SANE 2022 - Speech and Audio in the Northeast
October 6, 2022
The workshop is now over. Videos and slides for the talks are available through the links in the schedule below. There is also a YouTube Playlist for all talks.
SANE 2022, a one-day event gathering researchers and students in speech and audio from the Northeast of the American continent, was held on Thursday October 6, 2022 in Kendall Square, in Cambridge, MA.
It was the 9th edition in the SANE series of workshops, which started in 2012 and was held every year alternately in Boston and New York until 2019. After a 2-year hiatus due to the pandemic, we were happy to welcome the return of SANE, with 145 participants and 29 posters this year.
SANE 2022 featured invited talks by leading researchers from the Northeast as well as from the wider community. It also featured a lively poster session, open to both students and researchers.
Details
- Date: Thursday, October 6, 2022
- Venue: 314 Main Street, Cambridge, MA (above the Kendall Square T Station)
- Time: 8:30am - 6:10pm.
Schedule [Watch all recorded talks on YouTube]
Click on the talk title to jump to the abstract and bio.Registration
The workshop is now over. If you are interested in attending future SANE events, please sign up to the SANE News mailing list.
Directions
The workshop was hosted at 314 Main St, in Cambridge, MA, right above the Kendall Square Station on the T Red Line.
Organizing Committee
- Jonathan Le Roux (MERL)
- Arnab Ghoshal (Apple)
- John R. Hershey (Google)
- Shinji Watanabe (CMU)
Sponsors
SANE remains a free workshop thanks to the generous contributions of the sponsors below, and to the partnership of the Language Technologies Institute at Carnegie Mellon University as host organization.
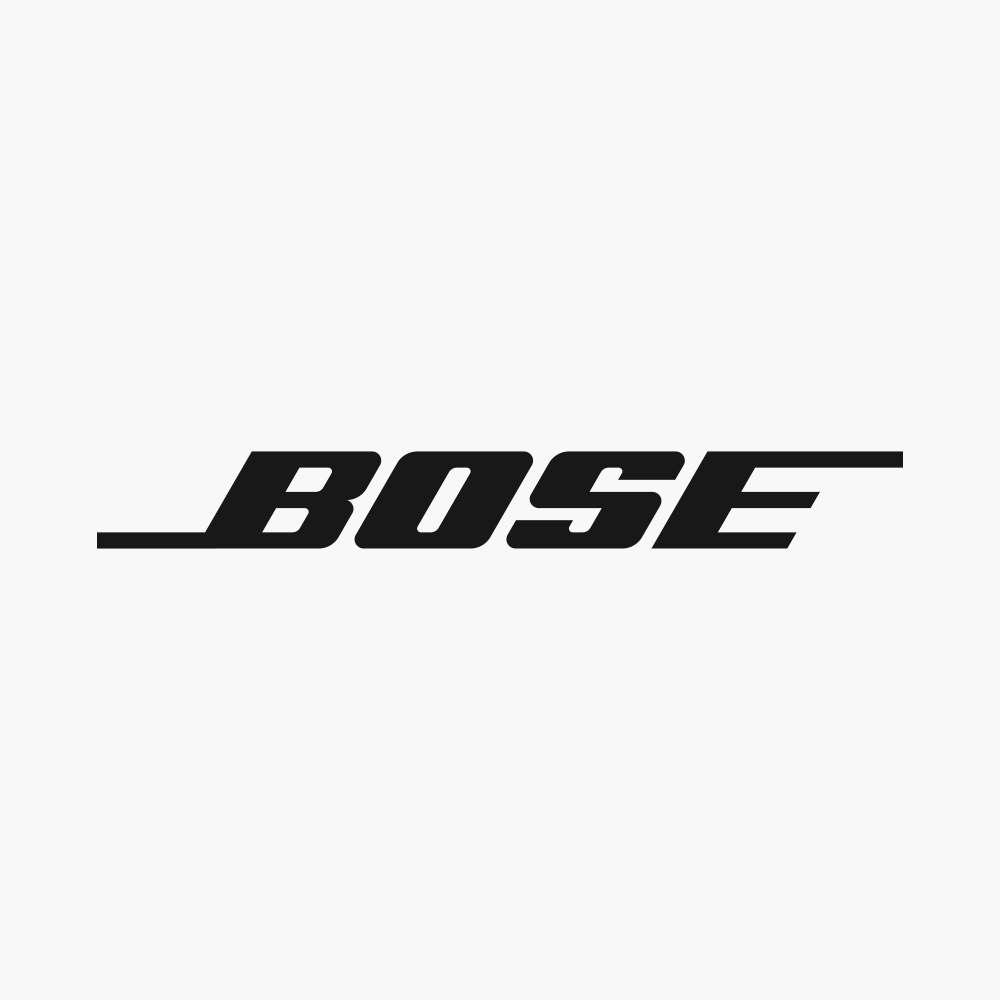
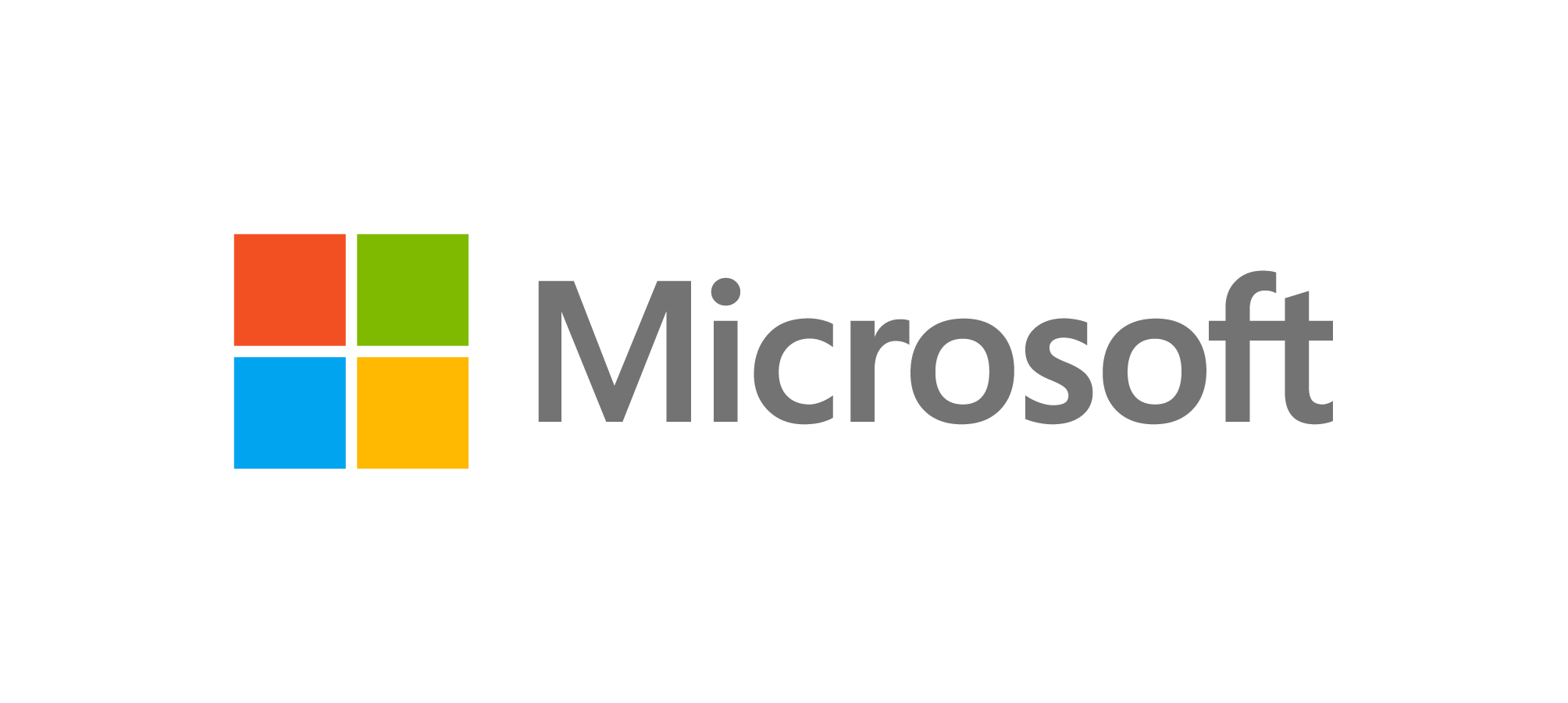
Talks
Beyond end-to-end speech synthesis: What have we created and how do we protect its misuse?
