the pitch contour input using Pearson correlation coefficient. By using pitch contours as an intermediate representation, we show that our model may be better equipped to listen and respond to musicians in a human-AI collaborative setting by highlighting two potential interaction use cases (1) primed generation, and (2) coarse pitch conditioning.
- 32. Efficient Detection of Audio Assault in Voice Chat: Replacing CNNs with Lightweight DSP Algorithms for Clipping Detection
Raquel Harrison, Orkun Bedir, Rachel Manzelli
ModulateAbstract- The detection of Audio Assault – disruptive and excessively loud sounds in video game voice chat – is crucial for maintaining quality and usability in many audio-based communication platforms. More recently, convolutional neural networks (CNNs) have been employed to detect such anomalies, and while CNNs provide a degree of accuracy, they are computationally expensive and slow in real-time applications, making them less practical for voice chat environments where low latency is essential. In this work, we propose a novel approach that replaces the traditional CNN-based detection model with a lightweight Digital Signal Processing (DSP) algorithm. The proposed algorithm, implemented in Python, is specifically designed to detect clipping – a form of waveform distortion that occurs when the audio signal exceeds the system’s capacity – with high precision while significantly reducing computational costs and latency. The DSP-based solution operates at a fraction of the cost and speed compared to the CNN model, offering a practical alternative for real-time applications. We evaluate both models using manually labeled test data that reflects various real-world audio conditions in voice chat. Our results demonstrate that the DSP algorithm not only outperforms the CNN in terms of speed but also achieves superior precision in detecting clipping events. This shift from deep learning-based approaches to efficient DSP algorithms represents a promising direction for handling audio assault detection in resource-constrained environments, such as video game voice chat.
- 33. Cost-Efficient Speech AI Optimization: Finetuning with Publicly Available Data at 90% Lower Data Costs
Wei Chu
OlewaveAbstract- This paper presents a cost-efficient approach to optimizing speech AI models through finetuning with publicly available data. Instead of relying on expensive, manually labeled datasets, we propose utilizing public data with a distribution that closely matches the client’s domain. Our data labeling solution follows three key steps: 1) Jump Start—curating and finetuning a model using a tailored public dataset with our Tycho SDK; 2) Auto Label—automatically labeling the client’s private data with the finetuned model, reducing human labeling costs and mitigating data breach risks; and 3) Iterate—creating a semi-automatic dataset of labeled public and private data for further finetuning. This iterative process enables continuous model improvement, enhancing both accuracy and cost-efficiency. Results from the Switchboard English conversation recognition task show our approach reduces the word error rate from 22.1% to 20.9%, while reducing data acquisition and labeling costs by 90%.
- 34. JAX Implementations of Descript Audio Codec and EnCodec
David Braun
Princeton UniversityAbstract- We present an open-source implementation of the Descript Audio Codec (DAC) using Google's JAX ecosystem of Flax, Optax, Orbax, AUX, and CLU. Our codebase enables the reuse of model weights from the original PyTorch DAC, and we confirm that the two implementations produce equivalent token sequences and decoded audio if given the same input. We provide a training and fine-tuning script which supports device parallelism, although we have only verified it using brief training runs with a small dataset. Even with limited GPU memory, the original DAC can compress or decompress a long audio file by processing it as a sequence of overlapping "chunks." We implement this feature in JAX and benchmark the performance on two types of GPUs. On a consumer-grade GPU, DAC-JAX outperforms the original DAC for compression and decompression at all chunk sizes. However, on a high-performance, cluster-based GPU, DAC-JAX outperforms the original DAC for small chunk sizes but performs worse for large chunks.
- 35. Graphic Equalizers Based on Limited Action Networks
Kurt James Werner
Soundtoys, Inc.Abstract- Several classic graphic equalizers, such as the Altec 9062A and the “Motown EQ,” have stepped gain controls and “proportional bandwidth” and used passive, constant-resistance, RLC circuit designs based on “limited-action networks.” These are related to bridged-T-network EQs, with several differences that cause important practical improvements, also affecting their sound. We study these networks, giving their circuit topologies, design principles, and design equations, which appear not to have been published before. We make a Wave Digital Filter which can model either device or an idealized “Exact” version, to which we can add various new extensions and features.
