SANE 2019 - Speech and Audio in the Northeast
October 24, 2019
The workshop is now over. Videos and slides for the talks are available through the links in the schedule below. There is also a YouTube Playlist for all talks.
SANE 2019, a one-day event gathering researchers and students in speech and audio from the Northeast of the American continent, was held on Thursday October 24, 2019 at Columbia University, in New York City.
It was the 8th edition in the SANE series of workshops, which started in 2012 and has been held every year alternately in Boston and New York. Since the first edition, the audience has steadily grown, with a previous record of 180 participants in 2017 and 2018, and a new record of 200 participants and 45 posters in 2019.
This year's SANE conveniently took place in conjunction both with the WASPAA workshop, held October 20-23 in upstate New York, and with the DCASE workshop, held October 25-26 in Brooklyn, NY, for a full week of speech and audio enlightenment and delight.
SANE 2019 featured invited talks by leading researchers from the Northeast as well as from the international community. It also featured a lively poster session, open to both students and researchers, in Columbia University's Low Memorial Library, a National Historic Landmark.
Details
- Date: Thursday, October 24, 2019
- Venue: Schapiro Center, Columbia University, New York, NY
Schedule [Watch all recorded talks on YouTube]
Click on the talk title to jump to the abstract and bio, and on Poster Session for the list of posters.8:30-8:55 | Breakfast |
8:55-9:00 | Welcome and insights on the SANE audience [Youtube] [Slides] |
9:00-9:45 | Brian Kingsbury (IBM TJ Watson Research Center) Training neural networks faster, and trying to understand what they're doing [Youtube] [Slides] |
9:45-10:30 | Kristen Grauman (University of Texas at Austin, Facebook AI Research) Eyes and Ears: Learning to Disentangle Sounds in Unlabeled Video [Youtube] [Slides] |
10:30-11:00 | Coffee break |
11:00-11:10 | Live Demo: Jonathan Le Roux (MERL), Seamless ASR [Youtube] [Slides] |
11:10-11:55 | Simon Doclo (University of Oldenburg) Blind multi-microphone noise reduction and dereverberation algorithms for speech communication applications [Youtube] [Slides] |
11:55-14:10 | Lunch and Poster Session in the Low Memorial Library |
14:10-14:20 | Live Demo: Andrew Titus (Apple), Automatic Language Selection in Dictation |
14:20-15:05 | Karen Livescu (TTI-Chicago) Acoustic (and acoustically grounded) word embeddings [Youtube] [Slides] |
15:05-15:50 | Gabriel Synnaeve (Facebook AI Research) wav2letter and the Many Meanings of End-to-End Automatic Speech Recognition [Youtube] [Slides] |
15:50-16:20 | Coffee break |
16:20-16:30 | Live Demo: Yin Cao, Saeid Safavi, Mark Plumbley (U. of Surrey), Sound Recognition & Generalization [Youtube] [Slides] |
16:30-17:15 | Hirokazu Kameoka (NTT Communication Science Laboratories) Voice conversion with image-to-image translation and sequence-to-sequence learning approaches [Youtube] [Slides] |
17:15-18:00 | Ron Weiss (Google Brain) (Live Demo by Fadi Biadsy) Generating speech from speech: How end-to-end is too far? [Youtube] [Slides] |
18:00-18:15 | Closing remarks |
18:15-........ | Drinks somewhere nearby |
Registration
The workshop is now over. If you are interested in attending future SANE events, please sign up to the SANE News mailing list.
Directions
The workshop was hosted at the Schapiro Center for Engineering and Physical Science Research, Columbia University, in New York City, NY. The closest subway station is the 1 Train's 116 Street Station - Columbia University.
Organizing Committee
- Jonathan Le Roux (MERL)
- Nima Mesgarani (Columbia)
- John R. Hershey (Google)
- Shinji Watanabe (Johns Hopkins)
- Steven J. Rennie (Pryon Inc.)
Sponsors
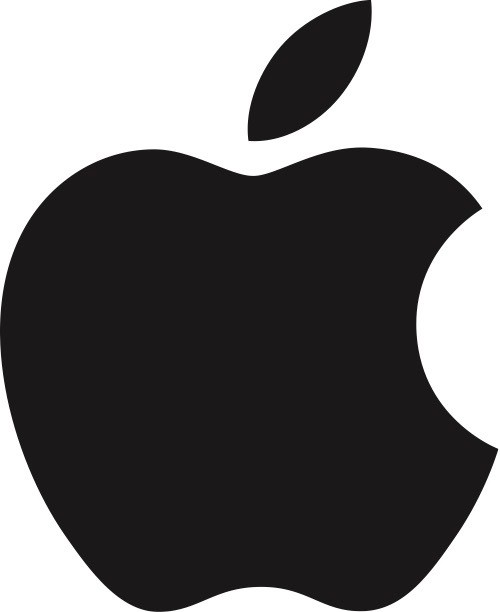
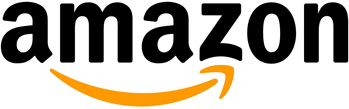
Talks
Training neural networks faster, and trying to understand what they're doing
This will be a two-part talk. In the first part, I will describe our work on using asynchronous, decentralized parallel stochastic gradient descent to accelerate the training of large acoustic models for speech recognition. I'll explain how we can reduce the wall-clock time for training a bidirectional LSTM model on the 2000-hour Switchboard dataset from 203 hours on a single NVIDIA V100 GPU to 5.2 hours on 64 V100s. In the second part of the talk, I will discuss the estimation of the mutual information I(X;T_ℓ) between the input features X and the output of the ℓ-th hidden layer T_ℓ. I(X;T_ℓ) is a quantity of interest in theories such as the information bottleneck, which try to explain the success of deep learning. I will show that in networks with internal noise, I(X;T_ℓ) is meaningful and there is a rigorous framework for its estimation. Moreover, compression (a decrease in I(X;T_ℓ) over epochs of training) is driven by progressive clustering of training samples from the same class. In deterministic networks, I will explain why I(X;T_ℓ) is vacuous, and show that the binning-based approximation of I(X;T_ℓ) used in previous studies was, in fact, also measuring clustering. This second project is joint work between IBM Research AI and MIT.
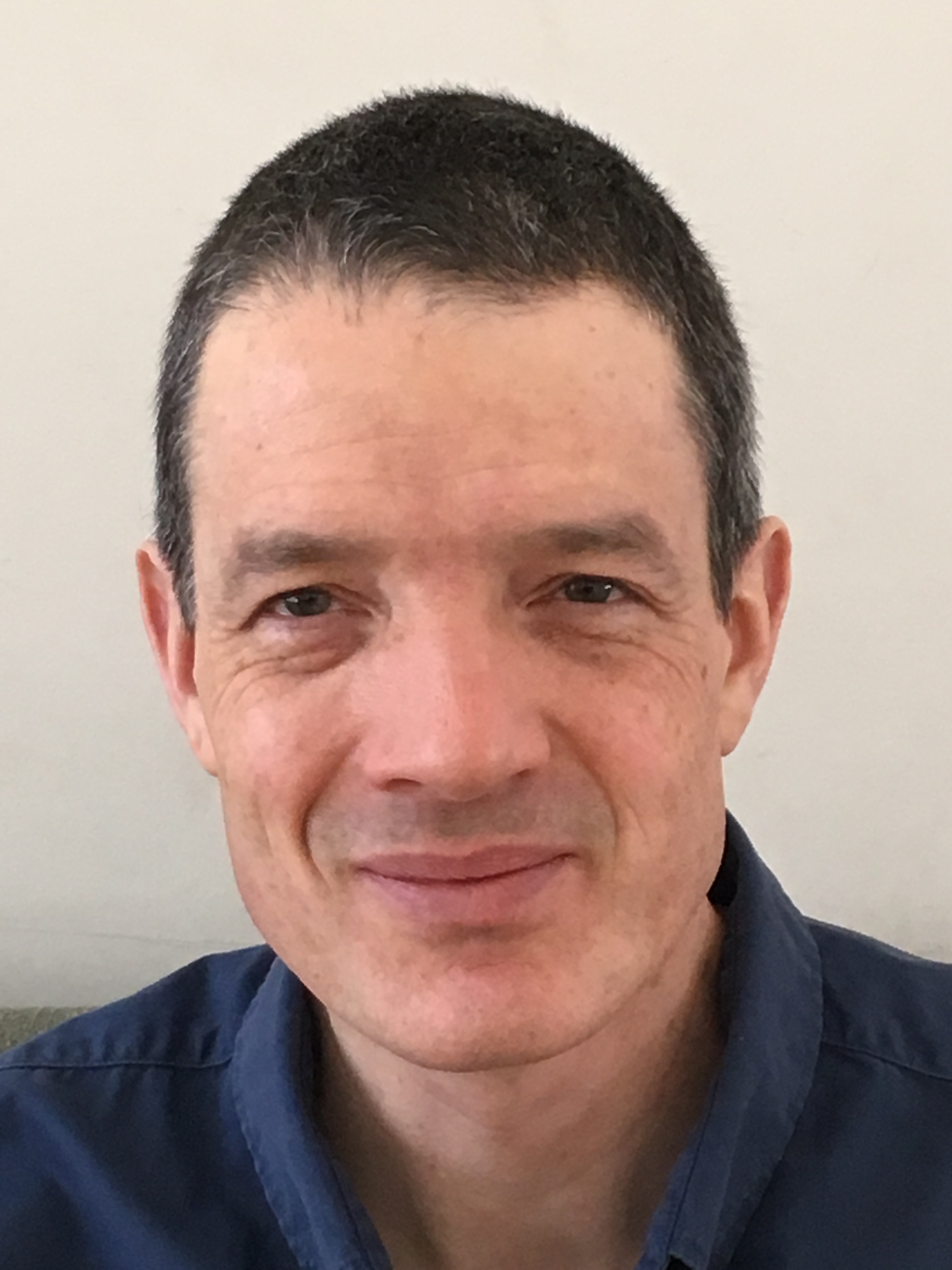
Brian Kingsbury is a distinguished research staff member in IBM Research AI and manager of the Speech Technologies research group at the T. J. Watson Research Center in Yorktown Heights, NY. He earned a BS in electrical engineering from Michigan State University and a PhD in computer science from the University of California, Berkeley. His research interests include deep learning, optimization, large-vocabulary speech transcription, and keyword search. From May 2012 until November 2016 he was co-PI and technical lead for LORELEI, an IBM-led consortium participating in the IARPA Babel program. Brian has contributed to IBM's entries in numerous competitive evaluations of speech technology, including Switchboard, SPINE, EARS, Spoken Term Detection, and GALE. He has served as as a member of the Speech and Language Technical Committee of the IEEE Signal Processing Society (2009-2011); as an ICASSP speech area chair (2010-2012); an associate editor for IEEE Transactions on Audio, Speech, and Language Processing (2012-2016); and as a program chair for the International Conference on Representation Learning (2014-2016). He is an author or co-author on more than 100 publications on speech recognition, machine learning, and VLSI design.