Within the past decade, advances in machine learning coupled with the ubiquity of conversational devices such as the Amazon Echo and Google Home have created the perfect storm to revitalize the field of speech synthesis. Suddenly, it wasn’t enough to have a handful of voices that took years to build and millions of dollars to bring to market. Today, all the big tech giants have reinvested in speech synthesis and a dozen+ startups are fast following by building upon open source repositories and in many cases even advancing the science using real world data. Text-to-speech and speech-to-speech methods are both in demand and achieving human-like quality is commonplace, especially for short form audio. The new goal post to shoot for is emotional, dramatic and performative quality speech. At the same time, listeners are becoming more concerned about synthetic media and its role in misinformation. This talk explores the next big problems to solve in this new dawn of synthetic media. How do we ensure that the very advances that enable those with speech disabilities to speak in an AI voice that conveys their identity or millions of non-literate people to access content in their local language, doesn’t feed the hungry mouths of disinformation?
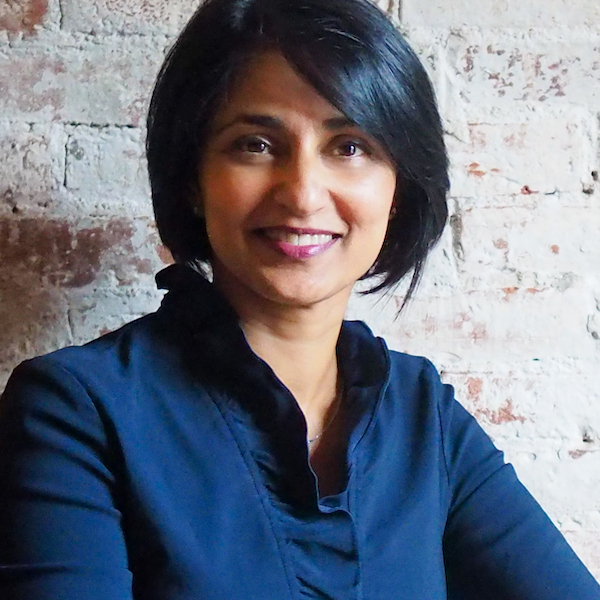
Rupal Patel Dr. Rupal Patel is an internationally renowned speech scientist turned entrepreneur, bringing decades of clinical, academic, scientific and social entrepreneurship experience to Veritone. As Vice President of Voice & Accessibility, Rupal leads strategy and innovation efforts in the use of voice AI for media and entertainment, in addition to expanding the reach and impact of Veritone’s voice solutions for those living with disabilities or inequities. A preeminent thought leader in voice AI, Dr. Patel advocates for ethical transparent and fair use policies that can broaden the monetization capabilities for voice-over artists by leveraging AI. Prior to Veritone, Dr. Patel was the Founder and CEO of VocaliD, a voice AI company acquired by Veritone in 2022, that creates synthetic voices with personality for discerning brands that understand the power of customized voice and for individuals living with speechlessness who want to be heard in a voice that is uniquely theirs. VocaliD was a spinout from Dr. Rupal’s research lab at Northeastern University, where she is a tenured Full Professor with interdisciplinary appointments in the Bouve College of Health Science and Khoury College of Computer Science. Named one of Fast Company’s 100 Most Creative People in Business, Rupal has been featured on TED, NPR, WIRED, and in major international news and technology publications.
Self-Supervised Learning for Speech Generation
Self-supervised learning (SSL) for speech has demonstrated great success on inference tasks such as speech recognition. However, it is less studied for generative tasks where the goal is to synthesize speech. In this talk, I will share our recent work on building unconditional and conditional generative speech models leveraging SSL. Instead of representing speech with traditional features like spectrogram, we showed that discrete units derived from self-supervised models serve as better generative modeling targets for several tasks. Specifically, we presented the first text-free spoken language models for prosodically rich speech as well as spoken dialogues, and achieved SOTA performance on speech-to-speech translation without intermediate text output.
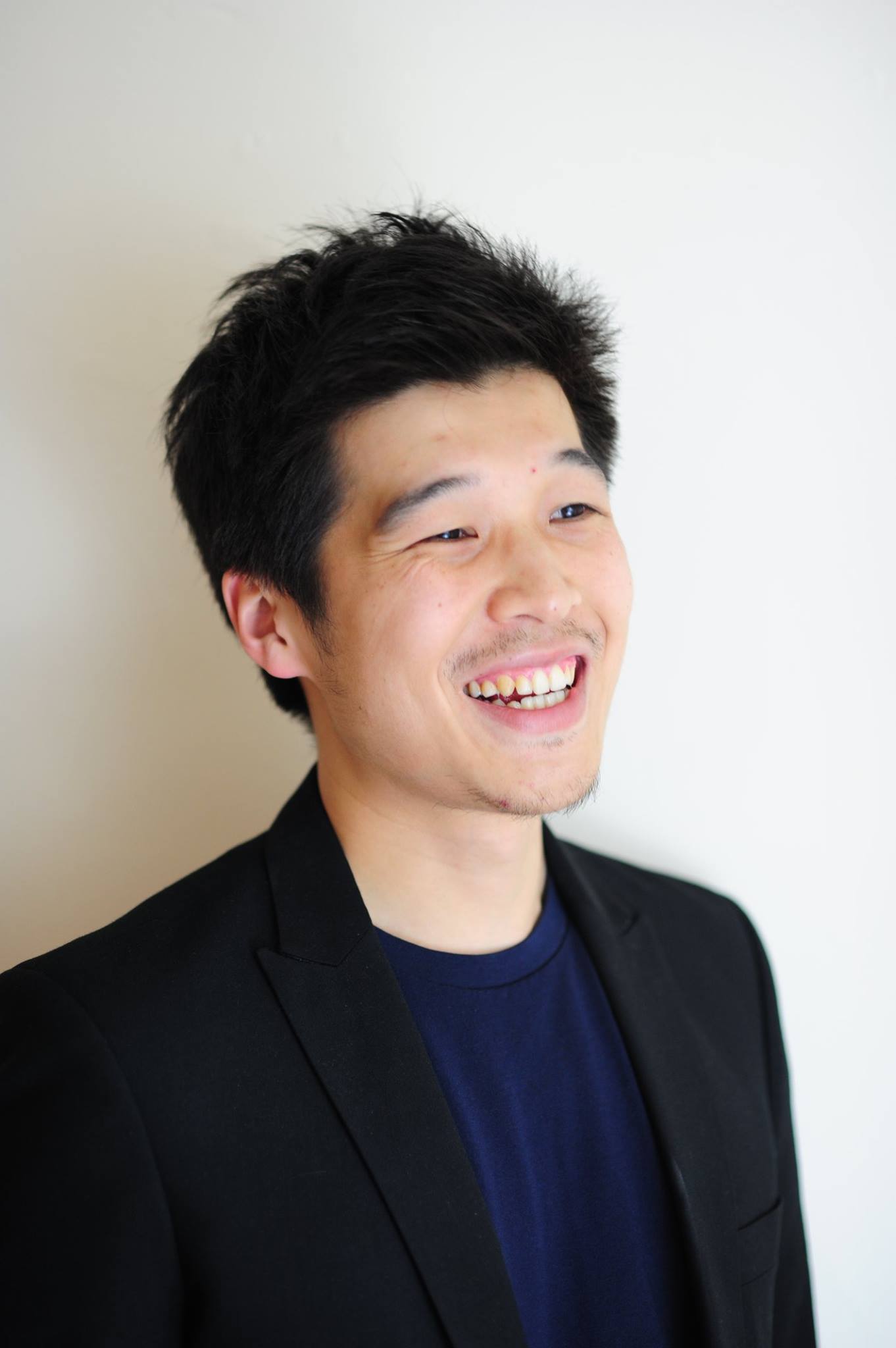
Wei-Ning Hsu Wei-Ning Hsu is a research scientist at Meta Fundamental AI Research (FAIR). His research focuses on representation learning, self-supervised learning, and structured generative modeling for unimodal and multimodal speech. He is passionate about reducing the supervision required for building various speech applications and developing technologies applicable to both written and unwritten languages. He has published over 40 peer-reviewed papers in ICLR, NeurIPS, ICML, Interspeech, ICASSP, TASLP, ACL, EMNLP, TACL. His recent work includes AV-HuBERT, data2vec, wav2vec-U, Textless NLP, textless speech-to-speech translation, and HuBERT. Prior to joining Facebook, Wei-Ning received his Ph.D. and S.M. degrees in Electrical Engineering and Computer Science from Massachusetts Institute of Technology in 2020 and 2018, under the supervision of Dr. James Glass. He received his B.S. degree in Electrical Engineering from National Taiwan University in 2014, under the supervision of Prof. Lin-shan Lee and Prof. Hsuan-Tien Lin. More information can be found at https://wnhsu.github.io/
Unsupervised Sound Separation: A New Paradigm for Audio Processing
Historically, sound separation models have been trained using synthetic mixtures of isolated reference signals. This supervised approach generally works well when matched isolated data is abundant, but for many domains such isolated data is not available, and it is difficult to simulate real conditions. I will describe our recent breakthrough in unsupervised sound separation, mixture invariant training (MixIT), which allows neural networks to learn to separate audio mixtures into their constituent sources without requiring isolated reference signals. Unsupervised sound separation is a potent new paradigm for audio processing, enabling a number of new directions. These include scaling up training data for universal sound separation, improved bird species classification by separating birdsong, adapting separation models to real-world meeting data, and a state-of-the-art audio-visual on-screen separation model called AudioScope, which can isolate the sounds of visible objects in a video regardless of their class. I will discuss our experiments using MixIT for these directions and describe several exciting avenues for future research.