- 36. What do MLLMs hear? Examining reasoning with text and sound components in Multimodal Large Language Models
Enis Berk Çoban, Michael I Mandel, Johanna Devaney
The Graduate Center, CUNYAbstract- Large Language Models (LLMs) have demonstrated remarkable reasoning capabilities, notably in connecting ideas and adhering to logical rules to solve problems. These models have evolved to accommodate various data modalities, including sound and images, known as multimodal LLMs (MLLMs), which are capable of describing images or sound recordings. Previous work has demonstrated that when the LLM component in MLLMs is frozen, the audio or visual encoder serves to caption the sound or image input facilitating text-based reasoning with the LLM component. We are interested in using the LLM's reasoning capabilities in order to facilitate classification. In this paper, we demonstrate through a captioning/classification experiment that an audio MLLM cannot fully leverage its LLM's text-based reasoning when generating audio captions. We also consider how this may be due to MLLMs separately representing auditory and textual information such that it severs the reasoning pathway from the LLM to the audio encoder.
- 37. Learning Fine-Grained Controllability on Speech Generation via Efficient Fine-Tuning
Chung-Ming Chien, Andros Tjandra, Apoorv Vyas, Matt Le, Bowen Shi, Wei-Ning Hsu
TTI-Chicago, MetaAbstract- As the scale of generative models continues to grow, efficient reuse and adaptation of pre-trained models have become crucial considerations. In this work, we propose Voicebox Adapter, a novel approach that integrates fine-grained conditions into a pre-trained Voicebox speech generation model using a cross-attention module. To ensure a smooth integration of newly added modules with pre-trained ones, we explore various efficient fine-tuning approaches. Our experiment shows that the LoRA with bias-tuning configuration yields the best performance, enhancing controllability without compromising speech quality. Across three fine-grained conditional generation tasks, we demonstrate the effectiveness and resource efficiency of Voicebox Adapter. Follow-up experiments further highlight the robustness of Voicebox Adapter across diverse data setups.
- 38. Few-Shot Spoken Language Understanding via Joint Speech-Text Models
Chung-Ming Chien, Mingjiamei Zhang, Ju-Chieh Chou, Karen Livescu
TTI-Chicago, The University of ChicagoAbstract- Recent work on speech representation models jointly pre-trained with text has demonstrated the potential of improving speech representations by encoding speech and text in a shared space. In this paper, we leverage such shared representations to address the persistent challenge of limited data availability in spoken language understanding tasks. By employing a pre-trained speech-text model, we find that models fine-tuned on text can be effectively transferred to speech testing data. With as little as 1 hour of labeled speech data, our proposed approach achieves comparable performance on spoken language understanding tasks (specifically, sentiment analysis and named entity recognition) when compared to previous methods using speech-only pre-trained models fine-tuned on 10 times more data. Beyond the proof-of-concept study, we also analyze the latent representations. We find that the bottom layers of speech-text models are largely task-agnostic and align speech and text representations into a shared space, while the top layers are more task-specific.
- 39. FAST BLACK-BOX OPTIMIZERS FOR LOW DELAY AUDIO SOURCE SEPARATION
Gerald Schuller
TU IlmenauAbstract- The goal of this paper is to show that black box optimizers (also referred to as derivative free optimizers) recently made significant progress, such that they can also be used for signal processing applications, even for cases where only a limited time budged is available, as in online and real time applications, as shown in the presented audio source separation example. Another advantage is that they can be used, e.g. for recurrent neural networks, which have the problem of vanishing gradients. These applications are used to compare a set of black box optimizers, all also suitable for higher dimensional problems. The results show that a suitable selection and adaptation of an optimizer for an application is crucial. For the presented applications, the presented optimizer of "Random Directions" consistently performs among the best for finding a good minimum of the objective- or loss-functions, and also for the shortest processing time.