Eyes and Ears: Learning to Disentangle Sounds in Unlabeled Video
University of Texas at Austin, Facebook AI Research
Understanding scenes and events is inherently a multi-modal experience: we perceive the world by both looking and listening. In this talk, I will present our recent work learning audio-visual models from unlabeled video. A key challenge is that typical videos capture object sounds not as separate entities, but as a single audio channel that mixes all their frequencies together and obscures their spatial layout. Considering audio as a source of both semantic and spatial information, we explore learning multi-modal models from real-world video comprised of multiple sound sources. In particular, we introduce new methods for visually guided audio source separation and “2.5D visual sound”, which lifts monaural audio into its immersive binaural counterpart via the visual video stream.
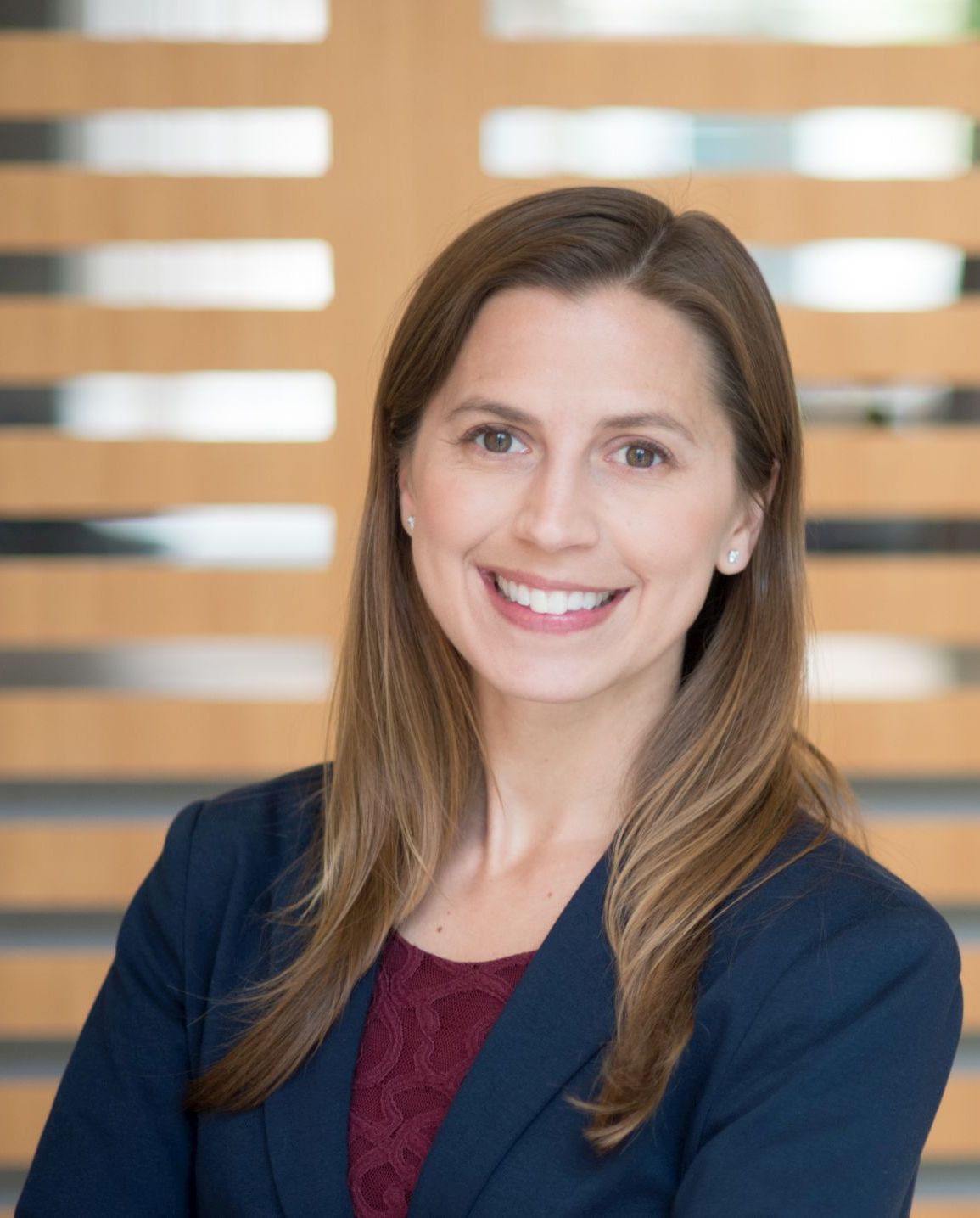
Kristen Grauman is a Professor in the Department of Computer Science at the University of Texas at Austin and a Research Scientist at Facebook AI Research. Her research in computer vision and machine learning focuses on visual recognition and search. Before joining UT Austin in 2007, she received her Ph.D. at MIT. She is a AAAI Fellow, a Sloan Fellow, and a recipient of the NSF CAREER, ONR YIP, PECASE, PAMI Young Researcher award, and the 2013 IJCAI Computers and Thought Award. She and her collaborators were recognized with best paper awards at CVPR 2008, ICCV 2011, ACCV 2016, and a 2017 Helmholtz Prize “test of time” award. She served as a Program Chair of the Conference on Computer Vision and Pattern Recognition (CVPR) in 2015 and Neural Information Processing Systems (NeurIPS) in 2018, and she currently serves as Associate Editor-in-Chief for the Transactions on Pattern Analysis and Machine Intelligence (PAMI).
Blind multi-microphone noise reduction and dereverberation algorithms for speech communication applications
Despite the progress in speech enhancement algorithms, speech understanding in adverse acoustic environments with background noise, competing speakers and reverberation is still a major challenge in many speech communication applications. In this presentation, some recent advances in blind multi-microphone noise reduction and dereverberation algorithms will be presented, with a particular focus on the multi-channel Wiener filter (MWF). First, several methods to jointly estimate all required time-varying quantities, i.e. the relative transfer functions of the target speaker and the power spectral densities of the target speaker, the reverberation and the noise, will be presented. Second, speech enhancement algorithms for binaural hearing devices will be discussed, where the objective is not only to selectively extract the target speaker and suppress background noise and reverberation, but also to preserve the auditory impression of the complete acoustic scene. Aiming at preserving the binaural cues of all sound sources while not degrading the noise reduction performance, different extensions of the binaural MWF will be presented, both for diffuse noise as well as for interfering sources. Third, it will be shown how such algorithms can be used in acoustic sensor networks, exploiting the spatial distribution of the microphones. Evaluation results will be presented in terms of objective performance measures as well as subjective listening scores for speech intelligibility and spatial quality.