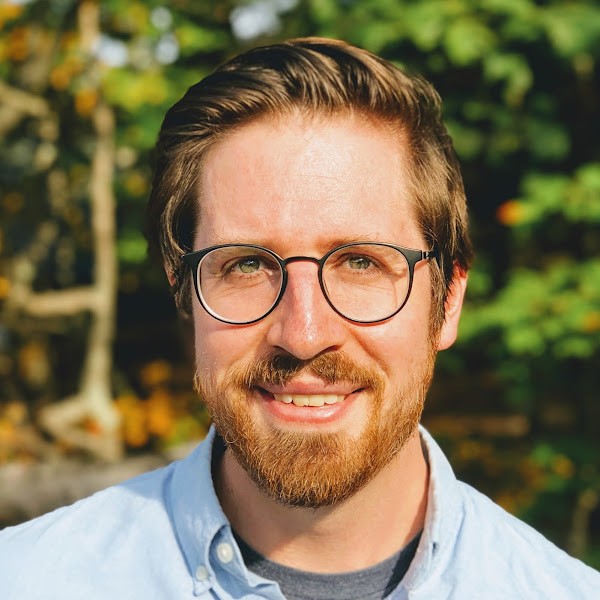
Scott Wisdom Scott Wisdom is a senior research scientist at Google in Cambridge, MA working on speech, audio, and audio-visual machine perception, with a focus on audio source separation. Scott completed his Ph.D. in electrical engineering from the University of Washington in Seattle in 2017. During this time, Scott also spent time as an intern at MERL and Microsoft Research. He serves as a member of the IEEE Signal Processing Society Technical Committee on Audio and Acoustic Signal Processing and was an area chair for ICASSP 2021, ICASSP 2022, and WASPAA 2021. He and his collaborators were recognized with a best student paper award at WASPAA 2017, and two best paper awards at WASPAA 2021.
End-to-End Speech Recognition: The Journey from Research to Production
End-to-end (E2E) speech recognition has become a popular research paradigm in recent years, allowing the modular components of a conventional speech recognition system (acoustic model, pronunciation model, language model), to be replaced by one neural network. In this talk, we will discuss a multi-year research journey of E2E modeling for speech recognition at Google. This journey has resulted in E2E models that can surpass the performance of conventional models across many different quality and latency metrics, as well as the productionization of E2E models for Pixel 4, 5 and 6 phones. We will also touch upon future research efforts with E2E models, including multi-lingual speech recognition.
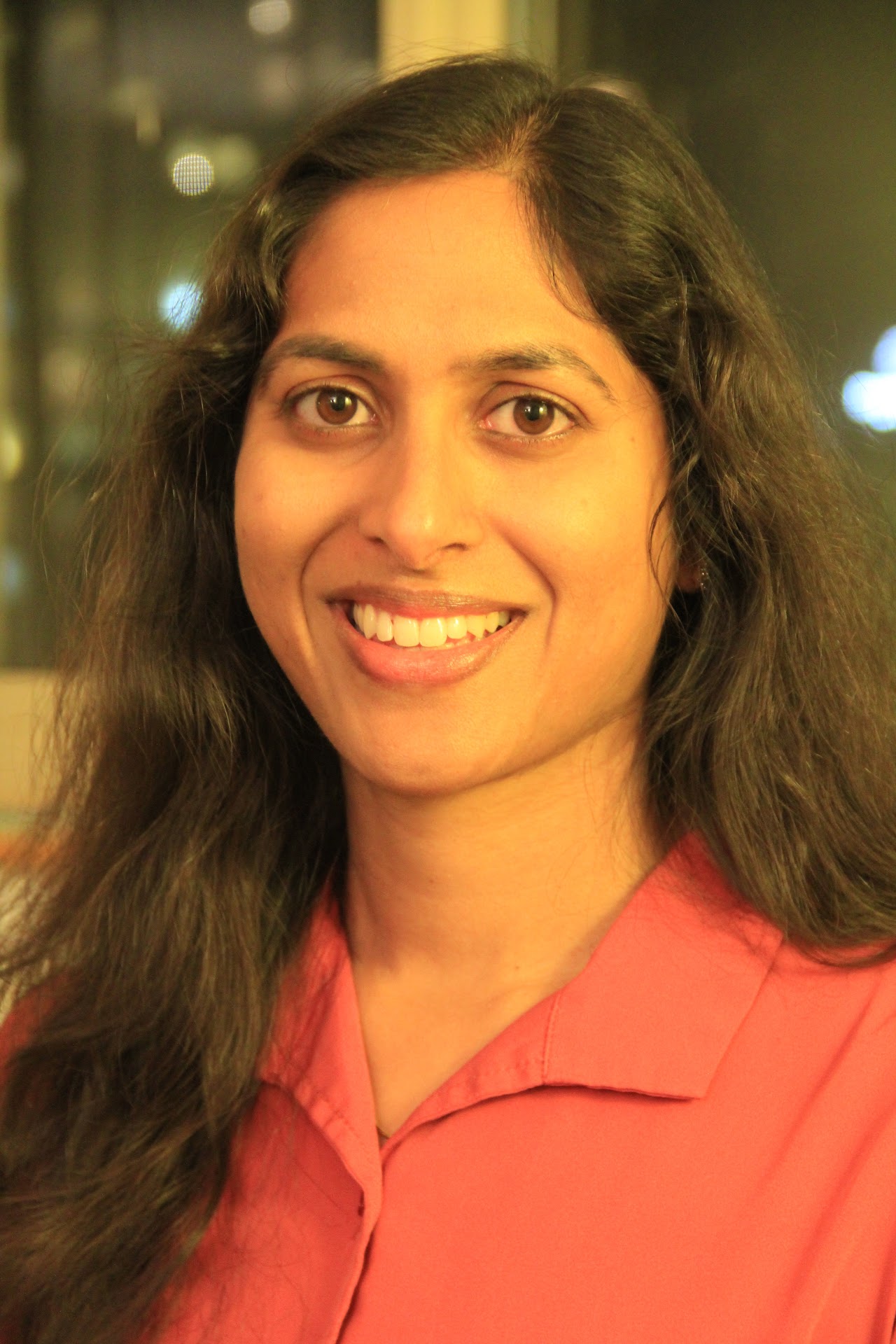
Tara Sainath Tara Sainath received her S.B., M.Eng and PhD in Electrical Engineering and Computer Science (EECS) from MIT. After her PhD, she spent 5 years at the Speech and Language Algorithms group at IBM T.J. Watson Research Center, before joining Google Research. She has served as a Program Chair for ICLR in 2017 and 2018. Also, she has co-organized numerous special sessions and workshops, including Interspeech 2010, ICML 2013, Interspeech 2016, ICML 2017, Interspeech 2019, NeurIPS 2020. In addition, she has served as a member of the IEEE Speech and Language Processing Technical Committee (SLTC) as well as the Associate Editor for IEEE/ACM Transactions on Audio, Speech, and Language Processing. She is an IEEE and ISCA Fellow and the recipient of the 2021 IEEE SPS Industrial Innovation Award. She is currently a Principal Research Scientist at Google, working on applications of deep neural networks for automatic speech recognition.
Explainable End-to-End Neural Networks for Far-Field Conversation Recognition
This presentation introduces some of our group’s attempts at building an end-to-end network that integrates various speech processing modules into a single neural network while maintaining explainability. We will focus on far-field conversation recognition as an example and show how to unify automatic speech recognition, denoising, dereverberation, separation, and localization. We will also introduce our latest techniques for combining self-supervised learning, careful pre-training/fine-tuning strategies, and multi-task learning within our integrated network. This work achieved the best performance reported in the literature on several noisy reverberant speech recognition benchmarks, reaching the clean speech recognition performance.