- 40. Sound Extraction Based on Audio Scene Understanding using LLMs
Shrishail Baligar, Brandon Pardi, Shawn Newsam
UC MercedAbstract- Enhancing human hearing and auditory experience remains an active research area with numerous potential applications. Multi-source Target Sound Extraction (TSE) is a technique used to suppress or enhance specific sounds within an audio scene, making desirable sounds more audible or creating a more pleasant auditory experience. The decision about what sounds should be emphasized can either be manually chosen or automated. In this work, we introduce the use of Large Language Models (LLMs) to reduce, and potentially eliminate, the need for human intervention in selecting the ideal sounds for a given environment. To evaluate the accuracy of LLMs in making these decisions, we conduct a user study across multiple sound scenes, measuring how closely LLMs' judgments align with human decision-making in real-world environments. Furthermore, we personalize the LLM's behavior by incorporating user feedback, refining the system's preferences for greater personalization. Finally, we quantify the improvements gained by this user-feedback-driven customization and demonstrate the effectiveness of LLM-recommender-based TSE in a real-time, causal setting.
- 41. CATSE: A Context-Aware Framework for Causal Target Sound Extraction
Shrishail Baligar, Mikolaj Kegler, Bryce Irvin, Marko Stamenovic, Shawn Newsam
UC Merced, Bose CorporationAbstract- Target Sound Extraction (TSE) focuses on the problem of separating sources of interest, indicated by a user's cue, from the input mixture. Most existing solutions operate in an offline fashion and are not suited to the low-latency causal processing constraints imposed by applications in live-streamed content such as augmented hearing. We introduce a family of context-aware low-latency causal TSE models suitable for real-time processing. First, we explore the utility of context by providing the TSE model with oracle information about what sound classes make up the input mixture, where the objective of the model is to extract one or more sources of interest indicated by the user. Since the practical applications of oracle models are limited due to their assumptions, we introduce a composite multi-task training objective involving separation and classification losses. Our evaluation involving single- and multi-source extraction shows the benefit of using context information in the model either by means of providing full context or via the proposed multi-task training loss without the need for full context information. Specifically, we show that our proposed model outperforms size- and latency-matched Waveformer, a state-of-the-art model for real-time TSE.
- 42. BeatNet+: Real-Time Rhythm Analysis for Diverse Music Audio
Mojtaba Heydari, Zhiyao Duan
University of RochesterAbstract- This paper presents a comprehensive study on real-time music rhythm analysis, covering joint beat and downbeat tracking for diverse kinds of music signals. We introduce BeatNet+, a two-stage approach to real-time rhythm analysis built on a previous state-of-the-art method named BeatNet. The main innovation of the proposed method is the auxiliary training strategy that helps the neural network model to learn a representation invariant to the amount of percussive components in the music. Together with other architectural improvements, this strategy significantly improves the model performance for generic music. Another innovation is on the adaptation strategies that help develop real-time rhythm analysis models for challenging music scenarios including isolated singing voices and non-percussive music. Two adaptation strategies are proposed and experimented with different neural architectures and training schemes. Comprehensive experiments and comparisons with multiple baselines are conducted, and results show that BeatNet+ achieves superior beat tracking and downbeat tracking F1 scores for generic music, isolated singing voices and non-percussive audio, with competitive latency and computational complexity. Finally, we release beat and downbeat annotations for two datasets that are designed for other tasks, and revised annotations of three existing datasets. We also release the code repository and pre-trained models on GitHub.