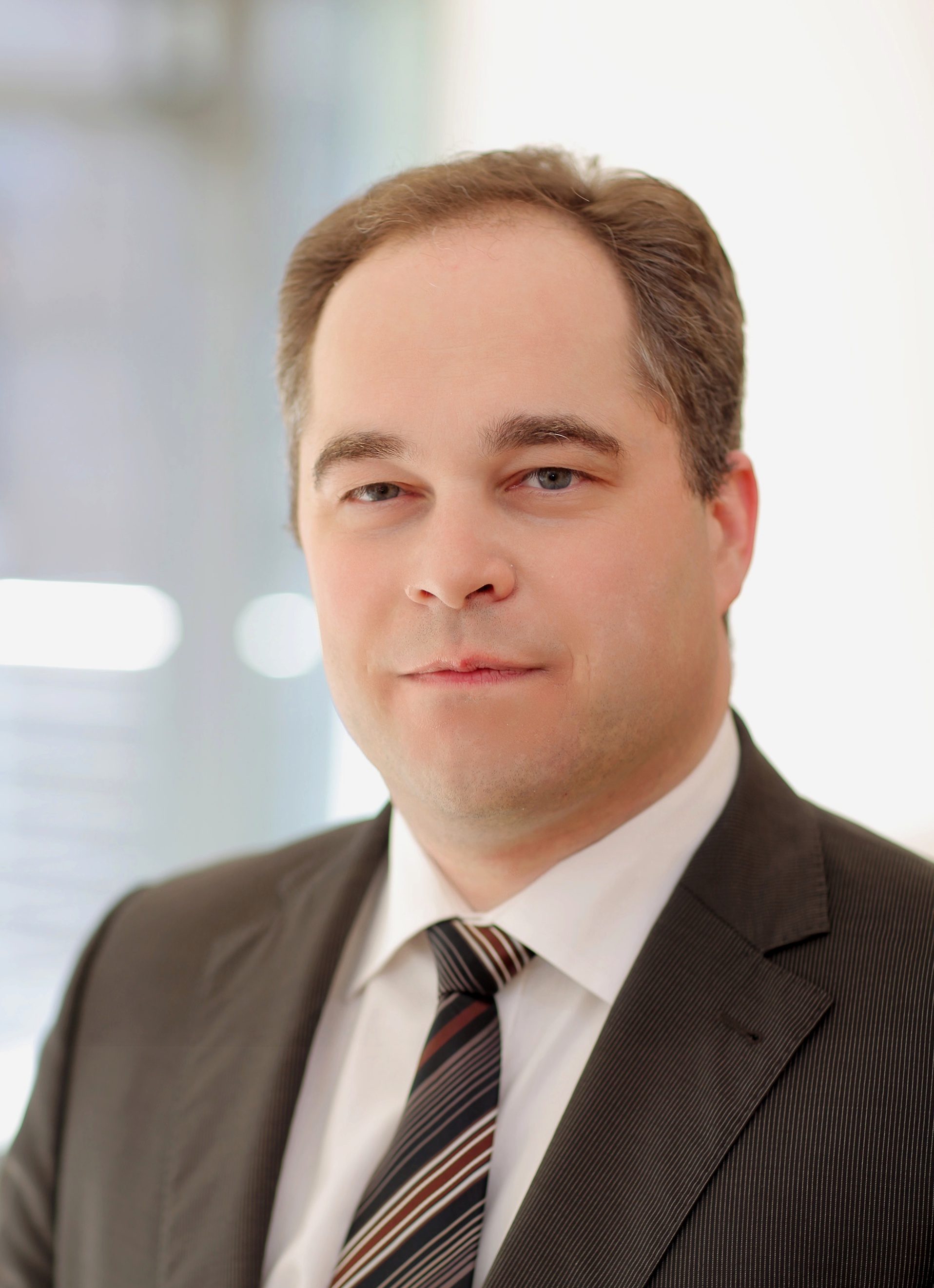
Simon Doclo received the M.Sc. degree in electrical engineering and the Ph.D. degree in applied sciences from KU Leuven, Belgium, in 1997 and 2003. From 2003 to 2007 he was a Postdoctoral Fellow at the Electrical Engineering Department (KU Leuven) and the Cognitive Systems Laboratory (McMaster University, Canada). From 2007 to 2009 he was a Principal Scientist with NXP Semiconductors in Leuven, Belgium. Since 2009 he is the head of the Signal Processing Group at the University of Oldenburg, Germany, and scientific advisor of the Fraunhofer Institute for Digital Media Technology. His research activities center around acoustical and biomedical signal processing, more specifically microphone array processing, speech enhancement, active noise control, auditory attention decoding and hearing aid processing.
Prof. Doclo received several awards, among which the EURASIP Signal Processing Best Paper Award in 2003, the IEEE Signal Processing Society 2008 Best Paper Award and the best paper award of the Information Technology Society (ITG) in 2019. He is member of the IEEE Signal Processing Society Technical Committee on Audio and Acoustic Signal Processing, the EURASIP Special Area Team on Acoustic, Speech and Music Signal Processing and the EAA Technical Committee on Audio Signal Processing. Prof. Doclo was Technical Program Chair of the IEEE Workshop on Applications of Signal Processing to Audio and Acoustics (WASPAA) in 2013 and Chair of the ITG Conference on Speech Communication in 2018. In addition, he served as guest editor for several special issues (IEEE Signal Processing Magazine, Elsevier Signal Processing) and is associate editor for IEEE/ACM Transactions on Audio, Speech and Language Processing and EURASIP Journal on Advances in Signal Processing.
Acoustic (and acoustically grounded) word embeddings
A ubiquitous tool in natural language processing is word embeddings, which represent the meanings of written words. On the other hand, for spoken language applications it may be more important to represent how a written word *sounds* rather than (or in addition to) what it means. For some applications it can also be helpful to represent variable-length acoustic segments corresponding to words, or other linguistic units, as fixed-dimensional vectors. This talk will present recent work on both acoustic word embeddings and "acoustically grounded" written word embeddings, including applications in speech recognition and search.
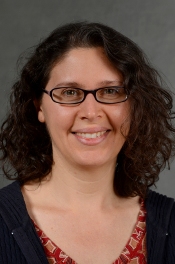
Karen Livescu is an Associate Professor at TTI-Chicago. She completed her PhD in electrical engineering and computer science at MIT. Her main research interests are in speech and language processing and machine learning. Her recent work includes multi-view representation learning, acoustic word embeddings, visually grounded speech modeling, and automatic sign language recognition. Her recent professional activities include serving as a member of the IEEE Spoken Language Technical Committee, an associate editor for IEEE Transactions on Audio, Speech, and Language Processing, a technical co-chair of ASRU 2015/2017/2019, and a program co-chair of ICLR 2019.
wav2letter and the Many Meanings of End-to-End Automatic Speech Recognition
What does it mean for an automatic speech recognition (ASR)system to be end-to-end? Why do we care if it is end-to-end or not? We will present different facets of making a speech recognition system end-to-end, from starting from the waveform instead of speech features, to outputting words directly, differentiating through the decoder, and decoding with or without explicit language models.
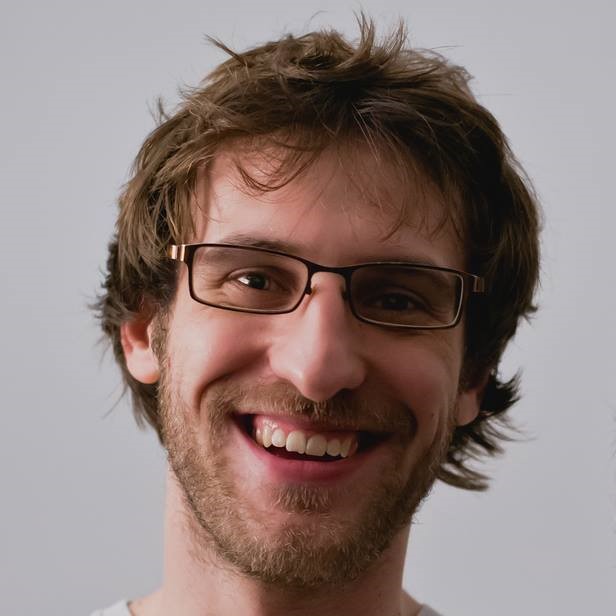
Gabriel Synnaeve is a research scientist on the Facebook AI Research (FAIR) team, which he joined as a postdoctoral researcher in 2015. He lead StarCraft AI research (2016-2019) with the TorchCraftAI team, and he worked on speech recognition there since the beginning of wav2letter (2015-). Prior to Facebook, he was a postdoctoral fellow in Emmanuel Dupoux’s team at École Normale Supérieure in Paris, working on reverse-engineering the acquisition of language in babies. He received his PhD in Bayesian modeling applied to real-time strategy games AI from University of Grenoble in 2012. Even before that (2009), he worked on inductive logic programming applied to systems biology at the national institute of informatics in Tokyo.