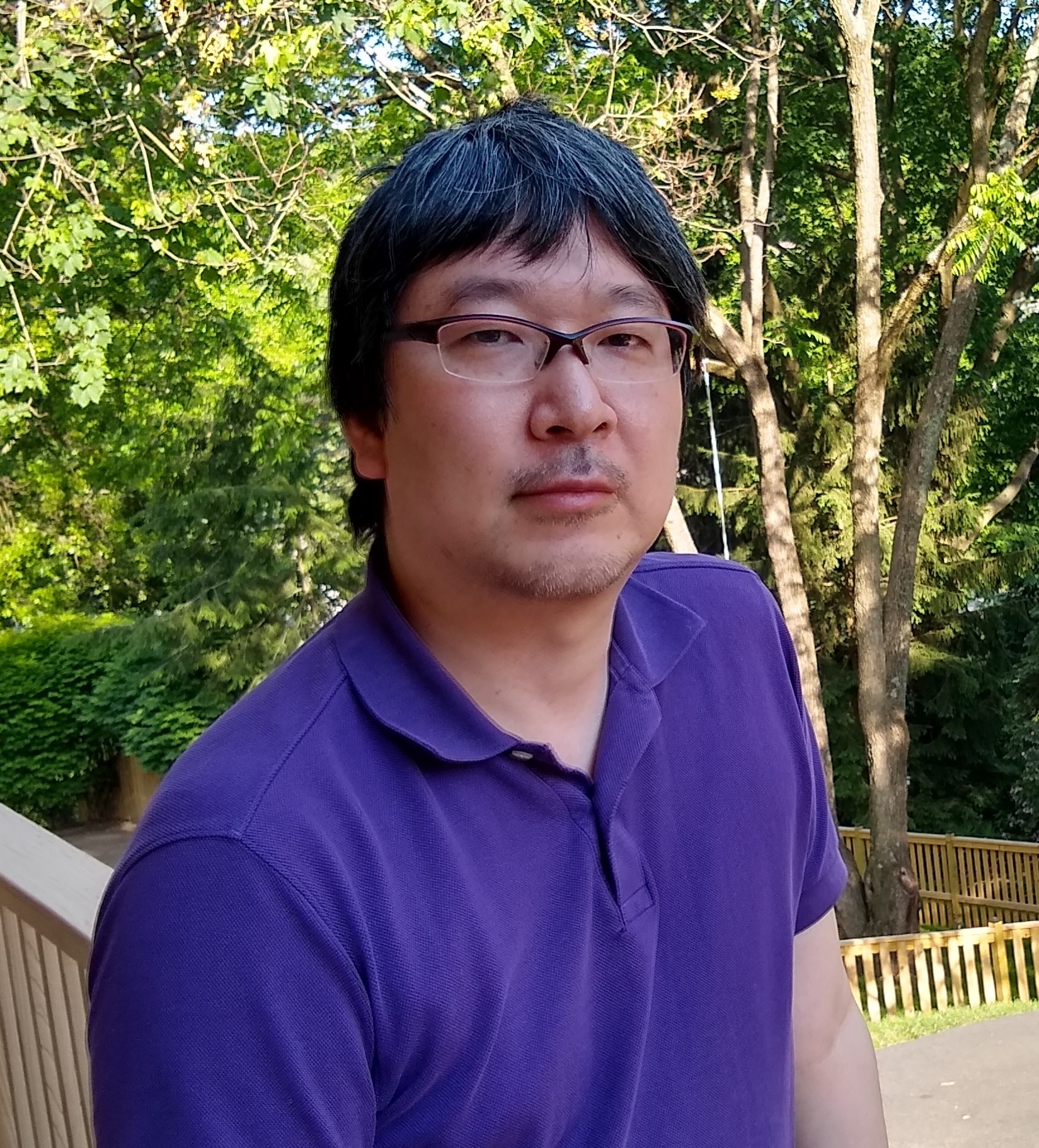
Shinji Watanabe Shinji Watanabe is an Associate Professor at Carnegie Mellon University, Pittsburgh, PA. He received his B.S., M.S., and Ph.D. (Dr. Eng.) degrees from Waseda University, Tokyo, Japan. He was a research scientist at NTT Communication Science Laboratories, Kyoto, Japan, from 2001 to 2011, a visiting scholar at Georgia institute of technology, Atlanta, GA, in 2009, and a senior principal research scientist at Mitsubishi Electric Research Laboratories (MERL), Cambridge, MA USA from 2012 to 2017. Prior to the move to Carnegie Mellon University, he was an associate research professor at Johns Hopkins University, Baltimore, MD, USA, from 2017 to 2020. His research interests include automatic speech recognition, speech enhancement, spoken language understanding, and machine learning for speech and language processing. He has published more than 300 papers in peer-reviewed journals and conferences and received several awards, including the best paper award from the IEEE ASRU in 2019. He serves as a Senior Area Editor of the IEEE Transactions on Audio Speech and Language Processing. He was/has been a member of several technical committees, including the APSIPA Speech, Language, and Audio Technical Committee (SLA), IEEE Signal Processing Society Speech and Language Technical Committee (SLTC), and Machine Learning for Signal Processing Technical Committee (MLSP).
Neural Scene Representations for Multimodal Machine Intelligence
The world around us is rich with complex information stretched across a myriad of modalities (such as vision, audio, and text, among many others) and to solve real-world tasks, an intelligent agent must possess the ability to abstract this information complexity via suitable data representations that allow for a seamless assimilation of distributed knowledge. Central to such a multimodal integration is the important question of what constitutes good data representations that may induce a natural emergence of intelligent behavior. In this talk, I will describe how visual scene graphs offer a compelling choice for multimodal alignment. A scene graph is essentially a graph data structure that decomposes a visual scene into neural representations of objects and their relationships. When backed by the power of graph neural networks and Transformers, such graphs can be adapted to solve a wide variety of multimodal reasoning problems. I will present our recent research in this direction that combines vision, audio, language, and 3D geometry using scene graphs for solving problems such as audio-visual scene aware dialog, visually-guided audio source separation, and visual commonsense reasoning. I will also touch upon our recent efforts towards integrating multimodal reasoning with embodied AI for solving interactive audio-visual navigation tasks. Experiments on benchmark datasets demonstrate that our approaches lead to state-of-the-art results.
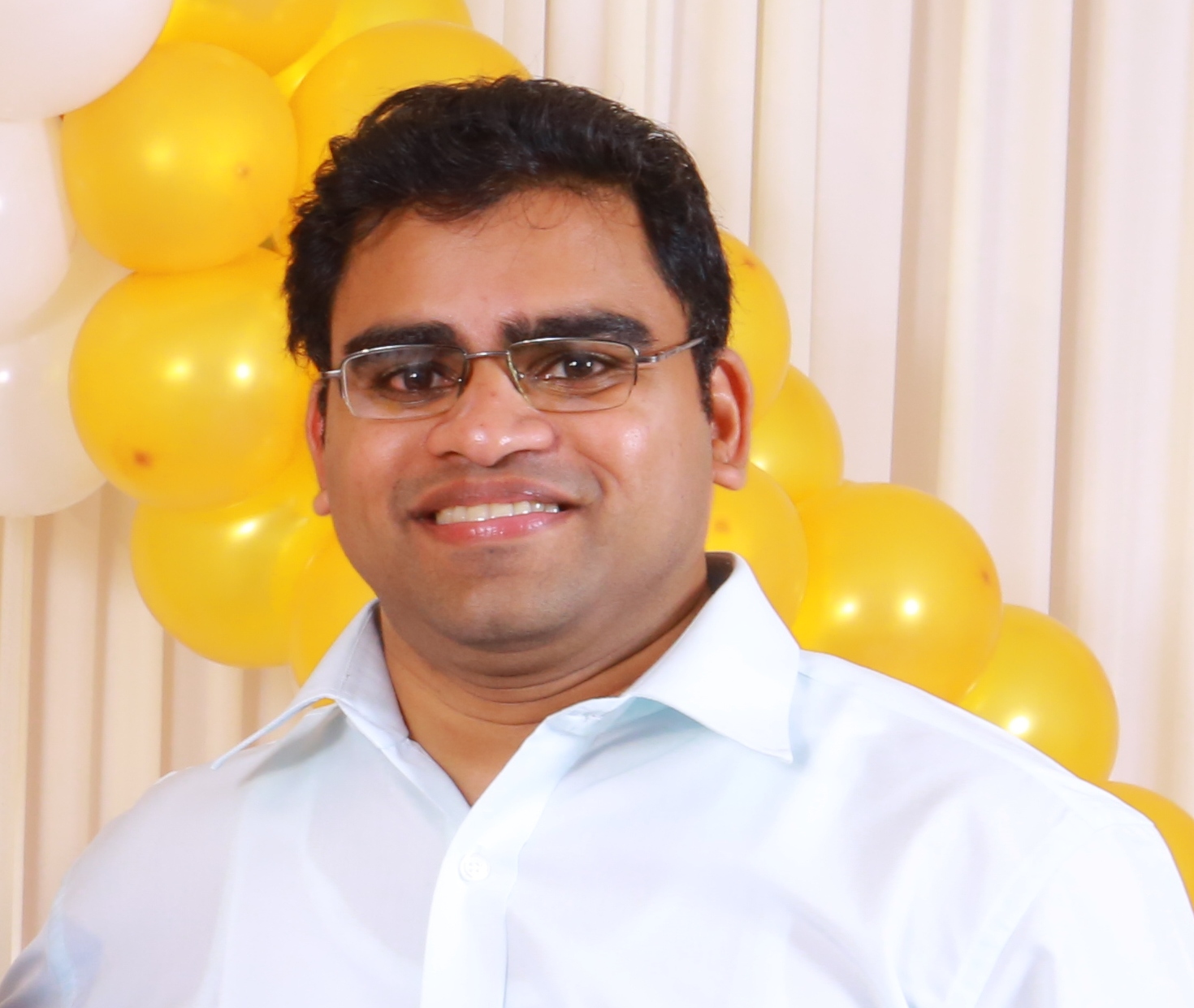
Anoop Cherian Anoop Cherian is a Principal Research Scientist with Mitsubishi Electric Research Labs (MERL), Cambridge, MA and an Adjunct Research Fellow with the Australian National University. He received his M.S. and Ph.D. degrees in computer science from the University of Minnesota, Minneapolis in 2010 and 2013, respectively. He was a postdoctoral researcher in the LEAR group at Inria, Grenoble from 2012-2015, and a Research Fellow at the Australian National University from 2015-2017. He is a recipient of the Best Student Paper Award at ICIP, 2012, MERL President's Award in 2020, among others, and several outstanding reviewer awards at top-tier computer vision and machine learning conferences. Anoop has broad interests in machine learning and computer vision, with a recent focus in topics related to neuro-symbolic scene representations and multimodal learning.