- 43. Scoring Time Intervals Using Non-Hierarchical Transformer for Automatic Piano Transcription
Yujia Yan, Zhiyao Duan
University of RochesterAbstract- The neural semi-Markov Conditional Random Field (semi-CRF) framework has demonstrated promise for event-based piano transcription. In this framework, all events (notes or pedals) are represented as closed {time} intervals tied to specific event types. The neural semi-CRF approach requires an interval scoring matrix that assigns a score for every candidate interval. However, designing an efficient and expressive architecture for scoring intervals is not trivial. This paper introduces a simple method for scoring intervals using scaled inner product operations that resemble how attention scoring is done in transformers. We show theoretically that, due to the special structure from encoding the non-overlapping intervals, under a mild condition, the inner product operations are expressive enough to represent an ideal scoring matrix that can yield the correct transcription result. We then demonstrate that an encoder-only non-hierarchical transformer backbone, operating only on a low-time-resolution feature map, is capable of transcribing piano notes and pedals with high accuracy and time precision. The experiment shows that our approach achieves the new state-of-the-art performance across all subtasks in terms of the F1 measure on the Maestro dataset.
- 44. Note-Level Transcription of Choral Music
Huiran Yu, Zhiyao Duan
University of RochesterAbstract- Choral music is a musical activity with one of the largest participant bases, yet it has yet to draw much attention from automatic music transcription research. The main reasons we argue are the lack of data and technical difficulties arising from diverse acoustic conditions and unique properties of choral singing. To address these challenges, we propose a Transformer-based framework for note-level transcription of choral music. This framework bypasses the frame-level processing and directly produces a sequence of notes with associated timestamps. We also introduce YouChorale, a novel choral music dataset in an a cappella setting curated from the Internet. YouChorale contains 452 real-world recordings in diverse acoustic configurations of choral music from over 100 composers as well as their MIDI scores. Trained on YouChorale, our proposed model achieves state-of-the-art performance in choral music transcription, marking a significant advancement in the field.
- 45. Leveraging Self-Supervised Learning for Multi-Pitch Estimation
Frank Cwitkowitz, Zhiyao Duan
University of RochesterAbstract- Multi-pitch estimation is a decades-long research problem involving the detection of pitch activity associated with concurrent musical events within polyphonic music. Supervised learning techniques have demonstrated solid performance on more narrow characterizations of the task, such as the transcription of solo piano recordings. However, supervised methods suffer in more general settings due to limitations concerning the shortage of large-scale and diverse polyphonic music datasets with high-quality multi-pitch annotations. In this work, we present a suite of self-supervised learning objectives for multi-pitch estimation, which encourage the concentration of support around harmonics, invariance to timbre, equivariance to time and frequency transformations, and invariance to percussion. These objectives are sufficient to train a fully convolutional neural network to produce multi-pitch salience-grams on either monophonic or polyphonic audio, without any fine-tuning on annotated data. Furthermore, we provide some preliminary results when combining the proposed self-supervised objectives with supervised learning on a small dataset with accompanying multi-pitch annotations.
- 46. GTR-Voice: Articulatory Phonetics Informed Controllable Expressive Speech Synthesis
Zehua Kcriss Li, Meiying Melissa Chen, Yi Zhong, Pinxin Liu, Zhiyao Duan
University of RochesterAbstract- Expressive speech synthesis aims to generate speech that captures a wide range of para-linguistic features, including emotion and articulation, though current research primarily emphasizes emotional aspects over the nuanced articulatory features mastered by professional voice actors. Inspired by this, we explore expressive speech synthesis through the lens of articulatory phonetics. Specifically, we define a framework with three dimensions: Glottalization, Tenseness, and Resonance (GTR), to guide the synthesis at the voice production level. With this framework, we record a high-quality speech dataset named GTR-Voice, featuring 20 Chinese sentences articulated by a professional voice actor across 125 distinct GTR combinations. We verify the framework and GTR annotations through automatic classification and listening tests, and demonstrate precise controllability along the GTR dimensions on two fine-tuned expressive TTS models. We open-source the dataset and TTS models.