Voice conversion with image-to-image translation and sequence-to-sequence learning approaches
NTT Communication Science Laboratories
There are many kinds of barriers that prevent individuals from efficient and smooth verbal communication. Examples of such barriers include language barriers and voice disorders. One key technique to overcome some of these barriers is voice conversion (VC), a technique to convert para/non-linguistic information contained in a given utterance without changing the linguistic information. In this talk, I will present our recent attempts to adopt two approaches to tackle VC problems. One is an image-to-image translation approach, where audio spectral sequences are viewed as natural images so that the "image texture" of the entire spectral sequence of source speech is converted to that resembling target speech. We have shown that the idea introduced to realize image-to-image translation from unpaired examples can also work for non-parallel VC tasks, where no parallel utterances, transcriptions, or time alignment procedures are required for training. The other is a sequence-to-sequence learning approach, which has already been applied with notable success to automatic speech recognition and text-to-speech. While many conventional VC methods are mainly focused on learning to convert only the voice characteristics, the sequence-to-sequence learning approach allows us to convert not only the voice characteristics but also the pitch contour and duration of input speech, thus realizing even more flexible conversion. I will demonstrate how these approaches perform in several VC tasks.
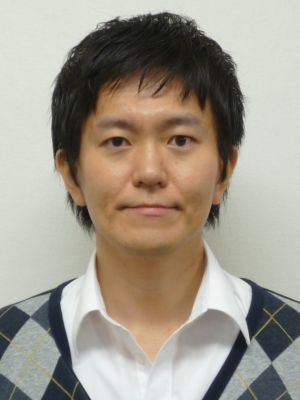
Hirokazu Kameoka received the B.E., M.S., and Ph.D. degrees from the University of Tokyo, Tokyo, Japan, in 2002, 2004, and 2007, respectively. He is currently a Distinguished Researcher with the NTT Communication Science Laboratories, Nippon Telegraph and Telephone Corporation, Atsugi, Japan, and an Adjunct Associate Professor with the National Institute of Informatics, Tokyo, Japan. From 2011 to 2016, he was an Adjunct Associate Professor with the University of Tokyo. He is the author or co-author of about 150 articles in journal papers and peer-reviewed conference proceedings. His research interests include audio, speech, and music signal processing, and machine learning. He has been an Associate Editor for the IEEE/ACM Transactions on Audio, Speech, and Language Processing since 2015, a member of the IEEE Audio and Acoustic Signal Processing Technical Committee since 2017, and a member of the IEEE Machine Learning for Signal Processing Technical Committee since 2019. He was the recipient of 17 awards, including the IEEE Signal Processing Society 2008 SPS Young Author Best Paper Award.
Generating speech from speech: How end-to-end is too far?
End-to-end neural network models for speech recognition and synthesis have become very popular in recent years. Given sufficient training data, such models have obtained state of the art performance on both tasks, resulting in compact models compared to more conventional approaches with substantially simplified data requirements and training configurations.
Building on these successes, I will describe several recent results demonstrating how sequence-to-sequence speech recognition and synthesis networks can be adapted to solve even more complex speech tasks, including voice conversion/normalization of atypical speech and speech (to text and speech) translation, ultimately combining speech recognition, machine translation, and speech synthesis into a single neural network.
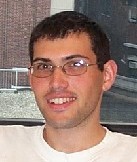
Ron Weiss is a software engineer at Google Brain where he works at the intersection between machine learning and sound, most recently focusing on speech recognition, translation, and synthesis. Ron completed his Ph.D. in electrical engineering from Columbia University in 2009 where he worked in the Laboratory for the Recognition of Speech and Audio. From 2009 to 2010 he was a postdoctoral researcher in the Music and Audio Research Laboratory at New York University.
Posters
Instructions for posters: the poster boards are 30"x40", and can be placed in portrait or landscape orientation.
- Machine-learning-based estimation of acoustical parameters using room geometry for room effect rendering
Ricardo Falcon Perez, Georg Gotz, Ville Pulkki (Aalto University)Abstract- The reverberation of sound inside rooms has a significant impact on sound quality. In virtual reality, the reverberation of rooms should be reproduced with sufficiently high accuracy to produce plausible rendering of room acoustics. One of the most important parameters when rendering reverberation artificially is the reverberation time, and the value for it should be estimated from the geometry of the virtual room, for any given source/receiver position and orientation. While complete physical models are typically too computationally costly, an approximation can be made using simplified mathematical models such as Sabine of Eyring formulas, at the expense of reduced accuracy. This work shows a proof of concept for a machine learning based method that predicts the reverberation time of a room, using geometric information and absorption coefficients as inputs. The proposed model is trained and evaluated using a novel dataset composed of real-world acoustical measurements of a single room with 832 different configurations of furniture and absorptive materials, for multiple loudspeaker positions. The method achieves a prediction accuracy of approximately 90 % for most frequency bands. Furthermore, when comparing against the Sabine and Eyring methods, the proposed approach exhibits a much higher accuracy, especially at low frequencies.
- Learning Gaussian Receptive Fields for Audio Recognition
Evan Shelhamer (Adobe/MIT)Abstract- Receptive field design is key to learning effective deep representations for audio recognition. There are many possible filter sizes for temporal and spectral-temporal convolution and accuracy can rely on the right choice. We make filter size differentiable by composing free-form convolutional filters with Gaussian filters. Optimizing their covariance end-to-end controls filter size to tune temporal and spectral dimensions to the task and data. We benchmark our compositional free-form and Gaussian convolution against standard and dilated convolutional architectures for environmental sound recognition on ESC-50 and UrbanSounds8k.
- SNDCNN: Self-Normalizing Deep CNNs With Scaled Exponential Linear Units for Speech Recognition
Zhen Huang, Tim Ng, Leo Liu, Henry Mason, Xiaodan Zhuang, Daben Liu (Apple)Abstract- Very deep CNNs achieve state-of-the-art results in both computer vision and speech recognition, but are difficult to train. The most popular way to train very deep CNNs is to use shortcut connections (SC) together with batch normalization (BN). Inspired by Self- Normalizing Neural Networks, we propose the self-normalizing deep CNN (SNDCNN) based acoustic model topology, by removing the SC/BN and replacing the typical RELU activations with scaled exponential linear unit (SELU) in ResNet-50. SELU activations make the network self-normalizing and remove the need for both shortcut connections and batch normalization. Compared to ResNet-50, we can achieve the same or lower word error rate (WER) while at the same time improving both training and inference speed by 60%- 80%. We also explore other model inference optimizations to further reduce latency for production use.
- Improving Language Identification for Multilingual Speakers
Andrew Titus, Jan Silovsky, Nanxin Chen, Roger Hsiao, Mary Young, Arnab Ghoshal (Apple, Johns Hopkins University )Abstract- Spoken language identification (LID) technologies have improved in recent years from discriminating largely distinct languages to discriminating highly similar languages or even dialects of the same language. One aspect that has been mostly neglected, however, is discrimination of languages for multilingual speakers, despite being a primary target audience of many systems that utilize LID technologies. As we show in this work, LID systems can have a high average accuracy for most combinations of languages while greatly underperforming for others when accented speech is present. We address this by using coarser-grained targets for the acoustic LID model and integrating its outputs with interaction context signals in a context-aware model to tailor the system to each user. This combined system achieves an average 97% accuracy across all language combinations while improving worst-case accuracy by over 60% relative to our baseline.
- DNNs versus Codebooks based Spectral Envelope Estimation for Partial Reconstruction of Speech Signals
Christopher Seitz, Mohammed Krini (Aschaffenburg University of Technology)Abstract- Improvement in the speech quality achieved by conventional noise suppression methods in high noise conditions is very limited and thus can be further improved. This is possible by adopting speech reconstruction techniques for recovering highly disturbed speech components. The speech reconstruction method presented in this paper is based on the so-called source-filter model of speech production. The focus hereby will be on the estimation of vocal tract filter characteristics (spectral envelope) at high noise conditions which is shown to be important for speech reconstruction. For this purpose a deep recurrent neural network (Deep-RNN) operating as a regression model given noisy features is utilized. The performance of such trained Deep-RNN will be compared with a priori trained spectral envelope codebooks. Furthermore, a synthetic speech signal is generated using the envelope estimated by the Deep-RNN and is then mixed adaptively with a conventionally noise reduced signal based on the SNR. The quality of the resulting enhanced speech is analysed with objective measures such as the log-spectral distance and also with subjective tests. Both the tests show that a remarkable qualitative improvement is possible compared to conventional schemes - especially in high noise scenarios.
- Improving Polyphonic Sound Event Detection Metrics
Cagdas Bilen, Francesco Tuveri, Giacomo Ferroni, Juan Azcarreta and Sacha Krstulovic (Audio Analytic - AA Labs)Abstract- In recent years, academic and industrial interest for automatic Sound Event Detection (SED) has been growing significantly, as suggested by the noticeable increase in the number of contributions to academic conferences, workshops and SED competitive evaluations in the past 5 years. However, in contrast to other well-established fields such as Automatic Speech Recognition (ASR), evaluation criteria for SED tasks have been widely varying across academic publications or public competitions. This hinders the development of the field insofar as it prevents the direct comparison of the published techniques. In this poster, we define a new and general framework for performance evaluation of polyphonic sound event detection systems, which improves upon existing metrics by tackling particular points of concern that are relevant to both academia and industry when assessing the performance of SED systems.