Learning to Perceive Physical Scenes from Multi-Sensory Data
UMass Amherst/MIT-IBM Watson AI Lab
Human sensory perception of the physical world is rich and multimodal and can flexibly integrate input from all five sensory modalities -- vision, touch, smell, hearing, and taste. However, in AI, attention has primarily focused on visual perception. In this talk, I will introduce my efforts in connecting vision with sound, allowing machine perception systems to see objects and infer physics from multi-sensory data. In the first part of my talk, I will discuss a self-supervised approach that could learn to parse images and separate the sound sources by watching and listening to unlabeled videos without requiring additional manual supervision. In the second part of my talk, I will introduce Neural Acoustic Fields (NAFs), an implicit representation that captures how sounds propagate in a physical scene and infer the underlying causal structure in 3D environments through visual and auditory observations. Finally, I will talk about how we may further build embodied agents to seek the sound source of repeating environmental sound (e.g., alarm) or identify what object has fallen and where from an intermittent impact sound.
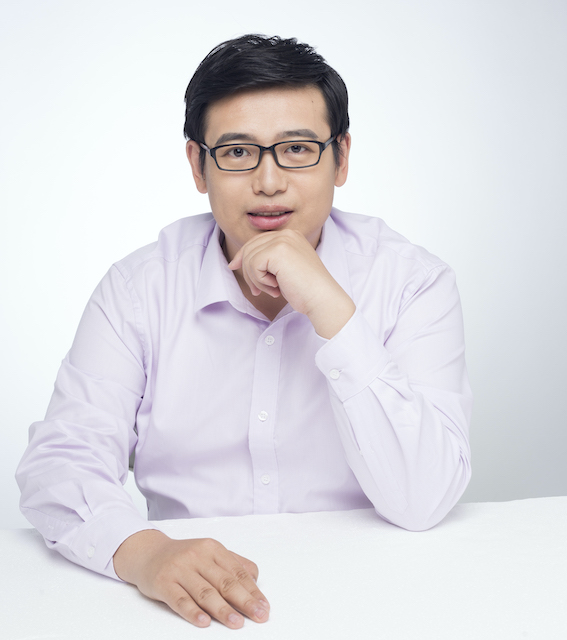
Chuang Gan Chuang Gan is an assistant professor at UMass Amherst and research manager at MIT-IBM Watson AI Lab. Before that, he was a researcher at MIT, working with Prof. Antonio Torralba and Prof. Josh Tenenbaum. He completed his Ph.D. with the highest honor at Tsinghua University, supervised by Prof. Andrew Chi-Chih Yao. His research interests sit at the intersection of computer vision, machine learning, and cognitive science. His research works have been recognized by Microsoft Fellowship, Baidu Fellowship, and media coverage from BBC, WIRED, Forbes, and MIT Tech Review. He has been an area chair of CVPR, ICCV, ECCV, ICML, ICLR, NeurIPS, and ACL and an associate editor of IEEE Transactions on Image Processing.
Posters
Instructions for posters: the poster boards are 32"x40", and can be placed in portrait or landscape orientation.
- 1. Meta-Learning for Adaptive Filters with Higher-Order Frequency Dependencies
Junkai Wu, Jonah Casebeer, Nicholas J. Bryan, Paris Smaragdis (UIUC)Abstract- Adaptive filters are applicable to many signal processing tasks including acoustic echo cancellation, beamforming, and more. Adaptive filters are typically controlled using al- gorithms such as least-mean squares (LMS), recursive least squares (RLS), or Kalman filter updates. Such models are often applied in the frequency domain, assume frequency independent processing, and do not exploit higher-order frequency dependencies, for simplicity. Recent work on meta-adaptive filters, however, has shown that we can con- trol filter adaptation using neural networks without manual derivation, motivating new work to exploit such information. In this work, we present higher-order meta-adaptive filters, a key improvement to meta-adaptive filters that incorporates higher-order frequency dependencies. We demonstrate our approach on acoustic echo cancellation and develop a family of filters that yield multi-dB improvements over competitive baselines, and are at least an order-of-magnitude less complex. Moreover, we show our improvements hold with or without a downstream speech enhancer.
- 2. Meta-AF: Meta-Learning for Adaptive Filters
Jonah Casebeer, Nicholas J. Bryan, Paris Smaragdis (UIUC, Adobe Research)Abstract- Adaptive filtering algorithms are pervasive throughout modern society and have had a significant impact on a wide variety of domains including audio processing, telecommunications, biomedical sensing, astrophysics and cosmology, seismology, and many more. Adaptive filters typically operate via specialized online, iterative optimization methods such as least-mean squares or recursive least squares and aim to process signals in unknown or nonstationary environments. Such algorithms, however, can be slow and laborious to develop, require domain expertise to create, and necessitate mathematical insight for improvement. In this work, we seek to go beyond the limits of human-derived adaptive filter algorithms and present a comprehensive framework for learning online, adaptive signal processing algorithms or update rules directly from data. To do so, we frame the development of adaptive filters as a meta-learning problem in the context of deep learning and use a form of self-supervision to learn online iterative update rules for adaptive filters. To demonstrate our approach, we focus on audio applications and systematically develop meta-learned adaptive filters for five canonical audio problems including system identification, acoustic echo cancellation, blind equalization, multi-channel dereverberation, and beamforming. We compare our approach against common baselines and/or recent state-of-the-art methods. We show we can learn high-performing adaptive filters that operate in real-time and, in most cases, significantly outperform each method and task we compare against -- all using a single general-purpose configuration of our method.
- 3. Efficient Personalized Speech Enhancement through Self-Supervised Learning
Aswin Sivaraman and Minje Kim (Indiana University)Abstract- This work presents self-supervised learning methods for developing monaural speaker-specific (i.e., personalized) speech enhancement models. While generalist models must broadly address many speakers, specialist models can adapt their enhancement function towards a particular speaker’s voice, expecting to solve a narrower problem. Hence, specialists are capable of achieving more optimal performance in addition to reducing computational complexity. However, naive personalization methods can require clean speech from the target user, which is inconvenient to acquire, e.g., due to subpar recording conditions. To this end, we pose personalization as either a zero-shot task, in which no additional clean speech of the target speaker is used for training, or a few-shot learning task, in which the goal is to minimize the duration of the clean speech used for transfer learning. With this paper, we propose self-supervised learning methods as a solution to both zero- and few-shot personalization tasks. The proposed methods are designed to learn the personalized speech features from unlabeled data (i.e., in-the-wild noisy recordings from the target user) without knowing the corresponding clean sources. Our experiments investigate three different self-supervised learning mechanisms. We set up a pseudo speech enhancement problem as a pretext task, which pretrains the models to estimate noisy speech as if it were the clean target. Contrastive learning and data purification methods regularize the loss function of the pseudo enhancement problem, overcoming the limitations of learning from unlabeled data. We assess our methods by personalizing the well-known ConvTasNet architecture to twenty different target speakers. The results show that self-supervised models achieve zero-shot and few-shot personalization using fewer model parameters and less clean data from the target user, achieving the data efficiency and model compression goals.
