SANE 2018 - Speech and Audio in the Northeast
October 18, 2018
The workshop is now over. Videos and slides for the talks are now available through the links in the schedule below. There is also a YouTube Playlist for all talks.
SANE 2018, a one-day event gathering researchers and students in speech and audio from the Northeast of the American continent, was held on Thursday October 18, 2018 at Google, in Cambridge, MA. It was by far the largest Boston-area SANE event, with 170 participants.
It was the 7th edition in the SANE series of workshops, which started in 2012. Since the first edition, the audience has steadily grown, with a record 180 participants in New York in 2017.
SANE 2018 featured invited talks by leading researchers from the Northeast as well as from the international community. It also featured a lively poster session, open to both students and researchers.
Details
- Date: Thursday, October 18, 2018
- Venue: Google, Cambridge, MA
Schedule [Watch all recorded talks on YouTube]
Click on the talk title to jump to the abstract and bio.
Registration
The workshop is now over. If you are interested in attending future SANE events, please sign up to the SANE News mailing list.
Directions
The workshop was hosted at Google, in Cambridge, MA. Google Cambridge is located at 355 Main St, right next to the Kendall/MIT station on the Red Line T (the subway).
Organizing Committee
- Jonathan Le Roux (MERL)
- Kevin Wilson (Google)
- John Hershey (Google)
- Shinji Watanabe (Johns Hopkins)
Sponsors
Talks
Learning to see and hear
One of the key reasons for the recent successes in computer vision is the access to massive annotated datasets that have become available in the last few years. Unfortunately, creating these datasets is expensive and labor intensive. On the other hand, babies learn with very little supervision, and, even when supervision is present, it comes in the form of an unknown spoken language that also needs to be learned. How can kids make sense of the world? In this work, I will show that an agent that has access to multimodal data (like vision and audition) can use the correlation between images and sounds to discover objects in the world without supervision. I will show that ambient sounds can be used as a supervisory signal for learning to see and vice versa (the sound of crashing waves, the roar of fast-moving cars – sound conveys important information about the objects in our surroundings). I will describe an approach that learns, by watching videos without annotations, to locate image regions that produce sounds, and to separate the input sounds into a set of components that represents the sound from each pixel. I will also show how we can use raw speech descriptions of images to jointly learn to segment words in speech and objects in images without any additional supervision.
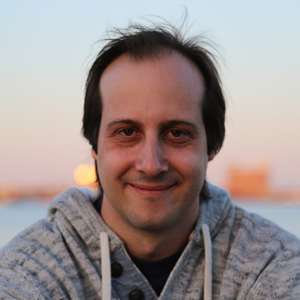
Antonio Torralba is a Professor of Electrical Engineering and Computer Science at the Massachusetts Institute of Technology (MIT), the MIT director of the MIT-IBM Watson AI Lab, and the inaugural director of the MIT Quest for Intelligence, a MIT campus-wide initiative to discover the foundations of intelligence. He received the degree in telecommunications engineering from Telecom BCN, Spain, in 1994 and the Ph.D. degree in signal, image, and speech processing from the Institut National Polytechnique de Grenoble, France, in 2000. From 2000 to 2005, he spent postdoctoral training at the Brain and Cognitive Science Department and the Computer Science and Artificial Intelligence Laboratory, MIT, where he is now a professor. Prof. Torralba is an Associate Editor of the International Journal in Computer Vision, and has served as program chair for the Computer Vision and Pattern Recognition conference in 2015. He received the 2008 National Science Foundation (NSF) Career award, the best student paper award at the IEEE Conference on Computer Vision and Pattern Recognition (CVPR) in 2009, and the 2010 J. K. Aggarwal Prize from the International Association for Pattern Recognition (IAPR). In 2017, he received the Frank Quick Faculty Research Innovation Fellowship and the Louis D. Smullin ('39) Award for Teaching Excellence.
Looking to Listen: Audio-Visual Speech Separation
People are remarkably good at focusing their attention on a particular person in a noisy environment while “muting” all other voices and sounds. The capability, known as the cocktail party effect, comes natural to us humans. However, achieving it computationally remains a significant challenge. In this talk, I’ll present a new deep network-based model that incorporates both visual and auditory signals to solve this task. The visual features are used to "focus" the audio on desired speakers in a scene and to improve the speech separation quality. The input to our model is a video (frames + single audio tack) and the output is a clean audio tracks for each of the speakers in the video. We are then able to produce videos in which speech of specific people is enhanced while all other sounds are suppressed. To train our joint audio-visual model, we introduce AVSpeech, a new dataset comprised of thousands of hours of video segments from the Web. I’ll demonstrate the quality of our speech separation results on a variety of real-world scenarios involving heated interviews, noisy bars, and screaming children, only requiring the user to select the face of the person in the video whose speech they want to isolate.
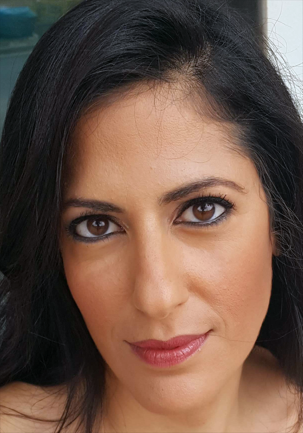
Tali Dekel is a Senior Research Scientist at Google, Cambridge, developing algorithms at the intersection of computer vision and computer graphics. Before Google, she was a Postdoctoral Associate at the Computer Science and Artificial Intelligence Lab (CSAIL) at MIT, working with Prof. William T. Freeman. Tali completed her Ph.D studies at the school of electrical engineering, Tel-Aviv University, Israel, under the supervision of Prof. Shai Avidan, and Prof. Yael Moses. Her research interests include computational photography, image synthesize, geometry and 3D reconstruction.