- Speech Declipping Using Complex Time-Frequency Filters
Wolfgang Mack and Emanuël A. P. Habets (AudioLabs Erlangen)Abstract- Recorded signals can be clipped in case the sound pressure or analog signal amplification is too large. Clipping is a non-linear distortion, which limits the maximal magnitude modulation of the signal and changes the energy distribution in frequency domain and hence degrades the quality of the recording. Consequently, for declipping, some frequencies have to be amplified, and others attenuated. We propose a declipping method by using the recently proposed deep filtering technique which is capable of extracting and reconstructing a desired signal from a degraded input. Deep filtering operates in the short-time Fourier transform (STFT) domain estimating a complex multidimensional filter for each desired STFT bin. The filters are applied to defined areas of the clipped STFT to obtain for each filter a single complex STFT bin estimation of the declipped STFT. The filter estimation, thereby, is performed via a deep neural network trained with simulated data using soft- or hard-clipping. The loss function minimizes the reconstruction mean-squared error between the non-clipped and the declipped STFTs. We evaluate our approach using simulated data degraded by hard- and soft-clipping and conducted a pairwise comparison listening test with measured signals comparing our approach to one commercial and one open-source declipping method. Our approach outperformed the baselines for declipping speech signals for measured data for strong and medium clipping.
- Multi-Microphone Speaker Separation based on Deep DOA Estimation
Shlomo E. Chazan, Hodaya Hammer, Gershon Hazan, Jacob Goldberger and Sharon Gannot (Bar-Ilan University)Abstract- In this work, we present a multi-microphone speech separation algorithm based on masking inferred from the speaker’s DOA. According to the W-disjoint orthogonality property of speech signals, each TF bin is dominated by a single speaker. This TF bin can therefore be associated with a single DOA.In our procedure, we apply a DNN with a U-net architecture to infer the DOA of each TF bin from a concatenated set of the spectra of the microphone signals. Separation is obtained by multiplying the reference microphone by the masks associated with the different DOA. Our proposed method is inspired by the recent advances in deep clustering methods. Unlike already established methods that apply the clustering in a latent embedded space, in our approach the embedding is closely associated with the spatial information, as manifested by the different speakers' directions of arrival.
- Natural Sounds are Coded by a Hierarchy of Timescales in Human Auditory Cortex
Sam V Norman-Haignere, Laura K. Long, Orrin Devinsky, Werner Doyle, Guy M. McKhann, Catherine A. Schevon, Adeen Flinker, Nima Mesgarani (Columbia University, NYU Langone Medical Center, Columbia University Medical Center)Abstract- Natural sounds like speech and music are structured at many different timescales from milliseconds to seconds. How does the brain extract meaning from these diverse timescales? Answering this question has been challenging because there are no general methods for estimating sensory timescales in the brain, particularly in higher-order brain regions that exhibit nonlinear tuning for natural sounds. Here, we develop a simple experimental paradigm for estimating the timescale of any sensory response to any stimulus set by measuring the time window needed for the response to become invariant to surrounding context. We apply this “temporal context invariance” (TCI) paradigm to intracranial recordings from human auditory cortex, which enables us to compare the timescale of different regions of the auditory cortical hierarchy. Our results reveal a four-fold increase in timescales as one ascends the cortical hierarchy, along which there is a transition from coding lower-level acoustic features to category-specific properties. Moreover, we show that auditory cortical timescales do not vary across different stimulus timescales, demonstrating they reflect an intrinsic property of the neural response. These findings reveal a central organizing principle used by the human auditory cortex to code natural sounds.
- Multi-talker Speech Separation for Machine Listening: A Review on Recent Progress
Abrar Hussain, Wei-Ping Zhu, Kalaivani Chellappan (Concordia University, Universiti Kebangsaan Malaysia)Abstract- The booming speech recognition technology, used for machine listening of mobile phones through multiparty human-machine interaction technology, and for hearing assisted devices, have made the human’s life easier over the decades. To assist this technology, speech separation works as front-end processing, where one or multiple speech sources can be separated in a multi-talker acoustic environment. To date, numerous techniques have been developed to facilitate the speech separation process, although the progress has mostly taken place in machine learning techniques. The purpose of this review is to present the up-to-date progress and technical insights on the multitalker speech separation techniques which have been not covered in the earlier published review articles. The review discusses the supervised techniques of speech separation under the broad category of single and multi-microphone scenarios in a short yet informative way.
- Deconfounding acoustic-prosodic entrainment and self-consistency through Deep Neural Networks
Andreas Weise, Rivka Levitan (CUNY)Abstract- Human interlocutors tend to engage in adaptive behavior known as entrainment to become more similar. Isolating the effect of self-consistency, i.e., speakers adhering to their individual styles, is a critical part of the analysis of entrainment. We propose to treat speakers’ initial vocal characteristics as confounds for the prediction of subsequent outputs. Using two existing neural approaches to deconfounding, we define new measures of entrainment. These successfully discriminate real interactions from fake ones. Interestingly, our stricter methods correlate with social variables in opposite direction from previous measures that do not account for self-consistency. These results demonstrate the utility of using neural networks to model entrainment and raise questions regarding how to interpret prior associations of conversation quality with entrainment measures that do not account for self-consistency.
- A Theory on Generalization Power of DNN-based Vector-to-Vector Regression with an Illustration of Speech Enhancement
Jun Qi, Xiaoli Ma, Chin-Hui Lee (Georgia Institute of Technology)Abstract- This work concentrates on a theoretical analysis on generalization power of deep neural network (DNN) based vector-to-vector regression. Leveraging upon statistical learning theory, the generalization power can be analyzed by quantifying a generalization loss of empirical risk minimizer (ERM), which can be upper bounded by the sum of an approximation error, an estimation error, and an optimization bias. Moreover, each of the three errors can be further bounded based on statistical learning and non-convex optimization, which results in an overall upper bound for the generalization loss of ERM. Besides, we evaluate our theorems based on experiments of speech enhancement under both consistent and inconsistent environmental conditions. The related empirical results of speech enhancement verify our theorems on the generalization power of DNN based vector-to- vector mapping.
- Recent Developments in Time-Scale Modification of Audio
Timothy Roberts (Griffith University)Abstract- Time-Scale Modification is the classical problem of modifying the temporal domain of a signal without impacting the spectral domain. Traditionally, this has been accomplished through using Phase Vocoder and similarity overlap-adding methods. Since Driedger, Muller and Ewert introduced TSM using Harmonic-Percussive Separation (2013) and published "A Review of Time-Scale Modification of Music Signals" (2016) there have been a number of developments within the field. These developments include, Audio Time-Stretching Using Fuzzy Classification of Spectral Bins (Damskagg, 2017), Epoch Synchronous Overlap-Add (Rudresh, 2018), Mel-Scale Sub-Band Modelling for Perceptually Improved TSM of Speech and Audio Signals, Frequency Dependent TSM (Roberts, 2018) and Fuzzy Epoch Synchronous Overlap-Add (Roberts, 2019). This poster presentation gives a brief overview of each of these developments, and the current research of the author within this field.
- MIMII Dataset: Sound dataset for Malfunctioning Industrial Machine Investigation and Inspection
Harsh Purohit, Ryo Tanabe, Kenji Ichige, Takashi Endo, Yuki Nikaido, Kaori Suefusa, and Yohei Kawaguchi (Hitachi, Ltd.)Abstract- Factory machinery is prone to failure or breakdown, resulting in significant expenses for companies. Hence, there is a rising interest in machine monitoring using different sensors including microphones. In scientific community, the emergence of public datasets has been promoting the advancement in acoustic detection and classification of scenes and events, but there are no public datasets that focus on the sound of industrial machines under normal and anomalous operating conditions in real factory environments. In this paper, we present a new dataset of industrial machine sounds which we call a sound dataset for malfunctioning industrial machine investigation and inspection (MIMII dataset). Normal and anomalous sounds were recorded for different types of industrial machines, i.e. valves, pumps, fans and slide rails. To resemble the real-life scenario, various anomalous sounds have been recorded, for instance, contamination, leakage, rotating unbalance, rail damage, etc. The purpose of releasing the MIMII dataset is to help the machine-learning and signal-processing community to advance the development of automated facility maintenance.
- Unsupervised Anomalous Sound Detection Based on Beta Variational Autoencoder Generalized by Beta Divergence
Yohei Kawaguchi, Kaori Suefusa, Harsh Purohit, Ryo Tanabe, and Takashi Endo (Hitachi, Ltd.)Abstract- To develop a sound-monitoring system for checking machine health, a method based on the beta variational autoencoder (beta VAE) for detecting anomalous sounds is proposed. The loss function of the beta VAE consists of two terms: one is a reconstruction-error term, and the other is a disentanglement term. For the reconstruction error, the Euclidian distance is the most widely used, but there is no rationale that it is the best. In this work, we generalize the reconstruction-error to the beta divergence and also experimentally check the AUC by changing the divergence beta and the other beta for controlling disentanglement.