- 4. Differentiable World Synthesizer-Based Neural Vocoder With Application To End-To-End Audio Style Transfer
Shahan Nercessian (iZotope, Inc.)Abstract- We propose a differentiable WORLD synthesizer and demonstrate its use in end-to-end audio style transfer tasks such as (singing) voice conversion and the DDSP timbre transfer task. Accord- ingly, our baseline differentiable synthesizer has no model parameters, yet it yields adequate synthesis quality. We can extend the baseline synthesizer by appending lightweight black-box postnets which ap- ply further processing to the baseline output in order to improve fidelity. An alternative differentiable approach considers extraction of the source excitation spectrum directly, which can improve naturalness albeit for a narrower class of style transfer applications. The acoustic feature parameterization used by our approaches has the added benefit that it naturally disentangles pitch and timbral information so that they can be modeled separately. Moreover, as there exists a robust means of estimating these acoustic features from monophonic audio sources, it allows for parameter loss terms to be added to an end-to-end objective function, which can help convergence and/or further stabilize (adversarial) training.
- 5. Time-varying filter stability and state-matrix products
Kurt James Werner, Russell McClellan (iZotope, Inc.)Abstract- We show a new sufficient criterion for time-varying digital filter stability: that the matrix norm of the product of state matrices over a certain finite number of time steps is bounded by 1. This extends Laroche’s Criterion 1, which only considered one time step, while hinting at extensions to two time steps. Further extending these results, we also show that there is no intrinsic requirement that filter coefficients be frozen over any time scale, and extend to any dimension a helpful theorem that allows us to avoid explicitly performing eigen- or singular value decompositions in studying the matrix norm. We give a number of case studies on filters known to be time-varying stable, that cannot be proven time-varying stable with the original criterion, where the new criterion succeeds.
- 6. Inference and Denoise: Causal Inference-based Neural Speech Enhancement
Huck Yang (Georgia Institute of Technology,)Abstract- This study is concerned with enhancing speech signals corrupted by sporadic additive noise. By modeling the noise presence as an intervention, we successfully address the speech enhancement (SE) task within the causal inference paradigm. The proposed causal inference-based speech enhancement (CISE) solution consists of a noise detector and two specular mask-based enhancement modules. In training, the magnitude of the noisy spectrum is fed into both enhancement modules, and the enhanced spectrum is reconstructed leveraging frames picked from those modules according to the presence of noise. In testing, the noise detector predicts the presence or absence of noise in a frame. Experimental evidence demonstrates that CISE outperforms a regular mask-based SE approach in the studied settings. Moreover, the average treatment effect metric is derived to quantify the causal effect adequately. Finally, CISE attains stable word error rates at various noise occurrence ratios.
- 7. Multi-Modal Word Discovery Does Not Improve Textless Speech-to-Speech Translation
Cheng-I Jeff Lai, Hirofumi Inaguma, Paul-Ambroise Duquenne, Hongyu Gong, Ilia Kulikov, Peng-Jen Chen, Yu-An Chung, Yun Tang, Changhan Wang, Holger Schwenk, Wei-Ning Hsu, Ann Lee (MIT, Meta AI/FAIR) - 8. SSAST: Self-Supervised Audio Spectrogram Transformer
Yuan Gong, Cheng-I Jeff Lai, Yu-An Chung, James Glass (MIT)Abstract- Recently, neural networks based purely on self-attention, such as the Vision Transformer (ViT), have been shown to outperform deep learning models constructed with convolutional neural networks (CNNs) on various vision tasks, thus extending the success of Transformers, which were originally developed for language processing, to the vision domain. A recent study showed that a similar methodology can also be applied to the audio domain. Specifically, the Audio Spectrogram Transformer (AST) achieves state-of-the-art results on various audio classification benchmarks. However, pure Transformer models tend to require more training data compared to CNNs, and the success of the AST relies on supervised pretraining that requires a large amount of labeled data and a complex training pipeline, thus limiting the practical usage of AST. This paper focuses on audio and speech classification, and aims to reduce the need for large amounts of labeled data for AST by leveraging self-supervised learning using unlabeled data. Specifically, we propose to pretrain the AST model with joint discriminative and generative masked spectrogram patch modeling (MSPM) using unlabeled audio from AudioSet and Librispeech. We evaluate our pretrained models on both audio and speech classification tasks including audio event classification, keyword spotting, emotion recognition, and speaker identification. The proposed self-supervised framework significantly boosts AST performance on all tasks, with an average improvement of 60.9%, leading to similar or even better results than a supervised pretrained AST. To the best of our knowledge, it is the first patch-based self-supervised learning framework in the audio and speech domain, and also the first self-supervised learning framework for AST.
- 9. Improving Automatic Speech Translation using Semantically Aligned XLSR
Sameer Khurana, Antoine Laurent, James Glass (MIT, Le Mans University)Abstract- We propose the SAMU-XLSR: Semantically-Aligned Multimodal Utterance-level Cross-Lingual Speech Representation learning framework. Unlike previous works on speech representation learning, which learns multilingual contextual speech embedding at the resolution of an acoustic frame (10-20ms), this work focuses on learning multimodal (speech-text) multilingual speech embedding at the resolution of a sentence (5-10s) such that the embedding vector space is semantically aligned across different languages. We combine state-of-the-art multilingual acoustic frame-level speech representation learning model XLS-R with the Language Agnostic BERT Sentence Embedding (LaBSE) model to create an utterance-level multimodal multilingual speech encoder SAMU-XLSR. Although we train SAMU-XLSR with only multilingual transcribed speech data, cross-lingual speech-text and speech-speech associations emerge in its learned representation space. To substantiate our claims, we use SAMU-XLSR speech encoder in combination with a pre-trained LaBSE text sentence encoder for cross-lingual speech-to-text translation retrieval and SAMU-XLSR alone for cross-lingual speech-to-speech translation retrieval. We highlight these applications by performing several cross-lingual text and speech translation retrieval tasks across several datasets. Also, we Fine-Tune SAMU-XLSR for the Multilingual Automatic Speech Translation task and improved 12 BLEU points on CoVoST-2 X to English Speech-to-Text Translation benchmark over XLSR. In particular, SAMU-XLSR improves by 16 BLEU points on low-resource spoken language translation tasks. Furthermore, in Zero-Shot translation scenario, where the translation model is trained on high-resource languages and evaluated on low-resource languages, SAMU-XLSR improves over XLSR by 11 to 18 BLEU points.
- 10. Differentiable object-based synthesis of contact sounds using nonlinear physical constraints
Vinayak Agarwal and Josh McDermott (MIT)Abstract- Object interactions – collisions, scraping and rolling – create many of the sounds that we hear in the world around us. These sounds are generated via lawful physical dynamics. Previous studies have explored ways to synthesize these sounds efficiently and intuitively but could not fully mimic the rich structure of real instances of these sounds. We present a novel source-filter model for realistic synthesis of impact, scraping and rolling sounds with physically and perceptually relevant controllable parameters constrained by principles of mechanics. Key features of our model include non-linearities to constrain the contact force, naturalistic normal force variation for different motions, and a method for morphing impulse responses within a material to achieve location-dependence. Perceptual experiments show that the presented model is able to synthesize realistic impact, scraping and rolling sounds while conveying physical information similar to that in recorded sounds.
- 11. Reverse Engineering a Multitrack Mix with Differentiable Digital Signal Processing
Joseph Colonel and Joshua Reiss (Queen Mary University of London)Abstract- A method to retrieve the parameters used to create a multitrack mix using only raw tracks and the stereo mixdown is presented. This method is able to model both linear and nonlinear time-invariant effects. A mixing chain composed of gain, pan, equalization, delay, reverb, distortion, and dynamic range compression is employed. Stochastic gradient descent can be used to estimate the mixing parameters as each of the modules in the mixing chain is explicitly implemented in a differentiable framework (Tensorflow).