End-to-end speech recognition in incomplete data scenarios
Building an automatic speech recognition (ASR) system is very expensive and time consuming, since it requires well-maintained acoustic and linguistic resources such as pronunciation dictionaries and a large amount of paired speech and text data to achieve high recognition accuracy. End-to-end ASR is an approach to alleviate such development cost of ASR systems, which eliminates the need for pronunciation dictionaries by learning a direct mapping from audio features to the corresponding character or word sequences using a deep network. Moreover, the end-to-end approach is beneficial in terms of recognition accuracy since the deep network can learn tightly coupled speech and language behaviors. Some end-to-end ASR systems have already achieved comparable or better performance than conventional systems in several benchmark tasks. However, such end-to-end systems generally require more training data than the conventional ones as they need to learn a more complex mapping function, and it has proven difficult to outperform conventional systems with a small amount of data. Solving this problem is crucial for deploying ASR systems to a large number of languages including low-resource ones. In this talk, I will present recent end-to-end ASR advances in low-resource scenarios, which have been developed mainly within the “Multilingual end-to-end speech recognition for incomplete data” project of the 2018 Jelinek summer workshop. In this project, the research team has explored novel end-to-end ASR techniques in different low-resource conditions, e.g., (1) low-resource sequence-to-sequence learning with hybrid CTC/attention, (2) transfer learning in multi-lingual end-to-end ASR for low-resource languages, and (3) end-to-end training with unpaired data based on back translation and cycle consistency. This talk will include an overview of the project and the latest results.
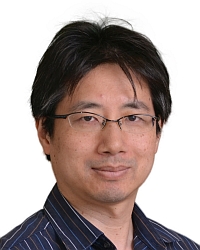
Takaaki Hori received the B.E. and M.E. degrees in electrical and information engineering from Yamagata University, Yonezawa, Japan, in 1994 and 1996, respectively, and the Ph.D. degree in system and information engineering from Yamagata University in 1999. From 1999 to 2015, he had been engaged in researches on speech recognition and understanding at Cyber Space Laboratories and Communication Science Laboratories in Nippon Telegraph and Telephone (NTT) Corporation, Japan. He was a visiting scientist at the Massachusetts Institute of Technology (MIT) from 2006 to 2007. In 2015, he joined Mitsubishi Electric Research Laboratories (MERL), Cambridge, Massachusetts, USA, and currently he is a senior principal research scientist at MERL. He has authored more than 100 peer-reviewed papers in speech and language research fields. He received the 24th TELECOM System Technology Award from the Telecommunications Advancement Foundation in 2009, the IPSJ Kiyasu Special Industrial Achievement Award from the Information Processing Society of Japan in 2012, and the 58th Maejima Hisoka Award from Tsushinbunka Association in 2013.
Distant microphone conversational speech recognition in domestic environments: Some initial outcomes of the 5th CHiME challenge.
The CHiME challenge series has been aiming to advance robust automatic speech recognition technology by promoting research at the interface of speech and language processing, signal processing and machine learning. This talk presents the 5th CHiME Challenge, which has considered the task of distant multi-microphone conversational speech recognition in domestic environments. The talk will present an overview of the CHiME-5 dataset, a fully-transcribed audio-video dataset that has captured 50 hours of audio from 20 separate dinner parties held in real homes each with 6 video channels and 32 audio channels. The talk will discuss the design of the light-weight recording set up that allowed for highly natural data to be recorded. The talk will present some analysis of the data highlighting the major sources of difficulty it presents for recognition systems. The talk will then present the outcomes of the challenge itself which attracted submissions from 19 teams submitting systems to single device or multiple device tracks. In particular, we will look at which techniques worked and which did not, and use the outcomes to identify priorities for future research and future challenges.
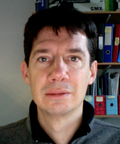
Jon Barker is a Professor in the Computer Science Department at the University of Sheffield. He received his degree from University of Cambridge (1991) and the Ph.D. from the University of Sheffield (1998). His research interests include human speech processing, speech intelligibility modelling and human-inspired approaches to speech separation and recognition. He has made significant contributions to the development of missing data speech recognition and statistical auditory scene analysis. In more recent years this has led to an interest in robust processing for distant microphone speech recognition. In 2011 he co-founded the CHiME series of workshops and evaluations for speech recognition and separation which are now in their 5th iteration.
Attention at the cocktail party: cognitive control of auditory processing
In our daily lives, we are constantly challenged to attend to specific sound sources or follow particular conversations in the midst of competing background chatter - a phenomenon referred to as the ‘cocktail party problem’. While completely intuitive in humans and animals alike, it is a multifaceted challenge whose neural underpinnings and theoretical formulations are not fully understood. In this talk, I discuss the role of the neural coding of complex sounds in the auditory system and particularly the adaptive processes induced by attentional feedback mechanisms. A growing body of work has been amending our views of processing in the auditory system; replacing the conventional view of ‘static’ processing in sensory cortex with a more ‘active’ and malleable mapping that rapidly adapts to the task at hand and listening conditions. After all, humans and most animals are not specialists, but generalists whose perception is shaped by experience, context and changing behavioral demands. Leveraging these attentional capabilities in audio technologies leads to promising improvements in our ability to track sounds of interest amidst competing distracters.