- Probabilistic Non-Convex Optimization for Stereo Source Separation
Gerald Schuller, Oleg Golokolenko (Ilmenau University of Technology)Abstract- We present a novel non-convex probabilistic optimization method for audio stereo source separation. Traditionally, Gradient Descent is used on a reformulated objective function to obtain coefficients for a separation function (as in subband ICA). But Gradient Descent is sensitive to getting stuck in local minima, and the reformulated objective function can be complex to compute, or introduce artifacts, for instance when subband decompositions, like the STFT, are used. Our new approach is to use a simple, non-convex objective function directly in the time domain (for instance the Kullback-Leibler Divergence) on the output of our separation or unmixing function, without reformulating it. Then we apply our new probabilistic optimization method, which has an update step that makes it suitable to real-time applications like tele-conferencing, and does not need derivatives. This leads to a simple structure. We show that it has low complexity, no unnatural sounding artifacts, and a separation performance comparable to previous approaches. Since this method does not need gradients, that also makes it advantageous for training deep neural networks. We will have audio demos (possibly live).
- Audio-Visual Speech Enhancement Using Conditional Variational Auto-Encoder
Mostafa Sadeghi, Simon Leglaive, Xavier Alameda-Pineda, Laurent Girin, Radu Horaud (INRIA Grenoble Rhone-Alpes, Gipsa-Lab, Univ. Grenoble Alpes)Abstract- Variational auto-encoders (VAEs) are deep generative latent variable models that can be used for learning the distribution of complex data. VAEs have been successfully used to learn a probabilistic prior over speech signals, which is then used to perform speech enhancement. One advantage of this generative approach is that it does not require pairs of clean and noisy speech signals at training. In this paper, we propose audio-visual variants of VAEs for single-channel and speaker-independent speech enhancement. We develop a conditional VAE (CVAE) where the audio speech generative process is conditioned on visual information of the lip region. At test time, the audio-visual speech generative model is combined with a noise model based on nonnegative matrix factorization, and speech enhancement relies on a Monte Carlo expectation-maximization algorithm. Experiments are conducted with the recently published NTCD-TIMIT dataset. The results confirm that the proposed audio-visual CVAE effectively fuses audio and visual information, and it improves the speech enhancement performance compared with the audio-only VAE model, especially when the speech signal is highly corrupted by noise. We also show that the proposed unsupervised audio-visual speech enhancement approach outperforms a state-of-the-art supervised deep learning method.
- Voice Conversion Using Phonetic Information and WaveRNN
Shahan Nercessian (iZotope)Abstract- A system for voice conversion using phonetic information and WaveRNN is presented. The system consists of three sub-networks. First, a phoneme classifier is trained, whose output effectively conveys the phonetic content of speech at each time frame. This network is used to map phonetic information to its corresponding Mel spectrogram for a target speaker. The predicted Mel spectrogram is then used as a local conditioning signal for a WaveRNN neural vocoder. Experimental results illustrate that the system can achieve convincing voice conversion of out-of-domain source speakers, and the approach can easily be extended to multiple target speakers.
- End-to-End Melody Note Transcription Based on a Beat-Synchronous Attention Mechanism
Ryo Nishikimi, Eita Nakamura, Masataka Goto, Kazuyoshi Yoshii (Kyoto University)Abstract- Our poster presents an end-to-end audio-to-symbolic singing transcription method for mixtures of vocal and accompaniment parts. Given audio signals with non-aligned melody scores, we aim to train a recurrent neural network that takes as input a magnitude spectrogram and outputs a sequence of melody notes (pitches and note values) and metrical structures (beats and downbeats). A promising approach to such sequence-to-sequence learning (joint input-to-output alignment and mapping) is to use an encoder-decoder model with an attention mechanism. This approach, however, cannot be used straightforwardly for singing transcription because a note-level decoder fails to estimate note values from latent representations obtained by a frame-level encoder that is good at extracting instantaneous features, but poor at extracting temporal features. To solve this problem, we focus on tatums instead of notes as output units and propose a tatum-level decoder that sequentially outputs tatum-level score segments represented by note pitches, note onset frags, and beat and downbeat flags. We then propose a beat-synchronous attention mechanism constrained in order to monotonically align tatum-level scores with input audio signals with a steady increment.
- Cutting Music Source Separation Some Slakh: a Dataset to Study the Impact of Training Data Quality and Quantity
Ethan Manilow, Gordon Wichern, Prem Seetharaman, Jonathan Le Roux (MERL, Northwestern University)Abstract- Music source separation performance has greatly improved in recent years with the advent of approaches based on deep learning. Such methods typically require large amounts of labelled training data, which in the case of music consist of mixtures and corresponding instrument stems. However, stems are unavailable for most commercial music, and only limited datasets have so far been released to the public. It can thus be difficult to draw conclusions when comparing various source separation methods, as the difference in performance may stem as much from better data augmentation techniques or training tricks to alleviate the limited availability of training data, as from intrinsically better model architectures and objective functions. In this paper, we present the synthesized Lakh dataset (Slakh) as a new tool for music source separation research. Slakh consists of high-quality renderings of instrumental mixtures and corresponding stems generated from the Lakh MIDI dataset (LMD) using professional-grade sample-based virtual instruments. A first version, Slakh2100, focuses on 2100 songs, resulting in 145 hours of mixtures. While not fully comparable because it is purely instrumental, this dataset contains an order of magnitude more data than MUSDB18, the de facto standard dataset in the field. We show that Slakh can be used to effectively augment existing datasets for musical instrument separation, while opening the door to a wide array of data-intensive music signal analysis tasks.
- Using TTS To Learn SLU
Loren Lugosch, Brett Meyer, Derek Nowrouzezahrai, Mirco Ravanelli (Mila / McGill University)Abstract- End-to-end models are an attractive new approach to spoken language understanding (SLU) in which the meaning of an utterance is inferred directly from the raw audio without employing the standard pipeline composed of a separately trained speech recognizer and natural language understanding module. The downside of end-to-end SLU is that in-domain speech data must be recorded to train the model. In this work, we propose a strategy for overcoming this requirement in which speech synthesis is used to generate a large synthetic training dataset from several artificial speakers. Experiments on two open-source SLU datasets confirm the effectiveness of our approach, both as a sole source of training data and as a form of data augmentation.
- Metamers of audio-trained deep neural networks
Jenelle Feather, Alex Durango, Ray Gonzalez, Josh McDermott (MIT)Abstract- Deep neural networks have been embraced as models of sensory systems, instantiating representational transformations that appear to resemble those in both the visual and auditory systems. To more thoroughly investigate the similarity of artificial neural networks to biological systems, we synthesized model metamers – stimuli that produce the same responses at some stage of a network’s representation – and asked whether they also produce similar responses in the human auditory system. We generated model metamers for natural speech by performing gradient descent on a noise signal, modifying the noise signal so as to match the responses of individual neural network layers to the responses elicited by a speech signal. We then measured whether model metamers were recognizable to human observers – a necessary condition for the model representations to replicate those of humans. Although model metamers from early network layers were recognizable to humans, those from deeper layers generally were not, indicating that the invariances instantiated in the network diverged from those of human perception. However, the model metamers became more recognizable after architectural modifications that might be expected to yield better models of a sensory system (by reducing aliasing artifacts from downsampling operations). Moreover, metamers were more recognizable for networks trained to recognize speech than those trained to classify auditory scenes, suggesting that model representations can be pushed closer to human perception with appropriate training tasks. Our results reveal discrepancies between model and human representations, but also show how metamers can elucidate model representations and guide model refinement.
- Sound Event Detection using Point-labeled Data
Bongjun Kim, Bryan Pardo (Northwestern University)Abstract- Building Sound Event Detection (SED) systems typically require a large amount of carefully annotated, strongly labeled data, where the exact time-span of a sound event (e.g. the dog bark starts at 1.2 seconds and ends at 2.0 seconds) in an audio scene (a recording of a city park) is indicated. However, manual labeling of sound events with their time boundaries within a recording is very time-consuming. One way to solve the issue is to collect data with weak labels that only contain the names of sound classes present in the audio file, without time boundary information for events in the file. Therefore, weakly-labeled sound event detection has become popular recently. However, there is still a large performance gap between models built on weakly labeled data and ones built on strongly labeled data, especially for predicting time boundaries of sound events. In this work, we introduce a new type of sound event label, which is easier for people to provide than strong labels. We call them point labels. To create a point label, a user simply listens to the recording and hits the space bar if they hear a sound event (dog bark). This is much easier to do than specifying exact time boundaries. In this work, we illustrate methods to train a SED model on point-labeled data. Our results show that a model trained on point labeled audio data significantly outperforms weak models and is comparable to a model trained on strongly labeled data.
- Spherical Microphone Array Shape to improve Beamforming Performance
Sakurako Yazawa, Hiroaki Itou, Kenichi Noguchi, Kazunori Kobayashi, and Noboru Harada (NTT)Abstract- A 360-degree steerable super-directional beamforming are proposed. We designed a new acoustic baffle for spherical microphone array to achieve both small size and high performance. The shape of baffle is sphere with parabola-like depressions; therefore, sound-collection performance can be enhanced using reflection and diffraction. We first evaluated its beamforming performance through simulation then fabricated a 3D prototype of an acoustic baffle microphone array with the proposed baffle shape and compared its performance to that of a conventional spherical 3D acoustic baffle. This prototype exhibited better beamforming performance. We built microphone array system that includes the proposed acoustic baffle and a 360-degree camera, our system can pick up matched sound to an image in a specific direction in real-time or after recording. We have received high marks from users who experienced the system demo.