- 12. A Treatise on Lattice Based MMI and CTC Training
Adnan Haider, Tim Ng, Zhen Huang, Xingyu Na and Antti Veikko Rosti (Apple)Abstract- Maximum mutual information (MMI) has become one of the two de facto methods for sequence-level discriminative training of speech recognition acoustic models. This paper aims to isolate, identify and bring forward the implicit modelling decisions induced by the design implementation of standard finite state transducer (FST) lattice based MMI training framework. The paper particularly investigates the necessity to maintain a pre-selected numerator alignment and raises the importance of determinizing FST denominator lattices on the fly. The efficacy of employing on the fly FST lattice determinization while using a fixed numerator alignment is mathematically shown to guarantee discrimination at the hypothesis level and is empirically shown through training deep CNN models on a 18K hours Mandarin dataset and on a 2.8K hours English dataset. On assistant and dictation tasks, the approach achieves between 2.3-4.6% relative WER reduction (WERR) over the standard FST lattice based approach. In addition, this paper presents a formal study on the behavior of CTC training. In particular, through the proof of a lemma, the treatise provides a strong mathematical argument to show how training a conformer word-piece model with CTC implicitly leads to the learning of a word-piece LM conditioned on the acoustic input sequence. The work also presents a discussion on how to quantify the internal LM bias within a CTC trained conformer model. In doing so, it brings forward the positive correlation induced between the run-away blank phenomenon and the strength of the internal LM.
- 13. Spatial Mixup: Directional Loudness Modification as Data Augmentation for Sound Event Localization and Detection
Rircardo Falcon-Perez, Kazuki Shimada, Yuichiro Koyama, Shusuke Takahashi, Yuki Mitsufuji (Aalto University, Sony)Abstract- Data augmentation methods have shown great importance in diverse supervised learning problems where labeled data is scarce or costly to obtain. For sound event localization and detection (SELD) tasks several augmentation methods have been proposed, with most borrowing ideas from other domains such as images, speech, or monophonic audio. However, only a few exploit the spatial properties of a full 3D audio scene. We propose Spatial Mixup, as an application of parametric spatial audio effects for data augmentation, which modifies the directional properties of a multi-channel spatial audio signal encoded in the ambisonics domain. Similarly to beamforming, these modifications enhance or suppress signals arriving from certain directions, although the effect is less pronounced. Therefore enabling deep learning models to achieve invariance to small spatial perturbations. The method is evaluated with experiments in the DCASE 2021 Task 3 dataset, where spatial mixup increases performance over a non-augmented baseline, and compares to other well known augmentation methods. Furthermore, combining spatial mixup with other methods greatly improves performance.
- 14. Towards Optimal Multi-rate Disentangling Models with Weak Supervision
Mohammad Rasool Izadi, Yujia Yan, Shuo Zhang, Robert Stevenson (Bose)Abstract- Learning a generative model with disentangled latent space has attracted some interests in the recent years. In this work, we examine a generative model with a disentangled latent-space for certain sequential data, of which attributes may have different temporal encoding rates. More specifically, we consider weak supervision via swapping multi-rate latent representations of pairs of samples that share the same attribute. Our model uses a transformer based variational autoencoder that can perform encoding and decoding on arbitrary temporal rate ratios. The proposed framework supports the disentanglement and generation of variable-length sequences in a way that it can model each aspect at its optimal rate. We show that this approach can achieve optimal disentanglement from weak supervision theoretically. Our experimental results support the effectiveness of the swapping algorithm for disentangled representation and controllable conversion.
- 15. Investigating Synthesis-Style Speech Enhancement
Bryce Irvin, Marko Stamenovic, Mikolaj Kegler, Richard Yang (Bose)Abstract- Recent years have shown large strides in the fields of audio machine learning in speech enhancement and source separation. Many of these successes approach enhancement through the lens of denoising, removing unwanted noise from speech, and learn masking filters to do so, whether in the spectral domain or implicitly in the latent space. The results of these masking methods are in many cases limited by the characteristics of the original signal. On the other hand, speech synthesis has also seen massive improvements due to deep learning. Many deep networks are now capable of efficiently generating high-quality speech in a causal fashion. In this work, we investigate the utility of synthesis-based techniques applied to speech enhancement. We explore a landscape of different learning criteria and the nature of handcrafted and learned representations. We give additional emphasis to the potential for low-latency applications and perceptual quality maximization and report our findings.
- 16. Classification of Foreign-Accented English
Daisy Lei, Antonio Moreno (The Pennsylvania State University, Interactions LLC)Abstract- Spoken communication is a rich channel of information. Beyond the words we speak, our voice carries a variety of attributes about our gender, age, emotion, mood, health and even geo/socio-economic background. These traits — some long-lived while others short-lived — are collectively known as paralinguistic attributes, making one’s speech a very personal signature closely related to our identity. The goal of our investigation is to support Analytics and Business Intelligence applications by inferring a person's foreign accent from short speech recordings (4sec). Our contribution is twofold. First, the FAE-CV22 accented-speech corpus, a configurable subset of the crowdsourced and freely available Mozilla/Common-Voice (English). Second, a method for accent recognition that repurposes a pre-trained Speaker Recognition DNN model by presenting it with data from FAE-CV22, obtaining an overall 45% accuracy on a recognition task with 11 accent-labels and recordings of around 4sec in length. The methodologies and results presented are fully reproducible on Colab or as a docker container. All source code is available for download on GitHub.
- 17. Music Source Separation with Generative Flow
Ge Zhu, Jordan Darefsky, Fei Jiang, Anton Selitskiy and Zhiyao Duan (University of Rochester)Abstract- Fully-supervised models for source separation are trained on parallel mixture-source data and are currently state-of-the-art. However, such parallel data is often difficult to obtain, and it is cumbersome to adapt trained models to mixtures with new sources. Source-only supervised models, in contrast, only require individual source data for training. In this paper, we first leverage flow-based generators to train individual music source priors and then use these models, along with likelihood-based objectives, to separate music mixtures. We show that in singing voice separation and music separation tasks, our proposed method is competitive with a fully-supervised approach. We also demonstrate that we can flexibly add new types of sources, whereas fully-supervised approaches would require retraining of the entire model.
- 18. SAMO: Speaker Attractor Multi-Center One-Class Learning for Voice Anti-Spoofing
Siwen Ding, You Zhang, Zhiyao Duan (University of Rochester)Abstract- Voice anti-spoofing systems are crucial auxiliaries for automatic speaker verification (ASV) systems. A major challenge is caused by unseen attacks empowered by advanced speech synthesis technologies. Our previous research on one-class learning has improved the generalization ability to unseen attacks by compacting the bona fide speech in the embedding space. However, such compactness lacks consideration of the diversity of speakers. In this work, we propose speaker attractor multi-center one-class learning (SAMO), which clusters bona fide speech around a number of speaker attractors and pushes away spoofing attacks from all the attractors in a high-dimensional embedding space. For training, we propose an EM-like algorithm for the co-optimization of bona fide speech clustering and bona fide/spoof classification. For inference, we propose strategies to enable anti-spoofing for speakers without enrollment. The performance of our system is evaluated on the ASVspoof2019 LA dataset without any augmentation. Our proposed system outperforms existing state-of-the-art single systems with an equal error rate (EER) of 0.91%.
- 19. How do anti-spoofing models generalize on unseen speakers or attacks?
Yongyi Zang, You Zhang, Ge Zhu, Zhiyao Duan (University of Rochester)Abstract- Spoofing attacks have become more sophisticated. With limited training data, generalization ability across diverse scenarios is essential in measuring the robustness of anti-spoofing models. The problem of generalization may be divided into three orthogonal subproblems: unseen channels, speakers, and attacks. The influence of the channel has been investigated; however, the generalization to unseen speakers and attacks are often examined jointly. To understand this problem more deeply, we set up two scenarios: unseen speakers (all attacks seen) and unseen attacks (all speakers seen), to examine their influence on the anti-spoofing model’s performance.
- 20. HRTF Field: Unifying Measured HRTF Magnitude Representation with Neural Field
You Zhang, Yuxiang Wang, Mark Bocko, Zhiyao Duan (University of Rochester)Abstract- Head-related transfer functions (HRTFs) are a set of functions of frequency describing the spatial filtering effect of the outer ear (i.e., torso, head, and pinnea) onto sound sources at different azimuth and elevation angles. They are widely used in spatial audio rendering. While the azimuth and elevation angles are intrinsically continuous, measured HRTFs in existing datasets employ specific spatial sampling schemes, making it difficult to model across datasets with different sampling schemes. In this work, we propose to use neural fields, a differentiable representation of functions through neural networks, to model HRTFs with arbitrary spatial sampling schemes. Such representation is differentiable with respect to azimuth and elevation angles and is unified across datasets with different spatial sampling schemes. We further introduce a generative model to learn the latent space of the HRTF neural field representation. We demonstrate that HRTFs for arbitrary azimuth and elevation angles can be derived from this representation. We believe that it will significantly advance data-driven research on many tasks, such as HRTF interpolation and personalization.