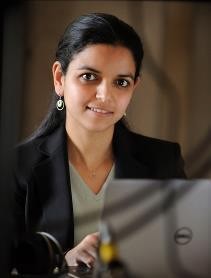
Mounya Elhilali received her Ph.D. degree in Electrical and Computer Engineering from the University of Maryland, College Park in 2004. She is now associate professor of Electrical and Computer Engineering at the Johns Hopkins University. She directs the Laboratory for Computational Audio Perception and is affiliated with the Center for Speech and Language Processing and the Center for Hearing and Balance. Her research examines sound processing by humans and machines in noisy soundscapes, and investigates reverse engineering intelligent processing of sounds by brain networks with applications to speech and audio technologies and medical systems. She was named the Charles Renn faculty scholar in 2015 and received a Johns Hopkins catalyst award in 2017. Dr. Elhilali is the recipient of the National Science Foundation CAREER award and the Office of Naval Research Young Investigator award.
Towards End-to-end Speech Synthesis
We describe a neural network-based system for text-to-speech (TTS) synthesis that is able to generate speech audio in the voice of different speakers and learn to model a large range of acoustic expressiveness, such as speed and speaking style. Our system consists of three independently trained components: (1) a speaker encoder network, trained on a speaker verification task using an independent dataset of noisy speech without transcripts from thousands of speakers, to generate a fixed-dimensional embedding vector from just seconds of reference speech from a target speaker; (2) a bank of embeddings called global style token to model different styles; (3) a sequence-to-sequence synthesis network based on Tacotron 2 that generates a mel spectrogram from text, conditioned on the speaker and style embedding; We demonstrate that (1) the speaker embedding model is able to transfer the knowledge of speaker variability learned by the discriminatively-trained speaker encoder to the multispeaker TTS task, and is able to synthesize natural speech from speakers unseen during training; (2) the style embedding model can learn different acoustic condition independent of speakers and text.
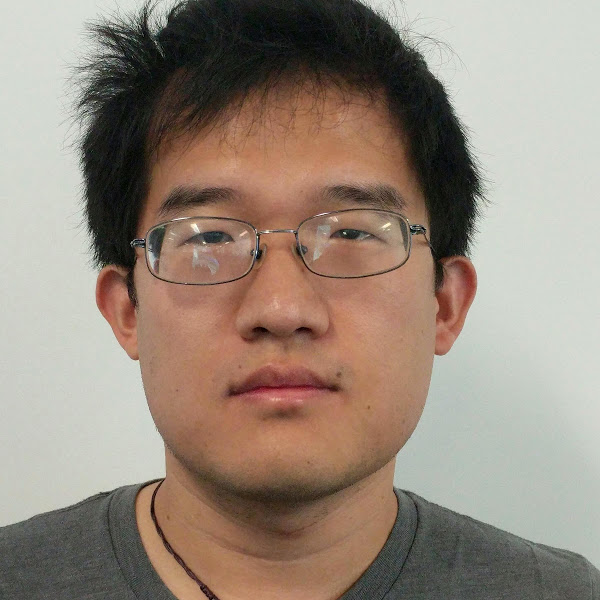
Yu Zhang is a research scientist at Google, where his research focuses on improving ML model performance for various speech processing applications. Currently, he is working on end-to-end ASR and TTS. Before coming to Google, he completed his Ph.D. at Massachusetts Institute of Technology, where his advisor was James Glass. Most of his Ph.D. work has been focused on automatic speech recognition using neural networks.
Robust Sound Event Detection in Acoustic Sensor Networks
The combination of remote acoustic sensors with automatic sound recognition represents a powerful emerging technology for studying both natural and urban environments. At NYU we've been working on two projects whose aim is to develop and leverage this technology: the Sounds of New York City (SONYC) project is using acoustic sensors to understand noise patterns across NYC to improve noise mitigation efforts, and the BirdVox project is using them for the purpose of tracking bird migration patterns in collaboration with the Cornell Lab of Ornithology. Acoustic sensors present both unique opportunities and unique challenges when it comes to developing machine listening algorithms for automatic sound event detection: they facilitate the collection of large quantities of audio data, but the data are unlabeled, constraining our ability to leverage supervised machine learning algorithms. Training generalizable models becomes particularly challenging when training data come from a limited set of sensor locations (and times), and yet our models must generalize to unseen natural and urban environments with unknown and sometimes surprising confounding factors. In this talk I will present our work towards tackling these challenges along several different lines with neural network architectures, including novel pooling layers that allow us to better leverage weakly labeled training data, self-supervised audio embeddings that allow us to train high-accuracy models with a limited amount of labeled data, and context-adaptive networks that improve the robustness of our models to heterogenous acoustic environments.
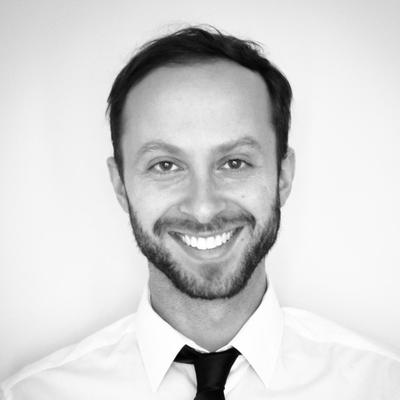
Justin Salamon is a Senior Research Scientist at New York University’s Music and Audio Research Laboratory and Center for Urban Science and Progress. He received a B.A. degree (2007) in Computer Science from the University of Cambridge, UK and M.Sc. (2008) and Ph.D. (2013) degrees in Computer Science from Universitat Pompeu Fabra, Barcelona, Spain. In 2011 he was a visiting researcher at IRCAM, Paris, France. In 2013 he joined NYU as a postdoctoral researcher, where he has been a Senior Research Scientist since 2016. His research focuses on the application of machine learning and signal processing to audio signals, with applications in machine listening, music information retrieval, bioacoustics, environmental sound analysis and open source software and data. For further information please see: www.justinsalamon.com.