- ToyADMOS: A Dataset of Miniature-Machine Operating Sounds for Anomalous Sound Detection
Yuma Koizumi, Shoichiro Saito, Hisashi Uematsu, Noboru Harada, Keisuke Imoto (NTT Media Intelligence Laboratories)Abstract- This paper introduces a freely available new dataset called "ToyADMOS" designed for anomaly detection in machine operating sounds (ADMOS). To build a large-scale dataset for ADMOS, we collected anomalous operating sounds of miniature machines (toys) by deliberately damaging them. The released dataset consists of three sub-datasets for machine-condition inspection, fault diagnosis of machines with geometrically fixed tasks, and fault diagnosis of machines with moving tasks. Each sub-dataset includes over 180 hours of normal machine-operating sounds and over 4,000 samples of anomalous sounds collected with four microphones at a 48-kHz sampling rate.
- DOA Estimation by DNN-based Denoising and Dereverberation from Sound Intensity Vector
Masahiro Yasuda (NTT Media Intelligence Laboratories)Abstract- We propose a direction of arrival (DOA) estimation method that combines a sound intensity vector (IV)-based DOA-estimation and DNN-based denoising and dereverberation. Since the accuracy of IV-based DOA-estimation is degraded by environmental noise and reverberation, two DNNs are used to remove such the effects from the observed IVs. Then DOA is estimated from calculated from the refined IVs based on the physics of wave propagation. Experiments on an open dataset show that the average DOA error of the proposed method was less than 0.7 degrees, and outperformed a conventional IV-based and DNN-based DOA estimation methods.
- Learning the helix topology of musical pitch
Vincent Lostanlen, Sripathi Sridhar, Brian McFee, Juan Bello (NYU)Abstract- Spectrograms being a useful visualization of musical information, monotonically organize frequencies along the vertical axis and time along the horizontal axis. This is appropriate to understand musical data in the context of how humans perceive pure tones. However, such a rectilinear representation does not match our perception of real-world sounds, which typically exhibit a Fourier series of overtones. The goal of this project is to explore alternate representations to visualize the statistical correlations across subbands in time-frequency representations of pitched sounds. To this end, we employ manifold learning on the Pearson correlations of datasets in an unsupervised manner. The Isomap algorithm is used to achieve a three-dimensional embedding of music (Studio On Line Dataset) and speech (North Texas Vowel Database) data. Our results indicate the identification of a helical topology from these long-range correlations. This is in tune with prior knowledge from music theory and music cognition, that musical notes lie on a helix characterized by angular and vertical organization of pitch class and pitch height respectively. However, the proposed framework could be used in deep learning architectures to inform weight sharing strategies through empirically derived local correlations in the data.
- Multi-Task Learning for Multiple Audio Source Separation
Stephen Carrow, Brian McFee (NYU)Abstract- Deep neural networks have achieved good results on musical source separation. However, the techniques explored previously train one model per source requiring either sequential training and inference or additional computational resources for parallelization. We hypothesize that some internal representations learned by these neural networks are in fact common across sources. We therefore develop a multi-task deep neural network that simultaneously separates multiple sources, which performs reasonably well compared to top performing single-task networks on MUSDB18 while reducing the parameter count by 52%.
- An extensible cluster-graph taxonomy for open set sound scene analysis
Helen L. Bear and Emmanouil Benetos (Queen Mary University of London)Abstract- We present a new extensible and divisible taxonomy for open set sound scene analysis. This new model allows complex scene analysis with tangible descriptors and perception labels. Its novel structure is a cluster graph such that each cluster (or subset) can stand alone for targeted analyses such as office sound event detection, whilst maintaining integrity over the whole graph (superset) of labels. The key design benefit is its extensibility as new labels are needed during new data capture. Furthermore, datasets which use the same taxonomy are easily augmented, saving future data collection effort. We balance the details needed for complex scene analysis with avoiding `the taxonomy of everything' with our framework to ensure no duplicity in the superset of labels and demonstrate this with DCASE challenge classifications.
- Adversarial Attacks in Audio Applications
Vinod Subramanian, Emmanouil Benetos, Mark Sandler (Queen Mary University of London)Abstract- Adversarial attacks refer to a set of methods that perturb the input to a classification model in order to fool the classifier. The perturbed inputs are called adversarial examples. Adversarial examples pose a security threat because security systems that use audio classification to identify gunshots, glass breaking etc. can be evaded. Adversarial examples also provide a tool to identify the robustness of features learned by a deep learning model. In this poster we will give a brief overview of adversarial attacks for sound event classification and singing voice detection. We will have a laptop with headphones so that people can listen to what an adversarial examples sounds like. Through the listening examples and the data we will demonstrate that adversarial attacks exist irrespective of model architecture and input transformation. For our future work we mention our approach to use adversarial attacks to identify the non-robust and robust features in a deep learning model.
- Neural Machine Transcription for Sound Events
Arjun Pankajakshan, Helen L. Bear, Emmanouil Benetos (Queen Mary University of London)Abstract- Sound event detection (SED) refers to the detection of sound event types and event boundaries in audio signals. Many recent works based on deep neural models adopt a frame-level multi-label classification approach to perform polyphonic SED. In a different approach, SED has been formulated as a multi-variable regression problem based on the frame position information associated with the sound events. In either of the approaches, SED systems are trained frame-wise; consequently, these systems fail to model individual sound event instances. We propose to formulate polyphonic SED as an event-instance based sequence learning problem similar to machine translation in natural language processing (NLP). Two main advantages are expected from our approach – 1) Event-instance based training yields representations for individual sound event instances in an audio signal similar to the word vectors for a sentence. 2) This model design is suitable for investigating the relevance of the temporal sequence information of the sound events in SED systems similar to the necessity of word sequence structure used to form a meaningful sentence in NLP.
- Overview of tasks and investigation of subjective evaluation methods in environmental sound synthesis and conversion
Yuki Okamoto, Keisuke Imoto, Tatsuya Komatsu, Shinnosuke Takamichi, Takumi Yagyu, Ryosuke Yamanishi, Yoichi Yamashita (Ritsumeikan University, LINE Corporation, The University of Tokyo)Abstract- Synthesizing and converting environmental sounds have the po- tential for many applications such as supporting movie and game production, data augmentation for sound event detection and scene classification. Conventional works on synthesizing and converting environmental sounds are based on a physical modeling or concate- native approach. However, there are a limited number of works that have addressed environmental sound synthesis and conversion with statistical generative models; thus, this research area is not yet well organized. In this paper, we overview problem definitions, appli- cations, and evaluation methods of environmental sound synthesis and conversion. We then report on environmental sound synthesis using sound event labels, in which we focus on the current perfor- mance of statistical environmental sound synthesis and investigate how we should conduct subjective experiments on environmental sound synthesis.
- Deep Unsupervised Drum Transcription
Keunwoo Choi, Kyunghyun Cho (Spotify, NYU)Abstract- We introduce DrummerNet, a drum transcription system that is trained in an unsupervised manner. DrummerNet does not require any ground-truth transcription and, with the data-scalability of deep neural networks, learns from a large unlabeled dataset. In DrummerNet, the target drum signal is first passed to a (trainable) transcriber, then reconstructed in a (fixed) synthesizer according to the transcription estimate. By training the system to minimize the distance between the input and the output audio signals, the transcriber learns to transcribe without ground truth transcription. Our experiment shows that DrummerNet performs favorably compared to many other recent drum transcription systems, both supervised and unsupervised.
- Zero-shot Audio Classification Based on Class Label Embeddings
Huang Xie, Tuomas Virtanen (Tampere University)Abstract- This paper proposes a zero-shot learning approach for audio classification based on the textual information about class labels without any audio samples from target classes. We propose an audio classification system built on the bilinear model, which takes audio feature embeddings and semantic class label embeddings as input, and measures the compatibility between an audio feature embedding and a class label embedding. We use VGGish to extract audio feature embeddings from audio recordings. We treat textual labels as semantic side information of audio classes, and use Word2Vec to generate class label embeddings. Results on the ESC-50 dataset show that the proposed system can perform zero-shot audio classification with small training dataset. It can achieve accuracy (26 % on average) better than random guess (10 %) on each audio category. Particularly, it reaches up to 39.7 % for the category of natural audio classes.