- 47. SVDD 2024: The Inaugural Singing Voice Deepfake Detection Challenge
You Zhang, Yongyi Zang, Jiatong Shi, Ryuichi Yamamoto, Tomoki Toda, Zhiyao Duan
University of Rochester, CMU, Nagoya UniversityAbstract- With the advancements in singing voice generation and the growing presence of AI singers on media platforms, the inaugural Singing Voice Deepfake Detection (SVDD) Challenge aims to advance research in identifying AI-generated singing voices from authentic singers. This challenge features two tracks: a controlled setting track (CtrSVDD) and an in-the-wild scenario track (WildSVDD). The CtrSVDD track utilizes publicly available singing vocal data to generate deepfakes using state-of-the-art singing voice synthesis and conversion systems. Meanwhile, the WildSVDD track expands upon the existing SingFake dataset, which includes data sourced from popular user-generated content websites. For the CtrSVDD track, we received submissions from 47 teams, with 37 surpassing our baselines and the top team achieving a 1.65% equal error rate. For the WildSVDD track, we benchmarked the baselines. This paper reviews these results, discusses key findings, and outlines future directions for SVDD research.
- 48. Learning Audio Concepts from Counterfactual Natural Language
Ali Vosoughi, Luca Bondi, Ho-Hsiang Wu, Chenliang Xu
University of Rochester, Bosch AI ResearchAbstract- Conventional audio classification relied on predefined classes, lacking the ability to learn from free-form text. Recent methods unlock learning joint audio-text embeddings from raw audio-text pairs describing audio in natural language. Despite recent advancements, there is little exploration of systematic methods to train models for recognizing sound events and sources in alternative scenarios, such as distinguishing fireworks from gunshots at outdoor events in similar situations. This study introduces causal reasoning and counterfactual analysis in the audio domain. We use counterfactual instances and include them in our model across different aspects. Our model considers acoustic characteristics and sound source information from human-annotated reference texts. To validate the effectiveness of our model, we conducted pre-training utilizing multiple audio captioning datasets. We then evaluate with several common downstream tasks, demonstrating the merits of the proposed method as one of the first works leveraging counterfactual information in audio domain. Specifically, the top-1 accuracy in open-ended language-based audio retrieval task increased by more than 43%.
- 49. AVVA: Audio-Video Vector Alignment on Unlabeled Data Curated with Multimodal Reasoning
Ali Vosoughi, Dimitra Emmanouilidou, Hannes Gamper
University of Rochester, Microsoft ResearchAbstract- Integrating audio and visual data for training multimodal foundational models remains a challenge. The Audio-Video Vector Alignment (AVVA) framework addresses this by considering AV scene alignment beyond mere temporal synchronization, and leveraging Large Language Models (LLMs) for data curation. AVVA implements a scoring mechanism for selecting aligned training data segments. It integrates Whisper, a speech-based foundation model, for audio and DINOv2 for video analysis in a dual-encoder structure with contrastive learning on AV pairs. Evaluations on AudioCaps, VALOR, and VGGSound demonstrate the effectiveness of the proposed model architecture and data curation approach. AVVA achieves a 7.6% improvement in top-1 accuracy for audio-to-video retrieval on VGGSound compared to ImageBind, while using only 192 hrs of curated training data (compared to ImageBind’s 5800 hrs). Furthermore, an ablation study indicates that the data curation process effectively trades data quality for data quantity, yielding increases of 47.8, 48.4, and 58.0 percentage points in top-3 accuracies on AudioCaps, VALOR, and VGGSound, respectively, compared to training on uncurated data.
- 50. Joint Music and Language Attention Models for Zero-shot Music Tagging
Xingjian Du, Zhesong Yu, Jiaju Lin, Bilei Zhu, Qiuqiang Kong
University of Rochester, Bytedance, The Chinese University of HongkongAbstract- Our poster presents a novel approach to zero-shot music tagging using a joint music and language attention (JMLA) model. This work addresses the open-set music tagging problem, extending beyond the limitations of traditional close-set tagging tasks. Our JMLA model combines a pretrained masked autoencoder as an audio encoder with a Falcon7B decoder. Key innovations include the introduction of a preceiver resampler for handling arbitrary-length audio inputs and dense attention connections to enhance information flow between encoder and decoder layers. We utilized a large-scale music and description dataset, enhanced by ChatGPT-generated formalized and diverse descriptions, to train our JMLA models. Our system achieves a zero-shot audio tagging accuracy of 64.82% on the GTZAN dataset, surpassing previous zero-shot systems. Additionally, it demonstrates comparable performance on the FMA and MagnaTagATune datasets.