Poster Session
- Audio Visual Scene-aware Dialog at DSTC7
Chiori Hori, Huda Alamri, Jue Wang, Gordon Wichern, Takaaki Hori, Anoop Cherian, Tim K. Marks, Dhruv Batra, Devi Parikh (MERL, Georgia Tech)Abstract- Natural spoken language interaction between humans and robots has been a long-standing dream of artificial intelligence. Recently, spoken dialog technologies have been applied in real-world man-machine interfaces including smartphone digital assistants, car navigation, voice-controlled speakers, and human-facing robots. Traditional dialog systems rely on hand-crafted rules to support a limited task domain, such as a query of information from a database. In this talk, we introduce deep learning architectures that combine spoken dialog technologies and multimodal attention-based video description technologies to realize a novel Audio-Visual Scene-Aware Dialog (AVSD) framework. These models can generate unified semantic representations of natural language and audio-visual inputs, which facilitate flexible discourse about a scene. Our goal for AVSD is to identify and detail the events in the video through dialog. Experiments are conducted based on dialogs consisting of 10 QAs and a summary for the Charades dataset, which captures people performing everyday actions in real-world settings with natural audio. This work represents a key step toward real-world human-robot interaction and will be a focal point of the 7th Dialog System Technology Challenge (DSTC7).
- Human auditory scene analysis as neurally guided Bayesian inference in sound source models
Maddie Cusimano, Luke B. Hewitt, Joshua B. Tenenbaum, Josh H. McDermott (MIT)Abstract- Inferring individual sound sources from the mixture of soundwaves that enters our ear is a central problem in auditory perception, termed auditory scene analysis (ASA). The study of ASA has uncovered a diverse set of illusions that suggest general principles underlying perceptual organization. However, most existing models of human perception focus on only a narrow subset of illusions or do not operate on the raw soundwave. To move toward a more comprehensive account, we frame ASA as analysis-by-synthesis in a probabilistic model based on representations of acoustic sources. Due to the rich structure of our generative model, inference from raw soundwaves poses a significant computational challenge. We overcome this by first training a deep neural network on sounds generated by the model, and then using this network to guide Markov chain Monte Carlo inference. Given a sound waveform, our system infers the number of sources present, parameters defining each source, and the sound produced by each source. This model qualitatively accounts for perceptual judgments on a variety of ASA illusions, and can in some cases infer perceptually valid sources from simple naturalistic audio.
- Auditory Texture Models Derived from Task-Optimized Deep Neural Network Representations
Jenelle Feather and Josh McDermott (MIT)Abstract- Textures are distinguished from other sound signals by homogeneity in time. The brain is believed to take advantage of this homogeneity by representing textures with statistics that average information across time. Models of texture perception are based on such statistics, and are commonly evaluated by synthesizing stimuli that produce the same representation in the model as a natural stimulus. Such synthetic sounds should evoke the same texture percept as the natural sound if the model replicates the representations underlying texture perception. Prior auditory (and visual) texture models produce textures that resemble natural textures, but use ad hoc statistics derived through trial and error. Further, traditional texture models rely on statistics measured from multiple stages of the underlying sensory cascade (for instance, statistics from cochlear filters as well as subsequent stages of modulation filters), but it is arguably implausible that perceptual decisions could be based directly on the output from the cochlea. We explored whether a single, simple class of statistic measured at a single stage of an auditory model could replicate the multistage, multistatistic representation of traditional texture models. We compared textures generated from three different sets of statistics: (1) the power from each of the first layer filters from a taskoptimized convolutional neural network, (2) the power from each of a set of spectrotemporal filters commonly used as a model of primary auditory cortex, and (3) statistics in the the McDermott and Simoncelli (2011) texture model (consisting of power and other marginal statistics measured from cochlear and temporal modulation filters, as well as correlations between filters). When cochlear statistics were included in the synthesis constraints, the learned filters and the spectrotemporal filters both produced textures that were as realistic and recognizable as those from the McDermott and Simoncelli model. However, when cochlear statistics were omitted, only textures generated from the learned filters maintained a high level of realism and recognizability – textures generated from the spectrotemporal filters became less realistic and recognizable when not explicitly constrained by cochlear statistics. These results held across convolutional networks trained on three different tasks: word identification, speaker identification, and genre classification. The results suggest that the learned filters incorporate peripheral information that matters for a task and that matters for perception, and that texture information could be represented at a single stage of cortical representation.