- Components Loss for Neural Networks in Mask-Based Speech Enhancement
Ziyi Xu, Samy Elshamy, Ziyue Zhao, Tim Fingscheidt (Technische Universität Braunschweig)Abstract- Estimating time-frequency domain masks for single-channel speech enhancement using deep learning methods has recently become a popular research field with promising results. In this paper, we propose a novel components loss (CL) for the training of neural networks for mask-based speech enhancement. During the training process, the proposed CL offers separate control over preservation of the speech component quality, suppression of the residual noise component, and preservation of a naturally sounding residual noise component. We illustrate the potential of the proposed CL by evaluating a standard convolutional neural network (CNN) for mask-based speech enhancement. The new CL obtains a better and more balanced performance in almost all employed instrumental quality metrics over the baseline losses, the latter comprising the conventional mean squared error (MSE) loss and also auditory-related loss functions, such as the perceptual evaluation of speech quality (PESQ) loss and the recently proposed perceptual weighting filter loss. Particularly, applying the CL offers better speech component quality, better overall enhanced speech perceptual quality, as well as a more naturally sounding residual noise. On average, an at least 0.1 points higher PESQ score on the enhanced speech is obtained while also obtaining a higher SNR improvement by more than 0.5 dB, for seen noise types. This improvement is stronger for unseen noise types, where an about 0.2 points higher PESQ score on the enhanced speech is obtained, while also the output SNR is ahead by more than 0.5 dB. The new proposed CL is easy to implement and code is provided at this https URL.
- ConvLSTM Layers Preserve Structural Information for Speech Enhancement: A Visualization Example
Maximilian Strake, Bruno Defraene, Kristoff Fluyt, Wouter Tirry, Tim Fingscheidt (Technische Universität Braunschweig)Abstract- In single-channel speech enhancement based on supervised learning, convolutional neural networks (CNNs) integrating several long short-term memory (LSTM) layers for temporal modeling and performing a direct mapping from noisy to clean speech spectra have recently become very popular. However, it can be difficult for standard fully-connected LSTM layers to preserve structural information passed on from previous CNN layers, where internal feature representations are organized in feature maps. Using visualizations of the feature representations in such a network, we show that information about the harmonic structure of speech existing in the feature representations of the CNN layers can be preserved much better when employing convolutional LSTMs (ConvLSTMs) for temporal modeling instead of fully-connected LSTMs, which in turn leads to improved speech enhancement performance.
- Perceptual soundfield reproduction over multiple sweet spots using a practical loudspeaker array
Huanyu Zuo, Prasanga N. Samarasinghe, and Thushara D. Abhayapala (The Australian National University (ANU))Abstract- Perceptual soundfield reproduction aims to provide impressive sound localization rather than reproduce an accurate physical approximation of a soundfield. Sound intensity has been controlled in reproduction systems to improve the performance of perceptual localization since sound intensity theories were developed for psychoacoustically optimum sound. However, most of the previous works in this field are either restricted to a single reproduction position, or constrained to an impractical loudspeaker array. In this work, we consider a practical reproduction system that is modelled to reconstruct the desired sound intensity over multiple sweet spots by driving an irregular loudspeaker array. We represent sound intensity in spherical harmonic domain and optimize it at all of the sweet spots by a cost function. The performance of the proposed method is evaluated by comparing it with the state of the art method through numerical simulations. Finally, Perceptual listening tests are conducted to verify the simulation results.
- Kernel Ridge Regression with Constraint of Helmholtz Equation for Sound Field Interpolation
Natsuki Ueno, Shoichi Koyama, and Hiroshi Saruwatari (The University of Tokyo)Abstract- Kernel ridge regression with the constraint of the Helmholtz equation for three-dimensional sound field interpolation is proposed. We show that sound field interpolation problems can be formulated as the kernel ridge regression with a reproducing kernel given by the zeroth-order spherical Bessel function of the first kind. We also evaluate the proposed method by numerical experiments.
- Using Blinky Sound-to-Light Conversion Sensors for Various Audio Processing Tasks
Robin Scheibler, Daiki Horiike, Nobutaka Ono (Tokyo Metropolitan University)Abstract- We designed the Blinky, a novel kind of sensor that converts sound power to light intensity. Light and battery-powered, it can be deployed in large numbers and the sound power over a large area harvested with a conventional camera. We will showcase in this presentation applications of this system to classic audio tasks such as source localization, separation, and beamforming.
- On the contributions of visual and textual supervision in low-resource semantic speech retrieval
Ankita Prasad, Bowen Shi, Herman Kamper, Karen Livescu (TTI-Chicago)Abstract- Recent work has shown that speech paired with images can be used to learn semantically meaningful speech representations even without any textual supervision. In real-world low-resource settings, however, we often have access to some transcribed speech. We study whether and how visual grounding is useful in the presence of varying amounts of textual supervision. In particular, we consider the task of semantic speech retrieval in a low-resource setting. We propose a multitask learning approach to leverage both visual and textual modalities. We find that visual grounding is helpful even in the presence of textual supervision, and we analyze this effect over a range of sizes of the transcribed data sets. Further, we analyze the cross-domain performance of our visually grounded model on a spoken corpus not describing visual scenes.
- Weakly Informed Audio Source Separation
Kilian Schulze-Forster, Clement Doire, Gaël Richard, Roland Badeau (Télécom Paris, Audionamix)Abstract- Prior information about the target source can improve audio source separation quality but is usually not available with the nec- essary level of audio alignment. This has limited its usability in the past. We propose a separation model that can nevertheless ex- ploit such weak information for the separation task while aligning it on the mixture as a byproduct using an attention mechanism. We demonstrate the capabilities of the model on a singing voice separa- tion task exploiting artificial side information with different levels of expressiveness. Moreover, we highlight an issue with the com- mon separation quality assessment procedure regarding parts where targets or predictions are silent and refine a previous contribution for a more complete evaluation.
- Wearable Microphone Arrays
Ryan M. Corey, Andrew C. Singer (University of Illinois at Urbana-Champaign)Abstract- Microphone array processing can help augmented listening devices, such as hearing aids, smart headphones, and augmented reality headsets, to better analyze and enhance the sounds around them. This poster summarizes challenges and recent advances in the design of wearable microphone arrays. It reviews our group’s recent results on the acoustic effects of the body, performance scaling in large wearable arrays, and the effects of body movement on array performance. It also presents a recently released open-access database of over 8000 acoustic impulse responses for wearable microphones.
- Acoustically Inspired Algorithms for Modeling and Audio Enhancement via Orthonormal Basis Functions
Sahar Hashemgeloogerdi (University of Rochester)Abstract- Interactive acoustic systems such as spatial audio rendering, 3D sound localization, and feedback cancellation systems rely on real-time audio signal processing. The ability of systems to adapt quickly and provide lifelike acoustic experiences depends on computational efficiency and accuracy of the audio signal processing algorithms. Hence, accurate modeling of acoustic environments, e.g., room acoustics and head related transfer functions (HRTFs), utilizing as few parameters as possible is essential. We first present an accurate yet computationally efficient modeling method for highly reverberant acoustic systems. The method relies on time-frequency representation of an acoustic system and orthonormal basis functions (OBFs), enabling accurate modeling in real-time over a wide range of frequencies. To realize subband decomposition, we introduce the utilization of dual-tree complex wavelet transform, providing aliasing-free subbands. The proposed method is also less sensitive to variations of the source and microphone locations since it incorporates common acoustical poles of the system. We further introduce an adaptive feedback cancellation (AFC) algorithm derived based on the OBFs for closed-loop identification of the feedback path by minimizing the prediction error. The proposed algorithm is extensively evaluated with speech and music source signals and with sudden changes in the feedback path. The experimental results show that the proposed method significantly increases the added stable gain, accelerates the convergence rate, and enhances the sound quality compared to the conventional AFC algorithms, while requiring far fewer adaptive parameters which leads to reduced computational complexity.
- Learning with Out-of-Distribution Data for Audio Classification
Turab Iqbal, Qiuqiang Kong, Yin Cao, Mark D. Plumbley, Wenwu Wang (University of Surrey)Abstract- In supervised machine learning, the standard assumptions of data and label integrity are not always satisfied due to cost constraints or otherwise. In this submission, we investigate a particular case of this for audio classification in which the dataset is corrupted with out-of-distribution (OOD) instances: data that does not belong to any of the target classes. We show that detecting and relabelling some of these instances, rather than discarding them, can have a positive effect on learning, and propose an effective method for doing so. Experiments are carried out on the FSDnoisy18k dataset, where OOD instances are very prevalent. Using a convolutional neural network as a baseline, the proposed method is shown to improve classification by a significant margin.
- Cross-task learning for audio tagging, sound event detection and spatial localization: DCASE 2019 baseline systems
Qiuqiang Kong, Yin Cao, Turab Iqbal, Yong Xu, Wenwu Wang, Mark D. Plumbley (University of Surrey)Abstract- The DCASE 2019 challenge introduces five tasks focusing on audio tagging, sound event detection and spatial localisation. Despite the different problems and conditions present in these tasks, it is of interest to be able to use approaches that are task-independent. This submission presents a number of cross-task baseline systems based on convolutional neural networks (CNNs). These systems allow us to investigate the performance of a variety of models across several audio recognition tasks without exploiting the specific characteristics of the tasks. The presentation looks at CNNs with 5, 9 and 13 layers. Experiments show that good performance is achievable despite the lack of specialised techniques, demonstrating that generic CNNs are powerful models.