- 21. ControlVC: Zero-Shot Voice Conversion with Time-Varying Controls
Meiying Chen and Zhiyao Duan (University of Rochester)Abstract- Recent developments in neural speech synthesis and vocoding have sparked a renewed interest in voice conversion (VC). Beyond timbre transfer, achieving controllability on para-linguistic parameters such as pitch and rhythm is critical in deploying VC systems in many application scenarios. Existing studies, however, either only provide utterance-level global control or lack interpretability on the controls. In this paper, we propose ControlVC, the first neural voice conversion system that achieves time-varying controls on pitch and rhythm. ControlVC uses pre-trained encoders to compute pitch embeddings and linguistic embeddings from the source utterance and speaker embeddings from the target utterance. These embeddings are then concatenated and converted to speech using a vocoder. It achieves rhythm control through TD-PSOLA pre-processing on the source utterance, and achieves pitch control by manipulating the pitch contour before feeding it to the pitch encoder. Systematic subjective and objective evaluations are conducted to assess the speech quality and controllability. Results show that, on non-parallel and zero-shot conversion tasks, ControlVC significantly outperforms two other self-constructed baselines on speech quality, and it can successfully achieve time-varying pitch control.
- 22. On the pragmatism of using binary classifiers over data-intensive neural network classifiers for detection of COVID-19 from voice
Ankit Shah, Hira Dhamyal, Yang Gao, Rita Singh, Bhiksha Raj (CMU)Abstract- Lately, there has been a global effort by multiple research groups to detect COVID-19 from voice. Different researchers use different kinds of information from the voice signal to achieve this. Various types of phonated sounds and the sound of cough and breath have all been used with varying degrees of success in automated voice-based COVID-19 detection apps. In this paper, we show that detecting COVID-19 from voice does not require custom-made non-standard features or complicated neural network classifiers rather it can be successfully done with just standard features and simple binary classifiers. In fact, we show that the latter is not only more accurate and interpretable and also more computationally efficient in that they can be run locally on small devices. We demonstrate this from a human-curated dataset collected and calibrated in clinical settings. On this dataset which comprises over 1000 speakers, a simple binary classifier is able to achieve 94% detection accuracy.
- 23. An overview of techniques for biomarker discovery in voice signal
Ankit Shah, Hira Dhamyal, Rita Singh (CMU)Abstract- This paper reflects on the effect of several categories of medical conditions on the human voice, focusing on those that may be hypothesized to have effects on voice, but for which the changes themselves may be subtle enough to have eluded observation in standard analytical examinations of the voice signal. It presents three categories of techniques that can potentially uncover such elusive biomarkers and allow them to be measured and used for predictive and diagnostic purposes. These approaches include proxy techniques, model-based analytical techniques, and data-driven AI techniques.
- 24. Bootstrapping Speech-To-Text for Gaming Voice Chat using wav2vec 2.0
Rachel Manzelli, Chinmay Warang (Modulate)Abstract- Creating robust speech-to-text (STT) systems that perform well on real-world data has proven to be a difficult task. When working with voice chat in video games, this problem becomes much more complex due to understudied anomalies in voice chat data. These include differences in audio fidelity due to different microphone setups (compression, clipping, lack of echo cancellation) as well as behavioral differences such as voice raids and heavy background noise (music, video game sound effects, etc). We describe a method to bootstrap a robust STT system via a pretrained wav2vec2.0 model and a small amount of labeled finetuning data from the voice chat domain. This model shows improvements in Word Error Rate (WER) over AWS Transcribe.
- 25. N-gram boosting: Improving contextual biasing with normalized n-gram targets
Wang Yau Li, Shreekantha Nadig, Karol Chang, Zafarullah Mahmood, Riqiang Wang, Simon Vandieken, Jonas Robertson, Fred Mailhot (Dialpad Inc)Abstract- Accurate transcription of proper names and technical terms is particularly important in speech-to-text applications for business conversations. These words, which are essential to understanding the conversation, are often rare and therefore likely to be under-represented in text and audio training data, creating a significant challenge in this domain. We present a two-step keyword boosting mechanism that successfully works on normalized unigrams and n-grams rather than just single tokens, which eliminates missing hits issues with boosting raw targets. We also modified the boosting weight application logic to avoid overboosting issues on n-grams. This improves our keyword recognition rate by 26% relative on our proprietary in-domain dataset and 2% on LibriSpeech, particularly on targets that involve alphanumeric characters.
- 26. Improving ASR accuracy on a collection of dialects using active learning techniques
Riqiang Wang*, Yiming Zhang*, Tania Habib, Shreekantha Nadig, Simon Vandieken, Jonas Robertson (Dialpad Inc)Abstract- A robust and equitable business automatic speech recognition (ASR) system needs to generalize well over a diverse set of accents and dialects. However, there is a prohibitive cost in collecting additional dialects data as dialects are difficult to identify, and data for many dialects is scarce. To approach this challenge, we fine-tuned our ASR model using a small subset of our data, borrowing ideas from active learning. Specifically, we selected data with low ASR confidence scores, using the average confidence score for each utterance, as well as for each speaker. This constraint on speakers’ confidence score helps eliminate outlier utterances having low confidence for reasons other than dialects. In addition, we reviewed and re-transcribed the selected data to ensure its quality. The fine-tuned model achieved up to 8.5% relative improvement on word error rate (WER) on a collection of dialects in our proprietary test set for unidentified dialects in the US while also providing improvement on the main US dialect. However, it is unclear whether this technique will generalize to any targeted domain and future work will focus on more generalized data selection criteria as well as the scalability of the technique.
- 27. EDANSA-2019: The Ecoacoustic Dataset from Arctic North Slope Alaska
Enis Berk Çoban, Megan Perra, Dara Pir, Michael I Mandel (CUNY)Abstract- The Arctic is warming at three times the rate of the global average, affecting the habitat and lifecycles of migratory species that reproduce there, like birds and caribou. Ecoacoustic monitoring can help efficiently track changes in animal phenology and behavior over large areas so that the impacts of climate change on these species can be better understood and potentially mitigated. We introduce here the Ecoacoustic Dataset from Arctic North Slope Alaska (EDANSA-2019), a dataset collected by a network of 100 autonomous recording units covering an area of 9000 square miles over the course of the 2019 summer season on the North Slope of Alaska and neighboring regions. We labeled over 27 hours of this dataset according to 28 tags with enough instances of 9 important environmental classes to train baseline convolutional recognizers. We are releasing this dataset and the corresponding baseline to the community to accelerate the recognition of these sounds and facilitate automated analyses of large-scale ecoacoustic databases.
- 28. Speaker Extraction with Co-Speech Gestures Cue
Zexu Pan, Xinyuan Qian, Haizhou Li (National University of Singapore)Abstract- Speaker extraction seeks to extract the clean speech of a target speaker from a multi-talker mixture speech. There have been studies to use a pre-recorded speech sample or face image of the target speaker as the speaker cue. In human communication, co-speech gestures that are naturally timed with speech also contribute to speech perception. In this work, we explore the use of co-speech gestures sequence, e.g. hand and body movements, as the speaker cue for speaker extraction, which could be easily obtained from low-resolution video recordings, thus more available than face recordings. We propose two networks using the co-speech gestures cue to perform attentive listening on the target speaker, one that implicitly fuses the co-speech gestures cue in the speaker extraction process, the other performs speech separation first, followed by explicitly using the co-speech gestures cue to associate a separated speech to the target speaker. The experimental results show that the co-speech gestures cue is informative in associating with the target speaker.
- 29. TorchAudio 0.12: IO, ASR, SSL, and More
Caroline Chen, Jeff Hwang, Moto Hira, Xiaohui Zhang, Zhaoheng Ni, Yumeng Tao (PyTorch, Meta)Abstract- TorchAudio is an open-source library that provides essential building blocks for audio and speech processing. It has been widely adopted by research community and other open-source libraries (e.g., ESPnet, SpeechBrain, Lhotse, etc). In the 0.12 release, we added more supports for both low-level utilities and deep learning based speech tasks. In this paper, we introduce the new added features into four categories: audio IO/feature extraction, automatic speech recognition (ASR), self-supervised learning (SSL), source separation/speech synthesis. In the future development, we will continue the supports and welcome feedback and contributions from the audio and speech community.