- Neural Networks Trained to Estimate F0 from Natural Sounds Replicate Properties of Human Pitch Perception
Mark R. Saddler, Ray Gonzalez and Josh H. McDermott (MIT, Harvard)Abstract- Despite a wealth of psychophysical data, developing computational models that account for pitch perception has proven challenging. In human listeners, the pitch of a sound depends on both spectral and temporal information available in the auditory periphery, but the relative contribution of the various cues and the reasons for their varying importance remain poorly understood. We investigated whether the properties of human pitch perception would emerge simply from optimizing a general-purpose architecture to estimate fundamental frequency (F0) from cochlear representations of natural sounds. We trained a convolutional neural network to classify simulated auditory nerve representations of speech and instrument sounds according to their F0. An established model of the auditory periphery (Bruce et al. 2018) was used to simulate the instantaneous firing rate responses of 50 auditory nerve fibers. Once trained, we simulated psychophysical experiments on the network. Pitch discrimination thresholds measured from the trained neural network replicated many of the known dependencies of human pitch discrimination on stimulus parameters such as harmonic composition and relative phase. Discrimination thresholds were best for stimuli containing low-numbered harmonics that were resolved by the cochlear filters and increased for stimuli containing only higher-numbered, unresolved harmonics. Randomizing the relative phase of harmonic components worsened pitch discrimination performance only when harmonics were unresolved, indicating the network learned to use temporal cues for pitch extraction when spectral cues were unavailable. Furthermore, the trained network qualitatively replicated human pitch judgments on a number of classic psychoacoustic manipulations (pitch-shifted inharmonic complexes, mistuned harmonics, transposed tones, alternating-phase harmonic complexes). We also simulated neurophysiological experiments on the trained network and found units in the later convolutional layers that exhibited pitch-tuning and selectivity for either resolved or unresolved harmonics. To better understand how the dependencies of pitch perception arise from either constraints of the peripheral auditory system or from statistics of sounds in the world, we independently manipulated parameters of the peripheral model and the training corpus, and found that each altered the network’s performance characteristics. The results collectively suggest that human pitch perception can be understood as having been optimized to estimate the fundamental frequency of natural sounds heard through a human cochlea.
- Unsupervised cross-modal alignment of speech and text embedding spaces
Yu-An Chung, Wei-Hung Weng, Schrasing Tong, and James Glass (MIT)Abstract- Recent research has shown that word embedding spaces learned from text corpora of different languages can be aligned without any parallel data supervision. Inspired by the success in unsupervised cross-lingual word embeddings, in this paper we target learning a cross-modal alignment between the embedding spaces of speech and text learned from corpora of their respective modalities in an unsupervised fashion. The proposed framework learns the individual speech and text embedding spaces, and attempts to align the two spaces via adversarial training, followed by a refinement procedure. We show how our framework could be used to perform spoken word classification and translation, and the experimental results on these two tasks demonstrate that the performance of our unsupervised alignment approach is comparable to its supervised counterpart. Our framework is especially useful for developing automatic speech recognition (ASR) and speech-to-text translation systems for low- or zero-resource languages, which have little parallel audio-text data for training modern supervised ASR and speech-to-text translation models, but account for the majority of the languages spoken across the world.
- Investigation of Deep Neural Networks in a Brain-Computer Interface for Two-Talker Attention Decoding
Gregory Ciccarelli, Michael Nolan, Joey Perricone, Paul Calamia, Thomas Quatieri, James O’Sullivan, Nima Mesgarani, Christopher Smalt (MIT Lincoln Laboratory, Columbia University)Abstract- A practical solution to the cocktail party problem -selecting the desired talker from a mixture and presenting only that speech to a listener- requires two components: speech separation and attention decoding. In this work, we compare the accuracy of decoding listener attention using a neural network vs. that using a linear decoder. Fourteen subjects gave informed consent and participated in a 28.7 minute experiment. Subjects listened to either a male or female talker (collocated) in a two-talker mixture for half the time, and then switched attention half way through the experiment. 64-channel electroencephalogram (EEG) data was collected from a wet electrode system, and the two decoders then transformed these signals into an approximation of the target speech’s temporal envelope. Results indicate that the neural-network-based, non-linear decoder outperforms the linear baseline in decoding accuracy for 9 out of 10 subjects for which both decoders’ accuracy was above chance. This experiment suggests that non-linear decoding methods may provide an avenue for achieving decoding performance required for a practical, attention-augmented hearing device.
- TasNet: Surpassing Ideal Time-Frequency Masking for Speech Separation
Yi Luo, Nima Mesgarani (Columbia University)Abstract- Robust speech processing in multitalker acoustic environments requires automatic speech separation. While single-channel, speaker-independent speech separation methods have recently seen great progress, the accuracy, latency, and computational cost of speech separation remain insufficient. The majority of the previous methods have formulated the separation problem through the time-frequency representation of the mixed signal, which has several drawbacks, including the decoupling of the phase and magnitude of the signal, the suboptimality of spectrogram representations for speech separation, and the long latency in calculating the spectrogram. To address these shortcomings, we propose the time-domain audio separation network (TasNet), which is a deep learning autoencoder framework for time-domain speech separation. TasNet uses a convolutional encoder to create a representation of the signal that is optimized for extracting individual speakers. Speaker extraction is achieved by applying a weighting function (mask) to the encoder output. The modified encoder representation is then inverted to the sound waveform using a linear decoder. The masks are found using a temporal convolutional network consisting of dilated convolutions, which allow the network to model the long-term dependencies of the speech signal. This end-to-end speech separation algorithm significantly outperforms previous time-frequency methods in terms of separating speakers in mixed audio, even when compared to the separation accuracy achieved with the ideal time-frequency mask of the speakers. In addition, TasNet has a smaller model size and a shorter minimum latency, making it a suitable solution for both offline and real-time speech separation applications. This study therefore represents a major step toward actualizing speech separation for real-world speech processing technologies.