- 51. Controversial sounds for encoding models of auditory cortex
David Skrill, Jenelle Feather, Sam Norman-Haignere
University of Rochester Medical Center, Flatiron InstituteAbstract- Deep neural networks (DNNs) are currently state-of-the-art models for predicting brain responses to complex natural stimuli such as speech and music, and there is substantial interest in testing the extent to which the computations in these models reflect those in the brain. To accomplish this goal, it is necessary to distinguish between competing models that instantiate different hypotheses about the underlying neuronal computations. However, in practice, distinct models often make similar predictions for neural data due to correlations between model features across natural stimuli. For example, there is growing evidence that adversarial training causes DNNs to better match human perception in terms of the model’s perceptual invariances, yet standard and adversarially trained models have virtually identical accuracy when predicting neural responses to natural stimuli. Here, we develop a method to synthesize “controversial stimuli” that best differentiate two competing models of sensory brain responses, and apply this method to standard and adversarially trained audio DNNs. In particular, we show that our method is able to synthesize a single sound set that is universally controversial, such that standard and adversarially trained models make different predictions for these sounds in virtually all regions of the auditory cortex across many different subjects, measured with both functional MRI and intracranial EEG recordings from human neurosurgical patients. The method is applicable to any two differentiable models and thus provides a promising approach for testing computational models of sensory responses.
- 52. A Critical Assessment of Visual Sound Source Localization Models Including Negative Audio
Xavier Juanola-Molet, Gloria Haro, Magdalena Fuentes
UPF (Universitat Pompeu Fabra), NYUAbstract- The task of Visual Sound Source Localization (VSSL) involves identifying the location of sound sources in visual scenes, integrating audio-visual data for enhanced scene understanding. Despite advancements in state-of-the-art (SOTA) models, we observe three critical flaws: i) The evaluation of the models is mainly focused on sounds produced by objects that are visible in the image, ii) The evaluation often assumes prior knowledge of the size of the sounding object, and iii) No universal threshold for localization in real-world scenarios is established, as previous approaches only consider positive examples without accounting for both positive and negative cases. In this paper, we introduce a novel test set and metrics designed to complete the current standard evaluation of VSSL models by testing them in scenarios where none of the objects in the image correspond to the audio input, i.e., a negative audio. We consider three types of negative audio: silence, noise, and offscreen. Our analysis reveals that numerous SOTA models fail to appropriately adjust their predictions based on audio input, suggesting that these models may not be leveraging audio information as intended. Additionally, we provide a comprehensive analysis of the range of maximum values in the estimated audio-visual similarity maps in both positive and negative audio cases, showing that most of the models are not discriminative enough, making them unfit to choose a universal threshold appropriate to perform sound localization without any a priori information about the sounding object, such as object size and visibility.
- 53. Speaker Diarization in the Classroom: How Much Does Each Student Speak in Group Discussions?
Jiani Wang, Shiran Dudy, Xinlu He, Zhiyong Wang, Rosy Southwell and Jacob Whitehill
Worcester Polytechnic InstituteAbstract- One important dimension of classroom group dynamics collaboration is how much each person contributes to the discussion. With the goal of measuring how much each student speaks, we investigate how automatic speaker diarization can be built to handle real-world classroom group discussions. We examine key design considerations such as the level of granularity of speaker assignment, speech enhancement techniques, voice activity detection, and embedding assignment method, so as to find an effective configuration. The best speaker diarization that we found was based on the ECAPA-TDNN speaker embedding model and used Whisper automatic speech recognition to find speech segments. Diarization error rates (DER) on challenging noisy spontaneous classroom data were around 34%, and the correlations of estimated vs. human annotations of how much each student spoke reached 0.62. The presented diarization system has potential to benefit educational research and also to give teachers and students useful feedback to understand their group dynamics.