- MaD TwinNet: Masker-Denoiser Architecture with Twin Networks for Monaural Sound Source Separation
Gerald Schuller (Technical University of Ilmenau / Fraunhofer IDMT)Abstract- Monaural singing voice separation task focuses on the prediction of the singing voice from a single channel music mixture signal. Current state of the art (SOTA) results in monaural singing voice separation are obtained with deep learning based methods. In this work we present a novel deep learning based method that learns long-term temporal patterns and structures of a musical piece. We build upon the recently proposed Masker-Denoiser (MaD) architecture and we enhance it with the Twin Networks, a technique to regularize a recurrent generative network using a backward running copy of the network. We evaluate our method using the Demixing Secret Dataset and we obtain an increment to signal-to-distortion ratio (SDR) of 0.37 dB and to signal-to-interference ratio (SIR) of 0.23 dB, compared to previous SOTA results.
- A Comparative Study of Convolutional and Recurrent Neural Network Architectures on Large-Scale Sound Event Classification
Emre Cakir, Dan Ellis (Google)Abstract- This work includes the performance comparison of depth-separable convolutional nets, LSTMs (and the combination of both), the effect of feedback refinement over weak labels and the analysis of recurrent layer activations.
- The Northwestern University Source Separation Library
Ethan Manilow, Prem Seetharaman, Bryan Pardo (Northwestern University )Abstract- Audio source separation is the process of isolating individ- ual sonic elements from a mixture or auditory scene. We present the Northwestern University Source Separation Library, or nussl for short. nussl (pronounced ‘nuzzle’) is an open-source, object-oriented audio source separation library implemented in Python. nussl provides imple- mentations for many existing source separation algorithms and a platform for creating the next generation of source separation algorithms. By nature of its design, nussl easily allows new algorithms to be benchmarked against existing algorithms on established data sets and facilitates development of new variations on algorithms. Here, we present the design methodologies in nussl, two experi- ments using it, and use nussl to showcase benchmarks for some algorithms contained within.
- Multi-resolution Common Fate Transform
Fatemeh Pishdadian, Bryan Pardo (Northwestern University )Abstract- The Multi-resolution Common Fate Transform (MCFT) is an audio signal representation useful for representing mixtures of multiple audio signals that overlap in both time and frequency. The MCFT combines the invertibility of a state-of-the-art representation, the Common Fate Transform (CFT), and the multi-resolution property of the cortical stage output of an auditory model. Since the MCFT is computed based on a fully invertible complex time-frequency representation, separation of audio sources with high time-frequency overlap may be performed directly in the MCFT domain, where there is less overlap between sources than in the time-frequency domain. The MCFT circumvents the resolution issue of the CFT by using a multi-resolution 2D filter bank instead of fixed-size 2D windows. Our experiments on highly overlapped audio mixtures show that the MCFT provide better separability than the commonly used time-frequency signal representations as well as the CFT.
- Vocal Imitation Set: a dataset of vocally imitated sound events using the AudioSet ontology
Bongjun Kim, Madhav Ghei, Bryan Pardo, Zhiyao Duan (Northwestern University, University of Rochester)Abstract- Query-By-Vocal Imitation (QBV) search systems enable searching a collection of audio files using a vocal imitation as a query. This can be useful when sounds do not have commonly agreed-upon text-labels, or many sounds share a label. As deep learning approaches have been successfully applied to QBV systems, datasets to build models have become more important. We present Vocal Imitation Set, a new vocal imitation dataset containing 11,242 crowd-sourced vocal imitations of 302 sound event classes in the AudioSet sound event ontology. It is the largest publicly-available dataset of vocal imitations as well as the first to adopt the widely-used AudioSet ontology for a vocal imitation dataset. Each imitation recording in Vocal Imitation Set was rated by a human listener on how similar the imitation is to the recording it was an imitation of. Vocal Imitation Set also has an average of 10 different original recordings per sound class. Since each sound class has about 19 listener-vetted imitations and 10 original sound files, the data set is suited for training models to do fine-grained vocal imitation-based search within sound classes. We provide an example of using the dataset to measure how well the existing state-of-the-art in QBV search performs on fine-grained search.
- Applying Triplet Loss to Siamese-Style Networks for Audio Similarity Ranking
Brian Margolis, Madhav Ghei, Bryan Pardo (Northwestern University)Abstract- Query by vocal imitation (QBV) systems let users search a library of general non-speech audio files using a vocal imitation of the desired sound as the query. The best existing system for QBV uses a similarity measure between vocal imitations and general audio files that is learned by a two-tower semi-Siamese deep neural network architecture. This approach typically uses pairwise training examples and error measurement. In this work, we show that this pairwise error signal does not correlate well with improved search rankings and instead describe how triplet loss can be used to train a two-tower network designed to work with pairwise loss, resulting in better correlation with search rankings. This approach can be used to train any two-tower architecture using triplet loss. Empirical results on a dataset of vocal imitations and general audio files show that low triplet loss is much better correlated with improved search ranking than low pairwise loss.
- Generating Albums with SampleRNN to Imitate Metal, Rock, and Punk Bands
CJ Carr, Zack Zukowski (Dadabots)Abstract- This early example of neural synthesis is a proof-of-concept for how machine learning can drive new types of music software. Creating music can be as simple as specifying a set of music influences on which a model trains. We demonstrate a method for generating albums that imitate bands in experimental music genres previously unrealized by traditional synthesis techniques (e.g. additive, subtractive, FM, granular, concatenative). Raw audio is generated autoregressively in the time-domain using an unconditional SampleRNN. We create six albums this way. Artwork and song titles are also generated using materials from the original artists’ back catalog as training data. We try a fully-automated method and a human-curated method. We discuss its potential for machine-assisted production.
- WaveNet Based Low Rate Speech Coding
W. Bastiaan Kleijn, Felicia S. C. Lim, Alejandro Luebs, Jan Skoglund, Florian Stimberg, Quan Wang, Thomas C. Walters (Google)Abstract- Traditional parametric coding of speech facilitates low rate but provides poor reconstruction quality because of the inadequacy of the model used. We describe how a WaveNet generative speech model can be used to generate high quality speech from the bit stream of a standard parametric coder operating at 2.4 kb/s.
- Multi-View Networks for Denoising of Arbitrary Numbers of Channels
Jonah Casebeer, Brian Luc, Paris Smaragdis (UIUC)Abstract- We propose a set of denoising neural networks capable of operating on an arbitrary number of channels at runtime, irrespective of how many channels they were trained on. We coin the proposed models multi-view networks since they operate using multiple views of the same data. We explore two such architectures and show how they outperform traditional denoising models in multi-channel scenarios. Additionally, we demonstrate how multi-view networks can leverage information provided by additional recordings to make better predictions, and how they are able to generalize to a number of recordings not seen in training.
- Acoustic speech analysis of patients with decompensated heart failure: a pilot study
Olivia Murton, Maureen Daher, Thomas Cunningham, Karla Verkouw, Sara Tabtabai, Johannes Steiner, Robert Hillman, G. William Dec, Dennis Ausiello, Daryush Mehta (Harvard Medical School, Massachusetts General Hospital)Abstract- Heart failure (HF) is a chronic condition characterized by impaired cardiac function, increased intracardiac filling pressures, and peripheral edema. HF can escalate into decompensation, requiring hospitalization. Patients with HF are typically monitored to prevent decompensation, but current methods are of only limited reliability (e.g., weight monitoring) or invasive and expensive (e.g., surgically implanted devices). This study investigated the ability of acoustic speech analysis to monitor patients with HF, since HF-related edema in the vocal folds and lungs was hypothesized to affect phonation and speech respiration. Ten patients with HF (8 male/2 female, mean age 70 years) undergoing inpatient treatment for decompensation performed a daily recording protocol of sustained vowels, read text, and spontaneous speech. Mean length of stay was 7 days, and average weight loss was 8.5 kg. Acoustic features extracted included fundamental frequency, cepstral peak prominence, automatically identified creaky voice segments, and breath group durations. After treatment, patients displayed increased fundamental frequency (mean change 3.7 Hz) , decreased cepstral peak prominence variation (mean change -0.41 dB), and a higher proportion of creaky voice in read passages (mean change 6.9 percentage points) and sentences (mean change 5.5 percentage points), suggesting that phonatory biomarkers may be early indicators of HF-related edema.
- Skeleton plays piano: online generation of pianist body movements from MIDI performances
Bochen Li, Akira Maezawa, Zhiyao Duan (University of Rochester, Yamaha)Abstract- Generating expressive body movements of a pianist for a given symbolic sequence of key depressions is important for music interaction, but most existing methods cannot incorporate musical context information and generate movements of body joints that are further away from the fingers such as head and shoulders. This paper addresses such limitations by directly training a deep neural network system to map a MIDI note stream and additional metric structures to a skeleton sequence of a pianist playing a keyboard instrument in an online fashion. Experiments show that (a) incorporation of metric information yields in 4% smaller error, (b) the model is capable of learning the motion behavior of a specific player, and (c) no significant difference between the generated and real human movements is observed by human subjects in 75% of the pieces.
- Bitwise Source Separation on Hashed Spectra: An Efficient Posterior Estimation Scheme Using Partial Rank Order Metrics
Lijiang Guo and Minje Kim (Indiana University)Abstract- This paper proposes an efficient bitwise solution to the single-channel source separation task. Most dictionary-based source separation algorithms rely on iterative update rules during the run time, which becomes computationally costly especially when we employ an overcomplete dictionary and sparse encoding that tend to give better separation results. To avoid such cost we propose a bitwise scheme on hashed spectra that leads to an efficient posterior probability calculation. For each source, the algorithm uses a partial rank order metric to extract robust features that form a binarized dictionary of hashed spectra. Then, for a mixture spectrum, its hash code is compared with each source's hashed dictionary in one pass. This simple voting-based dictionary search allows a fast and iteration-free estimation of ratio masking at each bin of a signal spectrogram. We verify that the proposed BitWise Source Separation (BWSS) algorithm produces sensible source separation results for the single-channel speech denoising task, with 6-8 dB mean SDR improvement. To our knowledge, this is the first dictionary based algorithm for this task that is completely iteration-free in both training and testing.
- Hierarchical Multitask Learning With CTC
Ramon Sanabria, Florian Metze (CMU)Abstract- In Automatic Speech Recognition, it is still challenging to learn useful intermediate representations when using high-level (or abstract) target units such as words. For that reason, when only a few hundreds of hours of training data are available, character or phoneme-based systems tend to outperform word-based systems. In this paper, we show how Hierarchical Multitask Learning can encourage the formation of useful intermediate representations. We achieve this by performing Connectionist Temporal Classification at different levels of the network with targets of different granularity. Our model thus performs predictions in multiple scales for the same input. On the standard 300h Switchboard training setup, our hierarchical multitask architecture demonstrates improvements over single-task architectures with the same number of parameters. Our model obtains 14.0% Word Error Rate on the Switchboard subset of the Eval2000 test set without any decoder or language model, outperforming the current state-of-the-art on non-autoregressive Acoustic-to-Word models.
- BirdVox-Imitation: A dataset of human imitations of birdsong with potential for research in psychology and machine listening
Kendra Oudyk, Vincent Lostanlen, Justin Salamon, Andrew Farnsworth, and Juan Pablo Bello (McGill, Cornell, NYU)Abstract- Bird watchers imitate bird sounds in order to elicit vocal responses from birds in the forest, and thus locate them. Field guides offer various strategies for learning birdsong, from visualizing spectrograms to memorizing onomatopoeic sounds such as "fee bee". However, imitating birds can be challenging for humans because we have a different vocal apparatus. Many birds can sing at higher pitches and over a wider range of pitches than humans. In addition, they can alternate between notes more rapidly and some produce complex timbres. Little is known about how humans spontaneously imitate birdsong, and the imitations themselves pose an interesting problem for machine listening. In order to facilitate research into these areas, here we present BirdVox-Imitation, an audio dataset on human imitations of birdsong. This dataset includes audio of a) 1700 imitations from 17 participants who each performed 10 imitations of 10 bird species; b) 100 birdsong stimuli that elicited the imitations, and c) over 6500 excerpts of birdsong, from which the stimuli were selected. These excerpts are ‘clean’ 3-10 second excerpts that were manually annotated and segmented from field recordings of birdsong. The original recordings were scraped from xeno-canto.org: an online, open access, crowdsourced repository of bird sounds. This dataset holds potential for research in both psychology and machine learning. In psychology, questions could be asked about how humans imitate birds – for example, about how humans imitate the pitch, timing, and timbre of birdsong, about when they use different imitation strategies (e.g., humming, whistling, and singing), and about the role of individual differences in musical training and bird-watching experience. In machine learning, this may be the first dataset that is both multimodal (human versus bird) and domain-adversarial (wherein domain refers to imitation strategy, such as whistling vs. humming), so there is plenty of opportunity for developing new methods. This dataset will soon be released on Zenodo to facilitate research in these novel areas of investigation.
- Efficient Training and Labeling with Large and Unlabeled Dataset Using Active Learning
Yu Wang, Ana Elisa Mendez Mendez, and Juan Pablo Bello (NYU)Abstract- In the Sounds of New York City (SONYC) project, we have been collecting urban sound recordings from acoustic sensors deployed in multiple locations around New York City to monitor noise pollution. This dataset is huge, unlabeled and grows continuously. A type of faulty signal was detected (in the form of interference noise) and has to be correctly identified within the dataset. Training a classifier with a large unlabeled and unbalanced dataset can be challenging and require a huge amount of human resources for annotation. In this study, we propose an active learning (AL) approach to solve this issue. We train a binary classifier using AL on the SONYC dataset with four different querying strategies. The optimal F-measure of 0.96 is achieved with only 92 labeled data points, while the baseline model trained using random sampling has F-measure of 0.88 with the same amount of labeled data. The examples queried during the AL training process have 47% positive examples, while random sampling returns 8% positive examples, which approximately represents the original data distribution. The results indicate that AL can be a good tool for a much more efficient training and labeling process with the least possible human resources, which is crucial when dealing with continuously growing unlabeled datasets.
- Neural Music Synthesis
Jong Wook Kim, Rachel Bittner, Aparna Kumar, Juan Pablo Bello (NYU, Spotify)Abstract- The recent successes of end-to-end audio synthesis models like WaveNet motivate a new approach for music synthesis, in which the entire process --- creating audio samples from a score and instrument information --- is modeled using generative neural networks. This poster describes an end-to-end audio synthesis model which consists of a recurrent neural network conditioned on a learned instrument embedding followed by a WaveNet vocoder. The learned embedding space successfully captures the diverse variations in timbres within a large dataset and enables timbre morphing by interpolating between instruments in the embedding space. The synthesis quality is evaluated both numerically and perceptually, and an interactive demo will be provided on a laptop.
- Single channel speech enhancement using neural networks
Stepan Sargsyan (2Hz.ai)Abstract- We propose a method of single channel speech enhancement using Neural Networks as a mapping function between noisy and clean speech signals. In order to improve mapping accuracy we have tried various types of Neural Networks and custom architectures. To get more generalized model we collected training data consisting of several thousand hours of noisy speech recordings and their clean versions. Our specially designed Net optimizes itself to recognize human speech and enhances it by suppressing the background noise. It should be noted that usually there is a trade-off between audio processing time and speech enhancement accuracy. Nevertheless, our technology is capable of enhancing speech with high level of accuracy in real time, without noticeable latency.
- Fixing Voice Breakups with Deep Learning
Karen Yeressian (2Hz.ai)Abstract- In communicating audio signals over the internet the lost data packets result in significant degradation of the audio quality. The task of a packet loss concealment algorithm is to fill in these gaps appropriately so that to regain the quality. There are well developed algorithms, such as the packet loss concealment algorithm integrated in the Opus codec, which works well for low rates of packet loss. To achieve a good performance, specially for higher packet loss rates, we have considered various approaches using deep neural networks. We extract appropriate features from available audio frames and feed these into our networks. The network produces the appropriate features for the lost audio frames. We have considered both the cases when one has access to the original available frames and when one only has the output of another packet loss concealment algorithm, for example that of the Opus codec. In both cases we obtain considerable improvement of the audio quality.